Few-Shot Learning for New User Recommendation in Location-based Social Networks
WWW '20: The Web Conference 2020 Taipei Taiwan April, 2020(2020)
摘要
The proliferation of GPS-enabled devices establishes the prosperity of location-based social networks, which results in a tremendous amount of user check-ins. These check-ins bring in preeminent opportunities to understand users’ preferences and facilitate matching between users and businesses. However, the user check-ins are extremely sparse due to the huge user and business bases, which makes matching a daunting task. In this work, we investigate the recommendation problem in the context of identifying potential new customers for businesses in LBSNs. In particular, we focus on investigating the geographical influence, composed of geographical convenience and geographical dependency. In addition, we leverage metric-learning-based few-shot learning to fully utilize the user check-ins and facilitate the matching between users and businesses. To evaluate our proposed method, we conduct a series of experiments to extensively compare with 13 baselines using two real-world datasets. The results demonstrate that the proposed method outperforms all these baselines by a significant margin.
更多查看译文
关键词
Customer recommendation, self-attention, few-shot learning
AI 理解论文
溯源树
样例
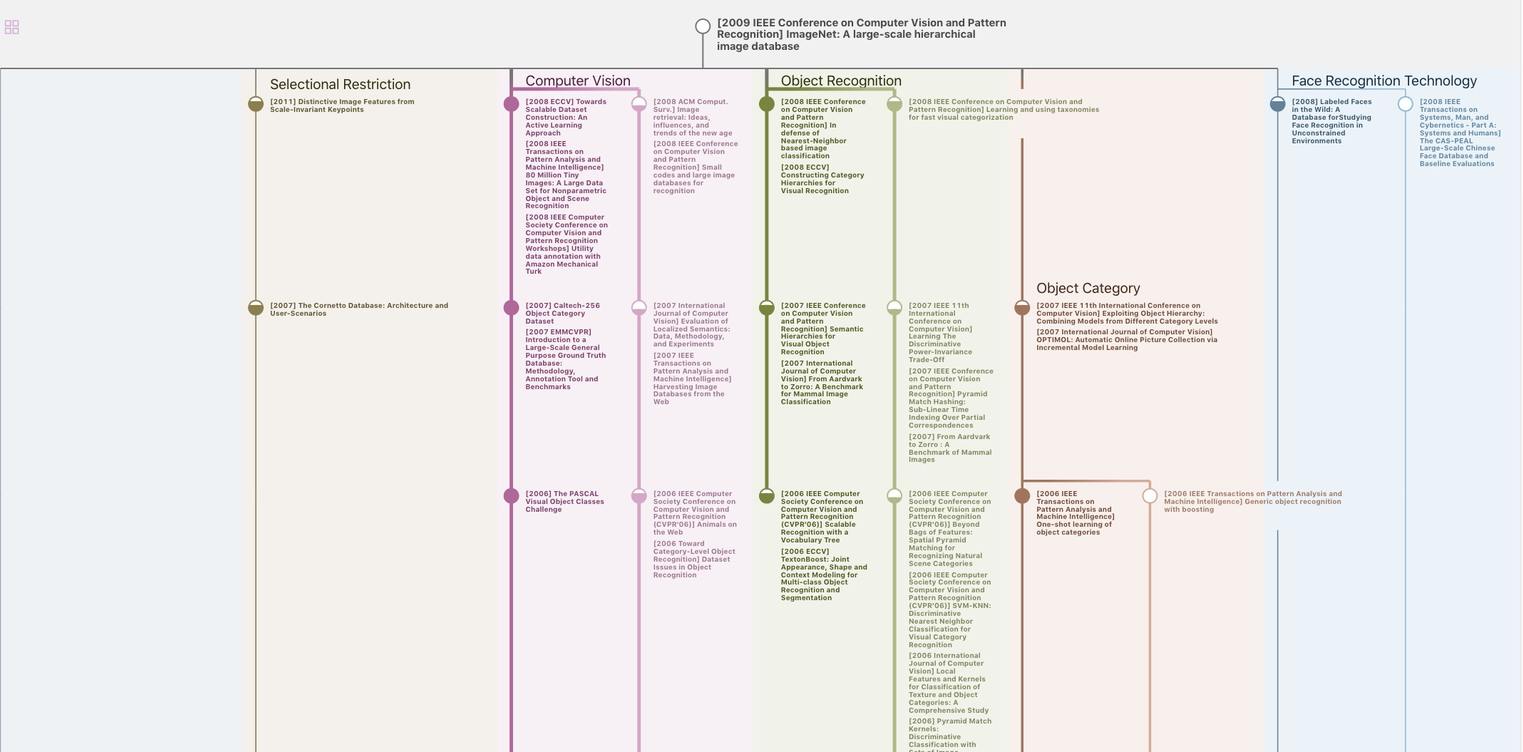
生成溯源树,研究论文发展脉络
Chat Paper
正在生成论文摘要