Seeding Network Influence in Biased Networks and the Benefits of Diversity
WWW '20: The Web Conference 2020 Taipei Taiwan April, 2020(2020)
摘要
The problem of social influence maximization is widely applicable in designing viral campaigns, news dissemination, or medical aid. State-of-the-art algorithms often select "early adopters" that are most central in a network unfortunately mirroring or exacerbating historical biases and leaving under-represented communities out of the loop. Through a theoretical model of biased networks, we characterize the intricate relationship between diversity and efficiency, which sometimes may be at odds but may also reinforce each other. Most importantly, we find a mathematically proven analytical condition under which more equitable choices of early adopters lead simultaneously to fairer outcomes and larger outreach. Analysis of data on the DBLP network confirms that our condition is often met in real networks. We design and test a set of algorithms leveraging the network structure to optimize the diffusion of a message while avoiding to create disparate impact among participants based on their demographics, such as gender or race.
更多查看译文
关键词
social networks,influence,fairness,graph algorithms
AI 理解论文
溯源树
样例
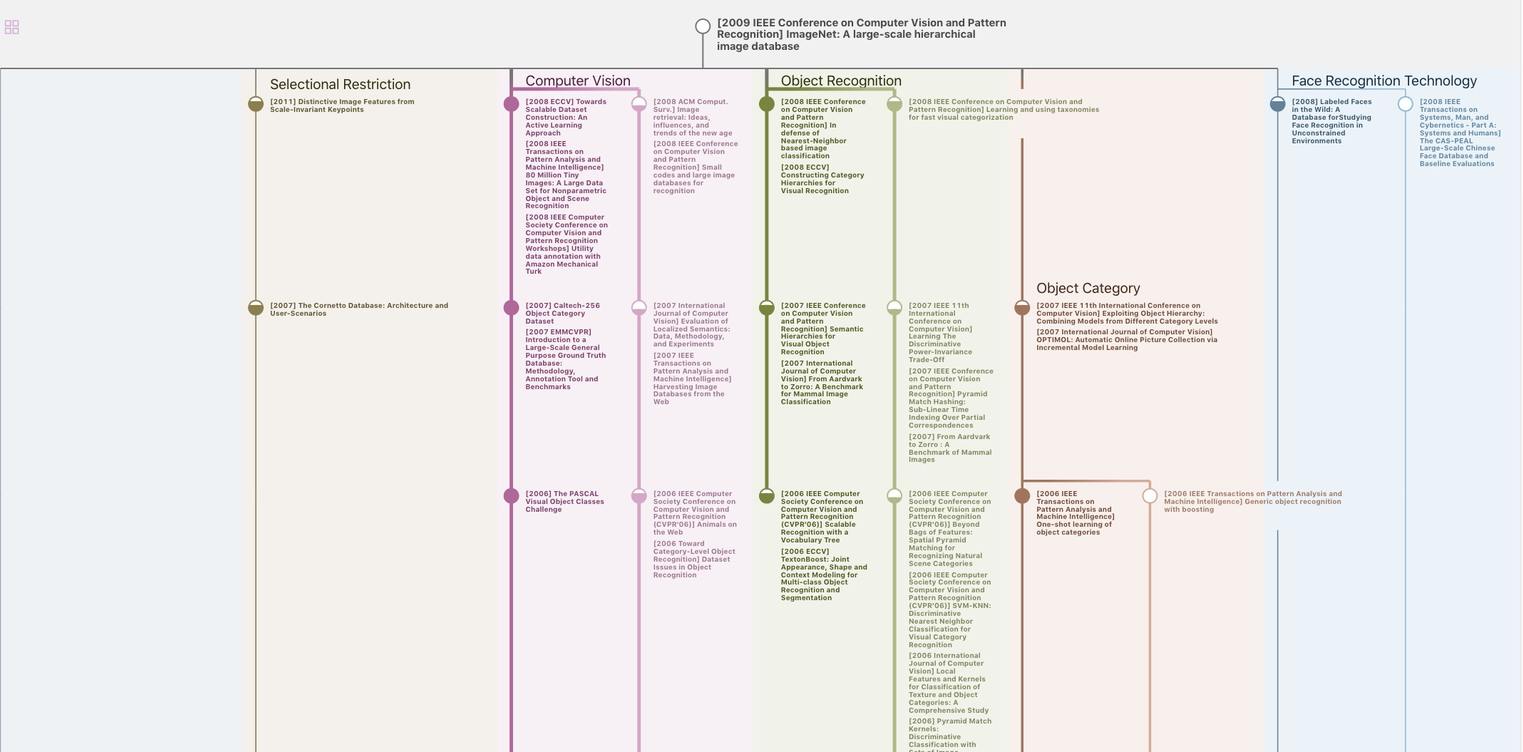
生成溯源树,研究论文发展脉络
Chat Paper
正在生成论文摘要