Noise-robust image fusion with low-rank sparse decomposition guided by external patch prior
Information Sciences(2020)
摘要
It is challenging to simultaneously achieve noise suppression and fine detail preservation in noisy image fusion. To address this challenge, we propose a novel strategy for noisy image fusion. Assuming that an image can be modeled as a superposition of low-rank and sparse (LR-and-S) components, we develop a novel discriminative dictionary learning algorithm to construct two dictionaries so as to decompose the input image into LR-and-S components. Specifically, to make dictionary possess discriminative power, we enforce spatial morphology constraint on each dictionary. Furthermore, we develop within-class consistency constraint by exploiting the similarity of low-rank components and impose it on the coding coefficients to further improve the discriminative power of the learned dictionary. In image decomposition, external patch prior and internal self-similarity prior of an image are exploited to build image decomposition model, based on which the latent subspace for fusion and recovery is estimated by minimizing rank-regularization of the subspace learned via clustering of similar patches. To construct different components of fused result, we use l1-norm maximization rule to fuse the decomposed components. Finally, the fused image is obtained by adding the fused components together. Experiments demonstrate that our method outperforms several state-of-the-art methods in terms of both objective quality assessment and subjective visual perception.
更多查看译文
关键词
Image fusion,Dictionary learning,Low-rank decomposition,Sparse representation
AI 理解论文
溯源树
样例
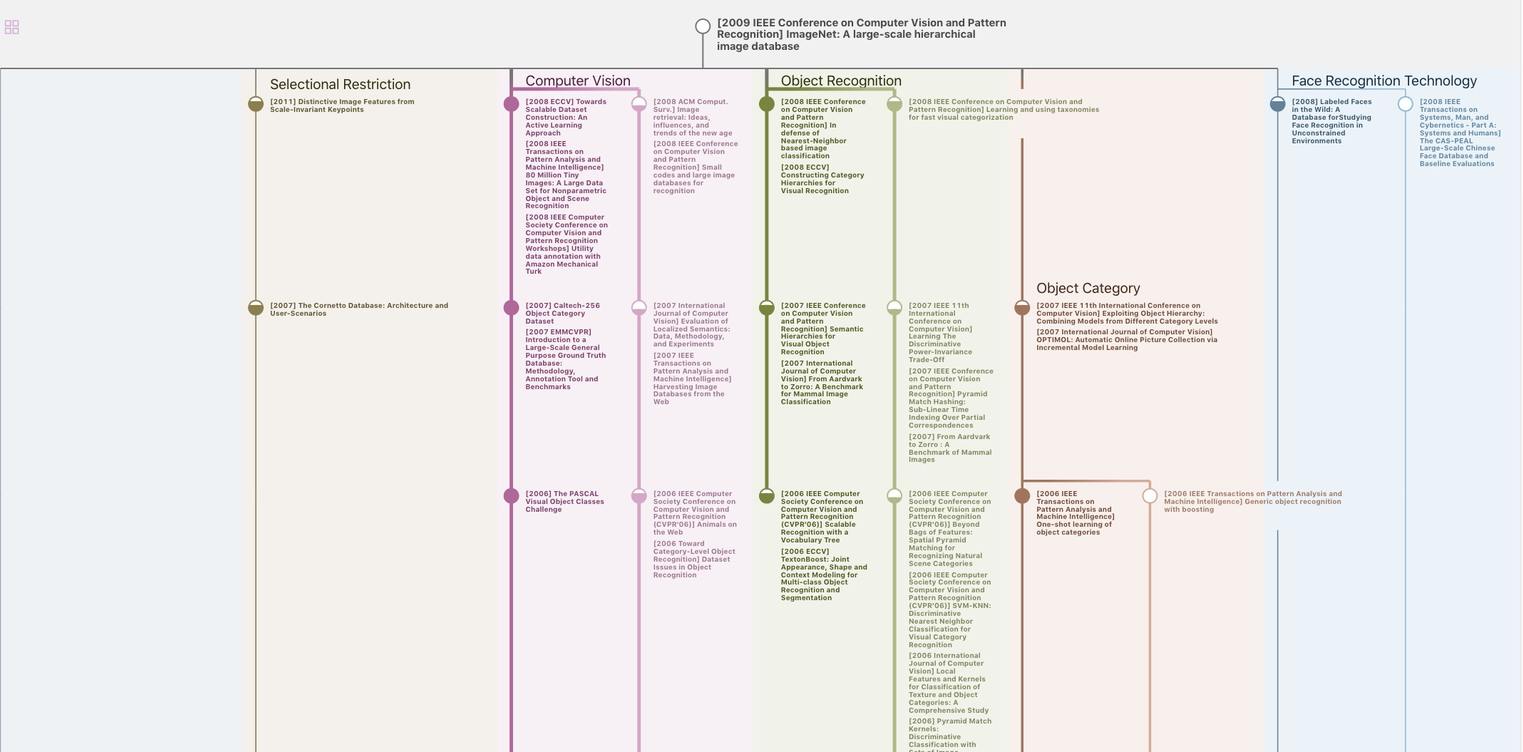
生成溯源树,研究论文发展脉络
Chat Paper
正在生成论文摘要