Evaluation of Reinforcement Learning Methods for a Self-learning System
ICAART: PROCEEDINGS OF THE 12TH INTERNATIONAL CONFERENCE ON AGENTS AND ARTIFICIAL INTELLIGENCE, VOL 2(2020)
摘要
In recent years, interest in self-learning methods has increased significantly. A challenge is to learn to survive in a real or simulated world by solving tasks with as little prior knowledge about itself, the task, and the environment. In this paper, the state of the art methods of reinforcement learning, in particular, Q-learning, are analyzed regarding applicability to such a problem. The Q-learning algorithm is completed with replay memories and exploration functions. Several small improvements are proposed. The methods are then evaluated in two simulated environments: a discrete bit-flip and a continuous pendulum environment. The result is a lookup table of the best suitable algorithms for each type of problem.
更多查看译文
关键词
Reinforcement Learning,Machine Learning,Self-learning,Neural Networks,Q-learning,Deep Q-learning,Replay Memory,Artificial Intelligence,Rewards,Algorithms
AI 理解论文
溯源树
样例
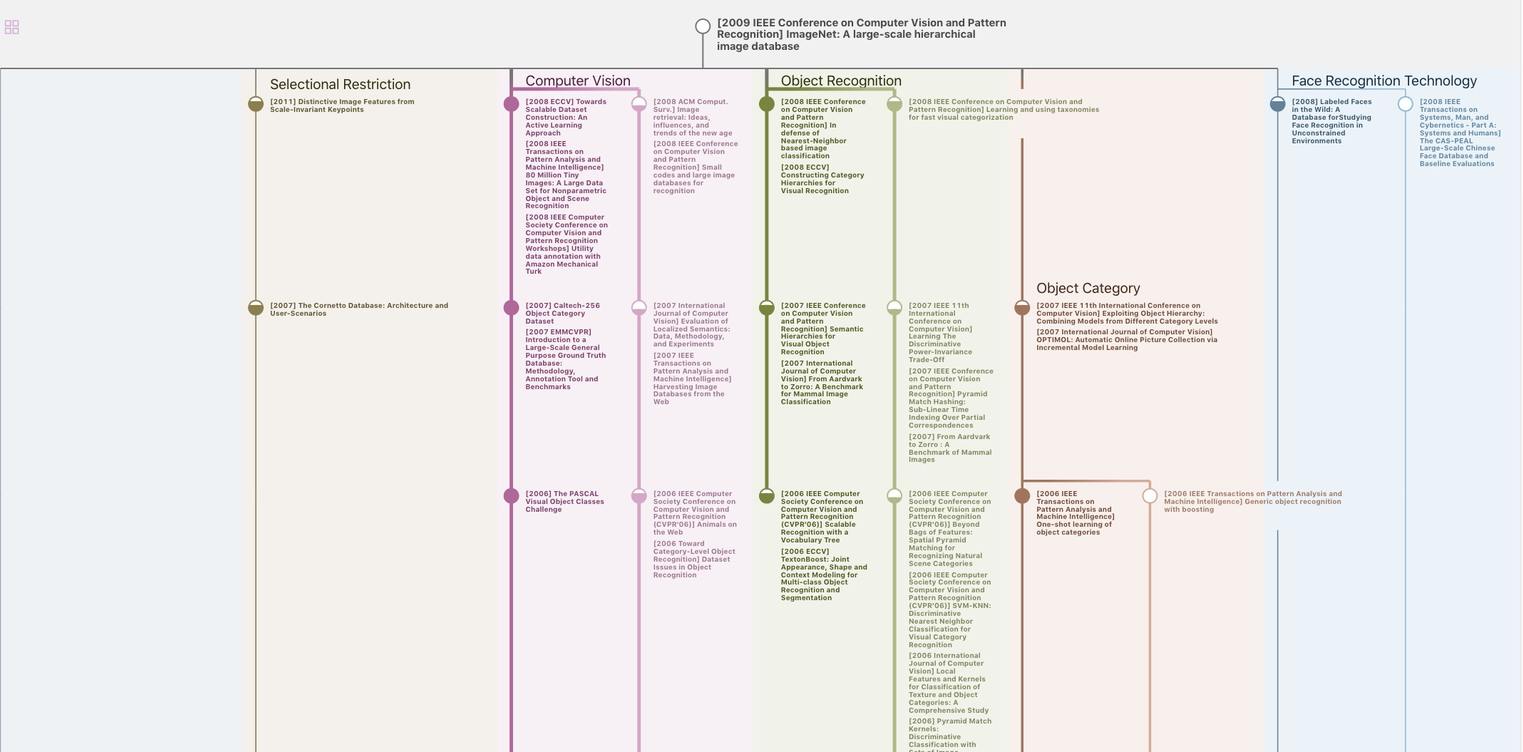
生成溯源树,研究论文发展脉络
Chat Paper
正在生成论文摘要