Searching for Embeddings in a Haystack: Link Prediction on Knowledge Graphs with Subgraph Pruning
WWW '20: The Web Conference 2020 Taipei Taiwan April, 2020(2020)
摘要
Embedding-based models of Knowledge Graphs (KGs) can be used to predict the existence of missing links by ranking the entities according to some likelihood scores. An exhaustive computation of all likelihood scores is very expensive if the KG is large. To counter this problem, we propose a technique to reduce the search space by identifying smaller subsets of promising entities. Our technique first creates embeddings of subgraphs using the embeddings from the model. Then, it ranks the subgraphs with some proposed ranking functions and considers only the entities in the top k subgraphs. Our experiments show that our technique is able to reduce the search space significantly while maintaining a good recall.
更多查看译文
AI 理解论文
溯源树
样例
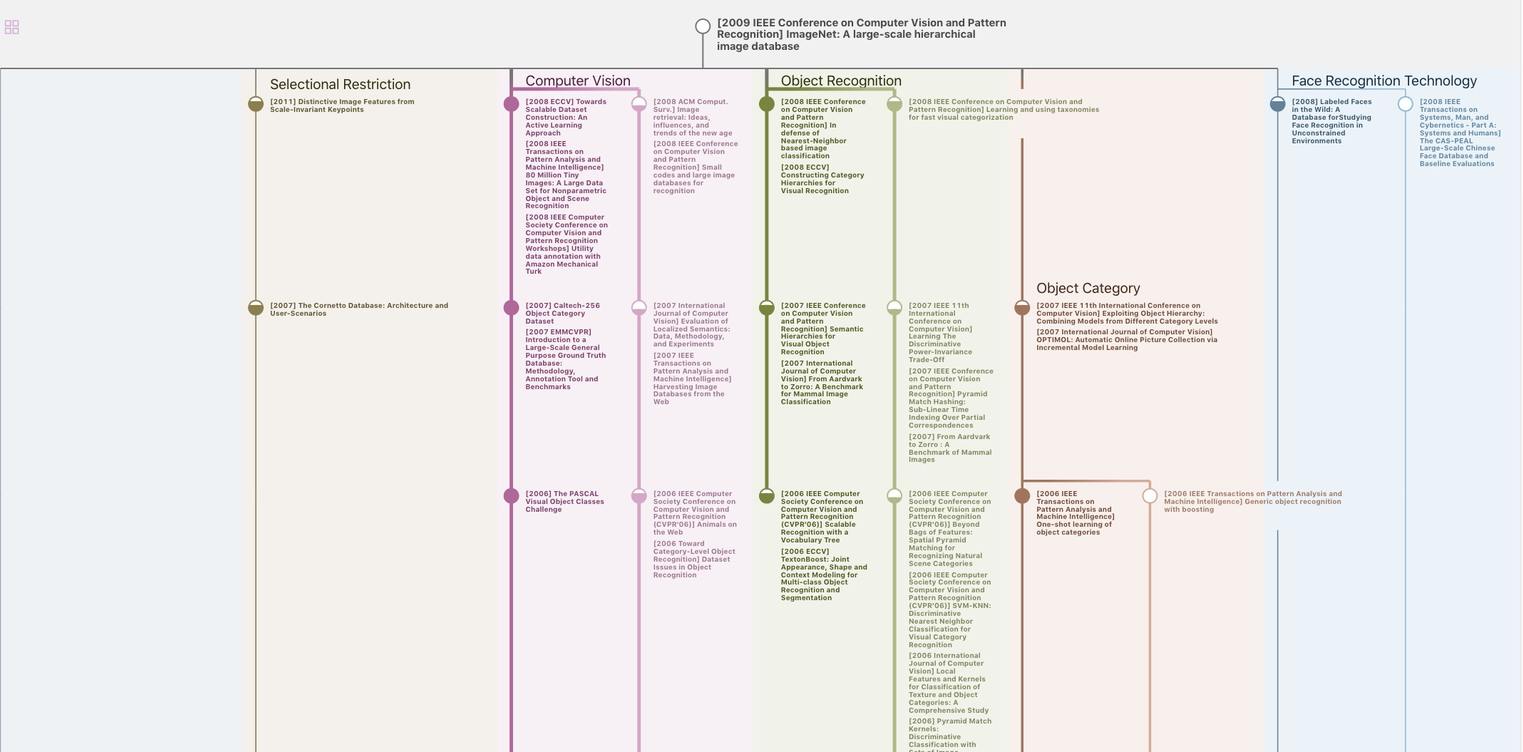
生成溯源树,研究论文发展脉络
Chat Paper
正在生成论文摘要