Distributed Learning In Network Games: A Dual Averaging Approach
2019 IEEE 58TH CONFERENCE ON DECISION AND CONTROL (CDC)(2019)
摘要
In this paper, we propose a distributed no-regret learning algorithm for network games using a primal-dual method, i.e., dual averaging. With only locally available observations, we consider the scenario where each player optimizes a global objective, formed by local objective functions on the nodes of a given communication graph. Our learning algorithm for each player involves taking steps along their individual payoff gradients, dictated by local observations of the other player's actions. The output is then projected back-again locally-to the set of admissible actions for each player. We provide the regret analysis of this distributed learning algorithm for the case of a deterministic network that is subjected to two teams with distinct objectives, and obtain an O(root T log(T)) regret bound. Our analysis indicates the key correlation between the rate of convergence and network connectivity that also appears in the distributed optimization setup via dual averaging. Furthermore, we show that the point of convergence of the proposed algorithm would be a Nash Equilibrium of the game. Finally, we showcase by an illustrative example the performance of our algorithm in relation to the size and connectivity of the network.
更多查看译文
关键词
network games,dual averaging approach,distributed no-regret,primal-dual method,objective functions,communication graph,individual payoff gradients,admissible actions,regret analysis,distributed learning algorithm,deterministic network,distributed optimization setup,Nash equilibrium
AI 理解论文
溯源树
样例
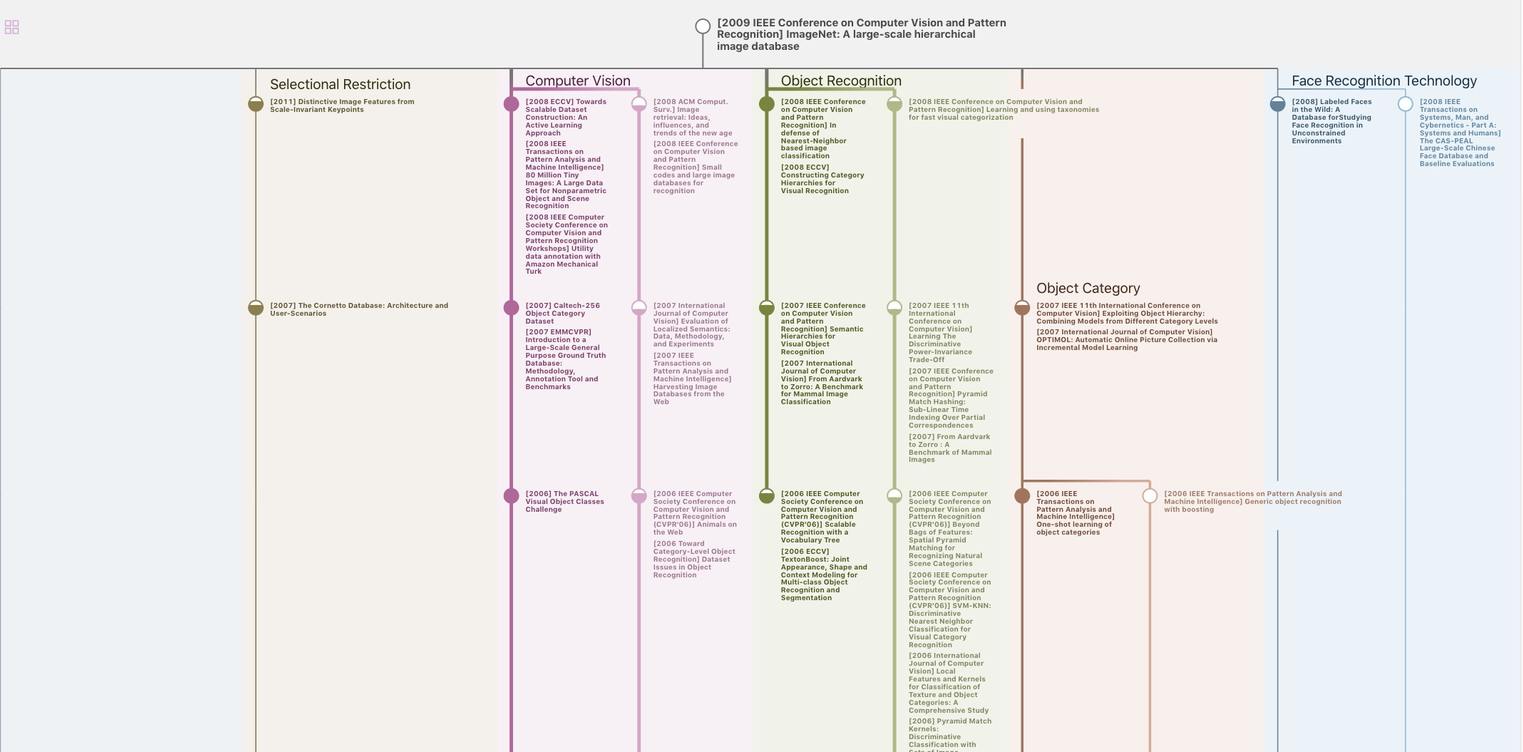
生成溯源树,研究论文发展脉络
Chat Paper
正在生成论文摘要