A Noval Weighted Meta Graph Method For Classification In Heterogeneous Information Networks
APPLIED SCIENCES-BASEL(2020)
摘要
There has been increasing interest in the analysis and mining of Heterogeneous Information Networks (HINs) and the classification of their components in recent years. However, there are multiple challenges associated with distinguishing different types of objects in HINs in real-world applications. In this paper, a novel framework is proposed for the weighted Meta graph-based Classification of Heterogeneous Information Networks (MCHIN) to address these challenges. The proposed framework has several appealing properties. In contrast to other proposed approaches, MCHIN can fully compute the weights of different meta graphs and mine the latent structural features of different nodes by using these weighted meta graphs. Moreover, MCHIN significantly enlarges the training sets by introducing the concept of Extension Meta Graphs in HINs. The extension meta graphs are used to augment the semantic relationship among the source objects. Finally, based on the ranking distribution of objects, MCHIN groups the objects into pre-specified classes. We verify the performance of MCHIN on three real-world datasets. As is shown and discussed in the results section, the proposed framework can effectively outperform the baselines algorithms.
更多查看译文
关键词
heterogeneous information networks,classification,meta graph,meta path
AI 理解论文
溯源树
样例
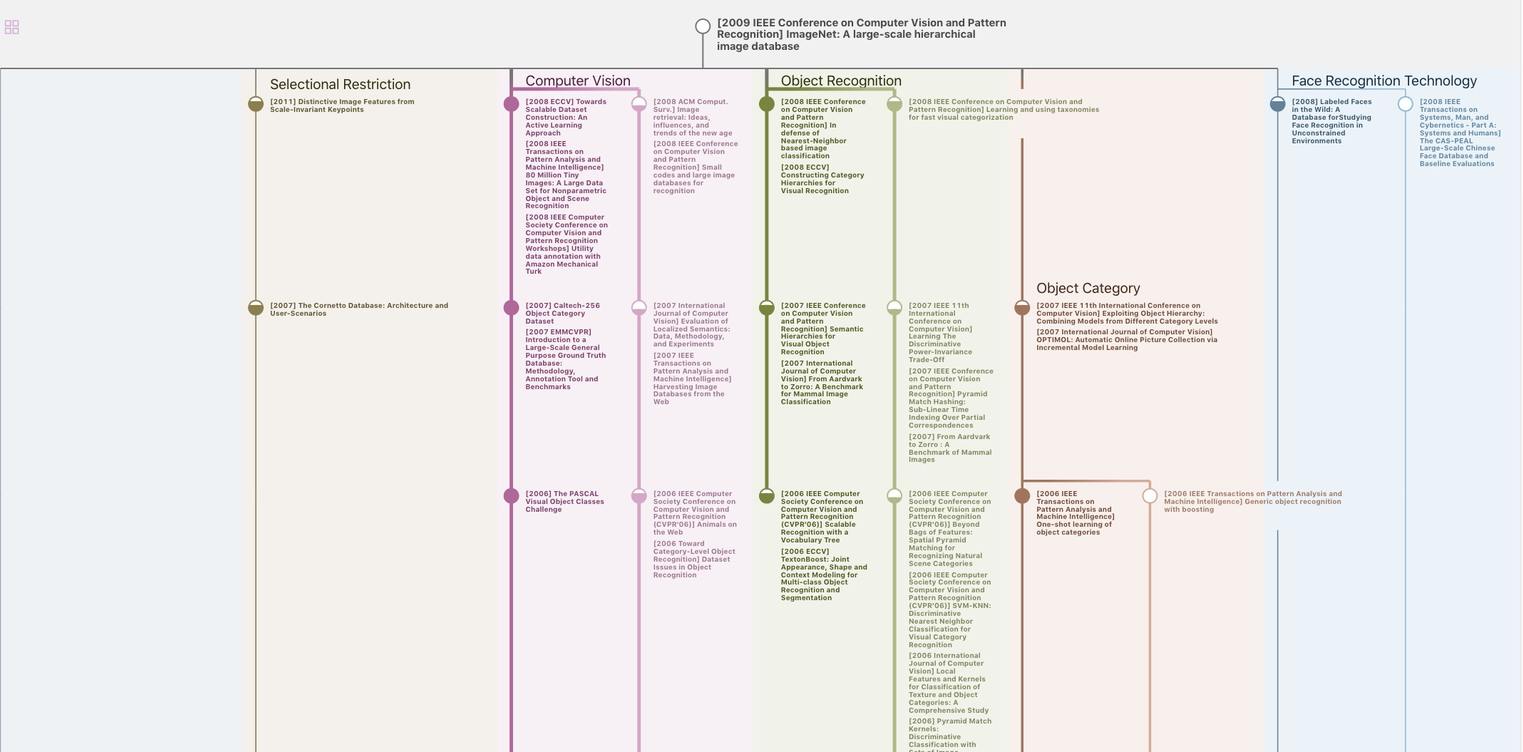
生成溯源树,研究论文发展脉络
Chat Paper
正在生成论文摘要