A Multi-View features hinged siamese U-Net for image Co-segmentation
MULTIMEDIA TOOLS AND APPLICATIONS(2020)
摘要
This paper proposes a new U-shape structure to extract multi-view features from different images which is incorporated into the network that can be trained end-to-end for image co-segmentation task. The multi-view features integrate global correlations between images, so we can segment the common objects in different images from the features directly. Before getting the multi-view features, we extract the deep features of input images through two weights-shared streams. Then we get pixel-level similarity maps through a similarity layer from deep features. The whole architecture is a Siamese U-net hinged by multi-view features, called iMFNet for short. We further introduce Dice loss and employ both positive and negative examples to train the whole network. Furthermore, a learnable conditional random field (CRF) layer is added to iMFNet for more accurate results. Using the training data from MSRC and PASCAL VOC 2012 datasets, the iMFNet achieves the state-of-the-art performance on the Internet datasets and the competitive performance on the iCoseg datasets.
更多查看译文
关键词
Image co-segmentation,Multi-view features,Siamese U-Net
AI 理解论文
溯源树
样例
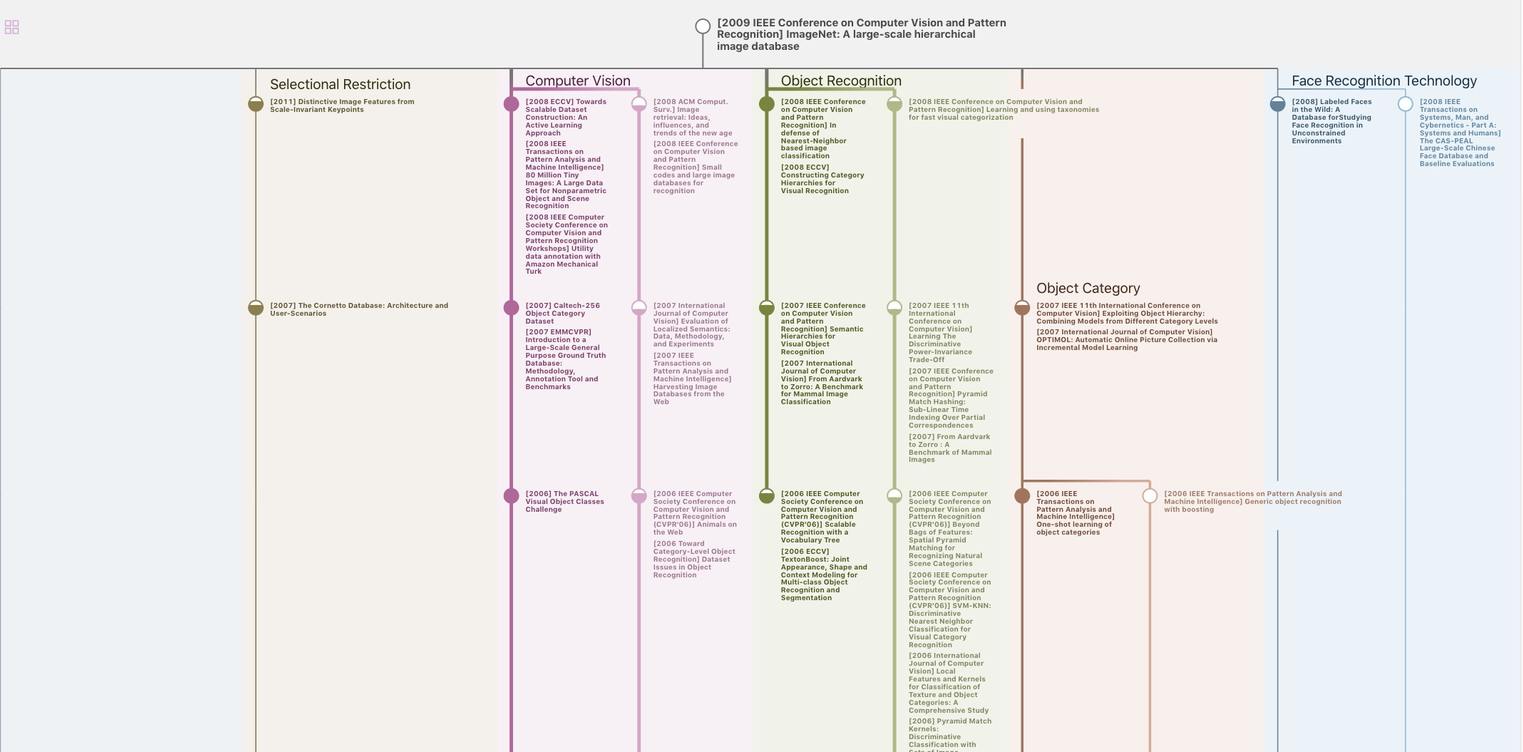
生成溯源树,研究论文发展脉络
Chat Paper
正在生成论文摘要