Adaptive Hierarchical Translation-based Sequential Recommendation
WWW '20: The Web Conference 2020 Taipei Taiwan April, 2020(2020)
摘要
We propose an adaptive hierarchical translation-based sequential recommendation called HierTrans that first extends traditional item-level relations to the category-level, to help capture dynamic sequence patterns that can generalize across users and time. Then unlike item-level based methods, we build a novel hierarchical temporal graph that contains item multi-relations at the category-level and user dynamic sequences at the item-level. Based on the graph, HierTrans adaptively aggregates the high-order multi-relations among items and dynamic user preferences to capture the dynamic joint influence for next-item recommendation. Specifically, the user translation vector in HierTrans can adaptively change based on both a user’s previous interacted items and the item relations inside the user’s sequences, as well as the user’s personal dynamic preference. Experiments on public datasets demonstrate the proposed model HierTrans consistently outperforms state-of-the-art sequential recommendation methods.
更多查看译文
AI 理解论文
溯源树
样例
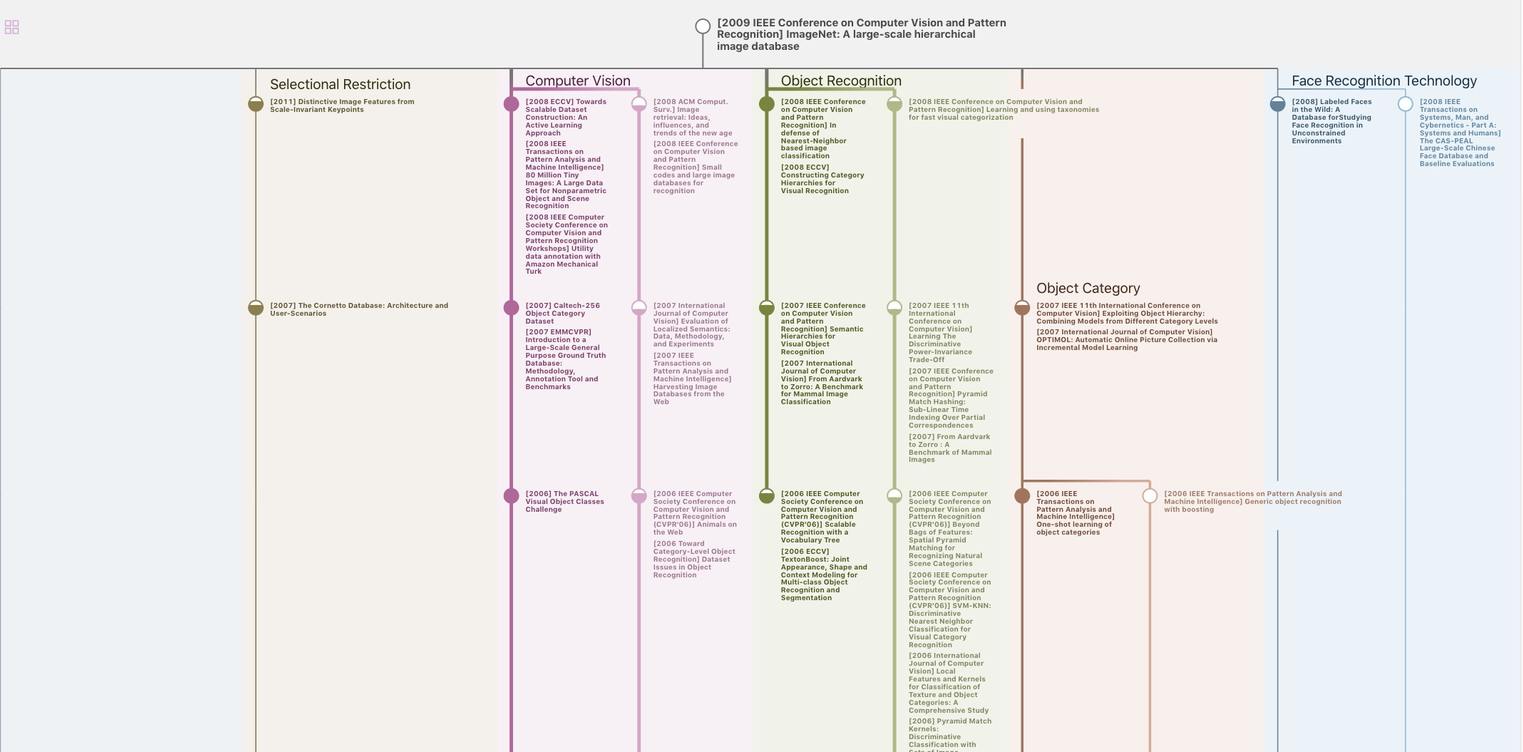
生成溯源树,研究论文发展脉络
Chat Paper
正在生成论文摘要