Parameter Estimation of Generalized Gamma Distribution Toward SAR Image Processing
IEEE Transactions on Aerospace and Electronic Systems(2020)
摘要
Statistical modeling of synthetic aperture radar (SAR) data is a crucial step in SAR image processing. In this context, the generalized gamma (GGamma) distribution, which generalizes many common distributions, has been applied to model SAR image statistics. Parameter estimation remains, however, a challenging step that conditions the quality of model fitting to data and, thus, of the required processing. In this article, we propose a novel parameter estimation method for GGamma distribution in the log-domain, named as the maximum likelihood and logarithmic cumulants (ML–LC) method. The ML–LC method constructs a novel scale-independent shape parameter estimator in the log-domain based on the Mellin transform and maximum-likelihood estimation and estimates the distribution parameters based on the multistart local search, gradient descent, and bisection methods, rather than solving the system of highly nonlinear equations in the traditional estimations. The ML–LC method is able to estimate the GGamma distribution parameters more accurately. To assess the performance of our estimation method, we perform the goodness-of-fit test on simulated data and real SAR images. In addition, we apply the ML–LC method in some SAR image processing tasks covering image segmentation, classification, and change detection. The results obtained confirm the interest of the proposed ML–LC method.
更多查看译文
关键词
Synthetic aperture radar,Maximum likelihood estimation,Parameter estimation,Shape,Data models,Transforms
AI 理解论文
溯源树
样例
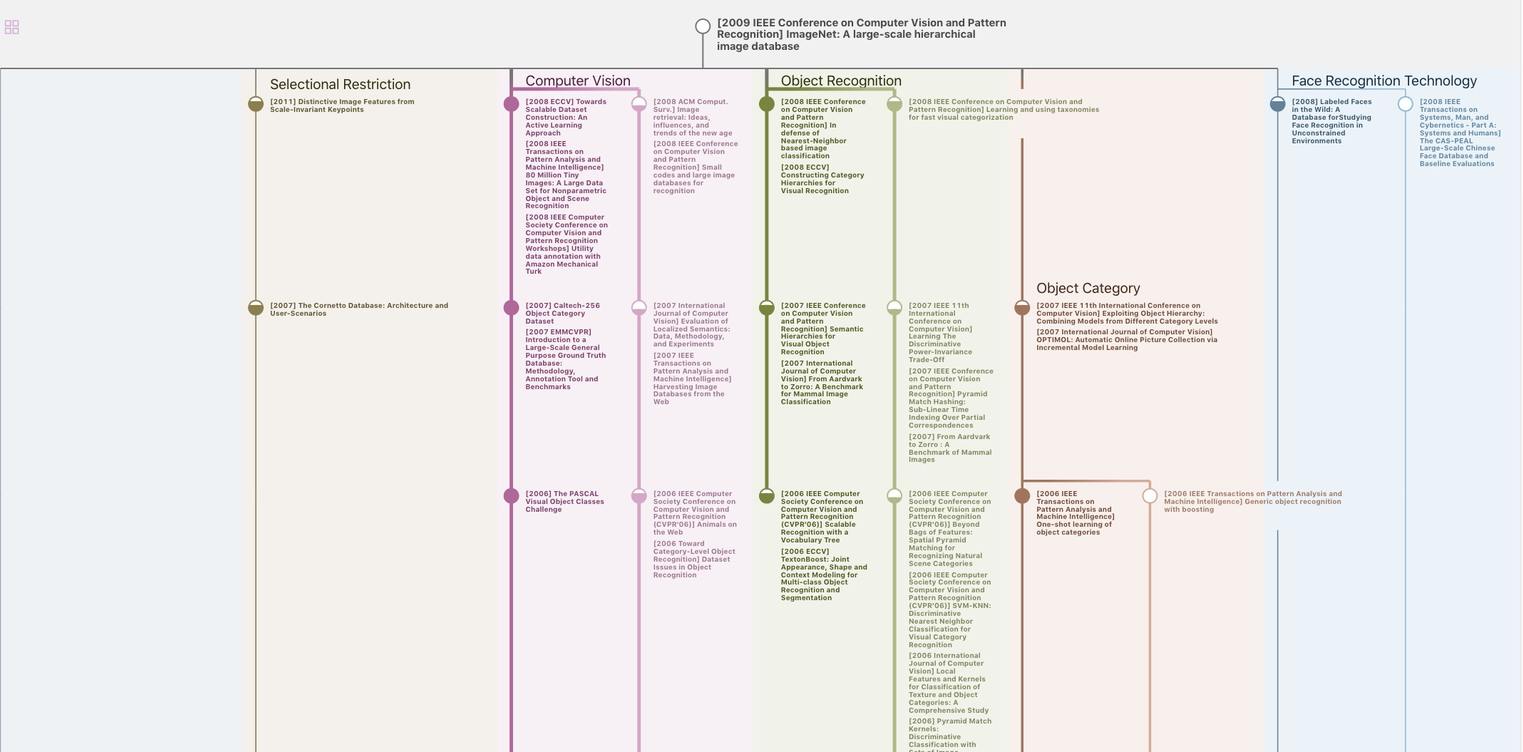
生成溯源树,研究论文发展脉络
Chat Paper
正在生成论文摘要