Bayesian Identification Of State-Space Models Via Adaptive Thermostats
2019 IEEE 58TH CONFERENCE ON DECISION AND CONTROL (CDC)(2019)
摘要
Bayesian modeling has been recognized as a powerful approach to system identification, not least due to its intrinsic uncertainty quantification. However, despite many recent developments, Bayesian identification of nonlinear state space models still poses major computational challenges. We propose a new method to tackle this problem. The technique is based on simulating a so-called thermostat, a stochastic differential equation constructed to have the posterior parameter distribution as its limiting distribution. Simulating the thermostat requires access to unbiased estimates of the gradient of the log-posterior. To handle this, we make use of a recent method for debiasing particle-filter-based smoothing estimates. Numerical results show a clear benefit of this approach compared to a direct application of (biased) particle-filter-based gradient estimates within the thermostat.
更多查看译文
关键词
particle-filter-based gradient estimates,limiting distribution,posterior parameter distribution,stochastic differential equation,nonlinear state space models,Bayesian identification,intrinsic uncertainty quantification,system identification,Bayesian modeling,state-space models
AI 理解论文
溯源树
样例
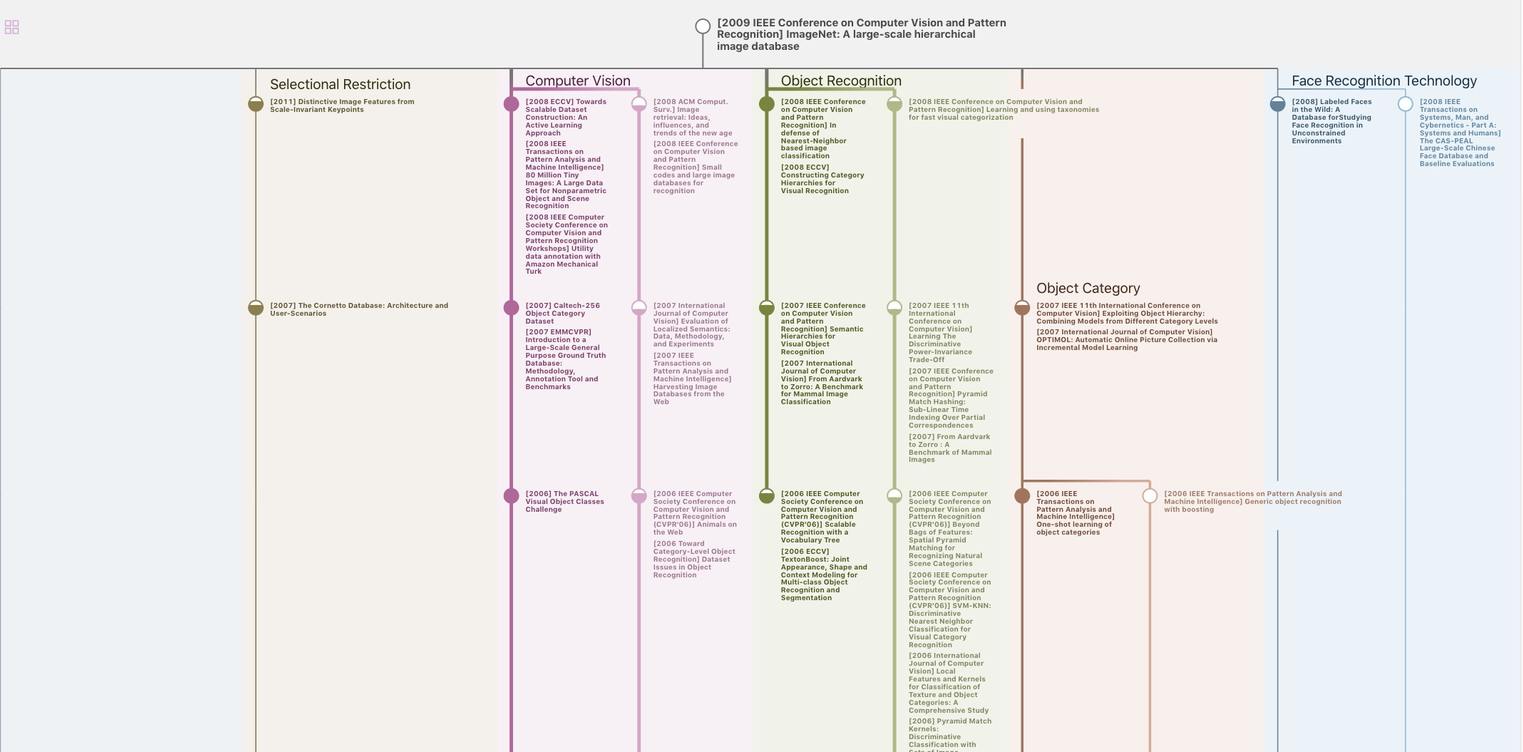
生成溯源树,研究论文发展脉络
Chat Paper
正在生成论文摘要