Towards Evaluating Performance of Domain Specific Transfer Learning for Pneumonia Detection from X-Ray Images
2019 IEEE 5TH INTERNATIONAL CONFERENCE FOR CONVERGENCE IN TECHNOLOGY (I2CT)(2019)
摘要
Medical diagnosis and treatment is a field where Artificial Intelligence (AI) has the potential to provide tremendous scope for targeted large-scale interventions. Trained on vast troves of digitized slides showing an enormous variety of tumors, AI systems will likely provide more accurate diagnoses than human pathologists, at least on fairly rote diagnostic tasks. To harness the power of deep learning using Convolutional Neural Networks (CNN), there arises a difficulty in training a CNN from scratch because it requires large amount of computing power and also large amount of training data. The latter aspect in training a CNN turns out to be an obstacle in the case of medical image analysis. Previous works have addressed this difficulty and have demonstrated that the use of pre-trained deep CNNs with sufficient training provide a performance better than training a CNN from scratch. In this paper, we provide a comparison of the results obtained by using weights pre-trained on medical images from CheXNet dataset with the results obtained by using weights pre-trained on the ImageNet dataset. We performed experiments on the Chest X-ray dataset obtained from Kaggle. A 121-layer DenseNet when trained on top of a finely tuned CheXNet model, a test accuracy of 88.78% was obtained with precision, recall and F1-score of 0.71, 0.97 and 0.81 respectively. Our experiments consistently demonstrated that: 1) A finely tuned deep CNN model when used as a base for training dataset of medical images provided better accuracy than the CNN model trained from scratch by generating initial random weights. 2) The use of deep CNN's pre-trained weights on a medical image dataset provides better performance for medical image classification in terms of accuracy, precision and recall, than models trained on natural images like ImageNet dataset.
更多查看译文
关键词
Deep Learning, Medical Imaging, Transfer Learning
AI 理解论文
溯源树
样例
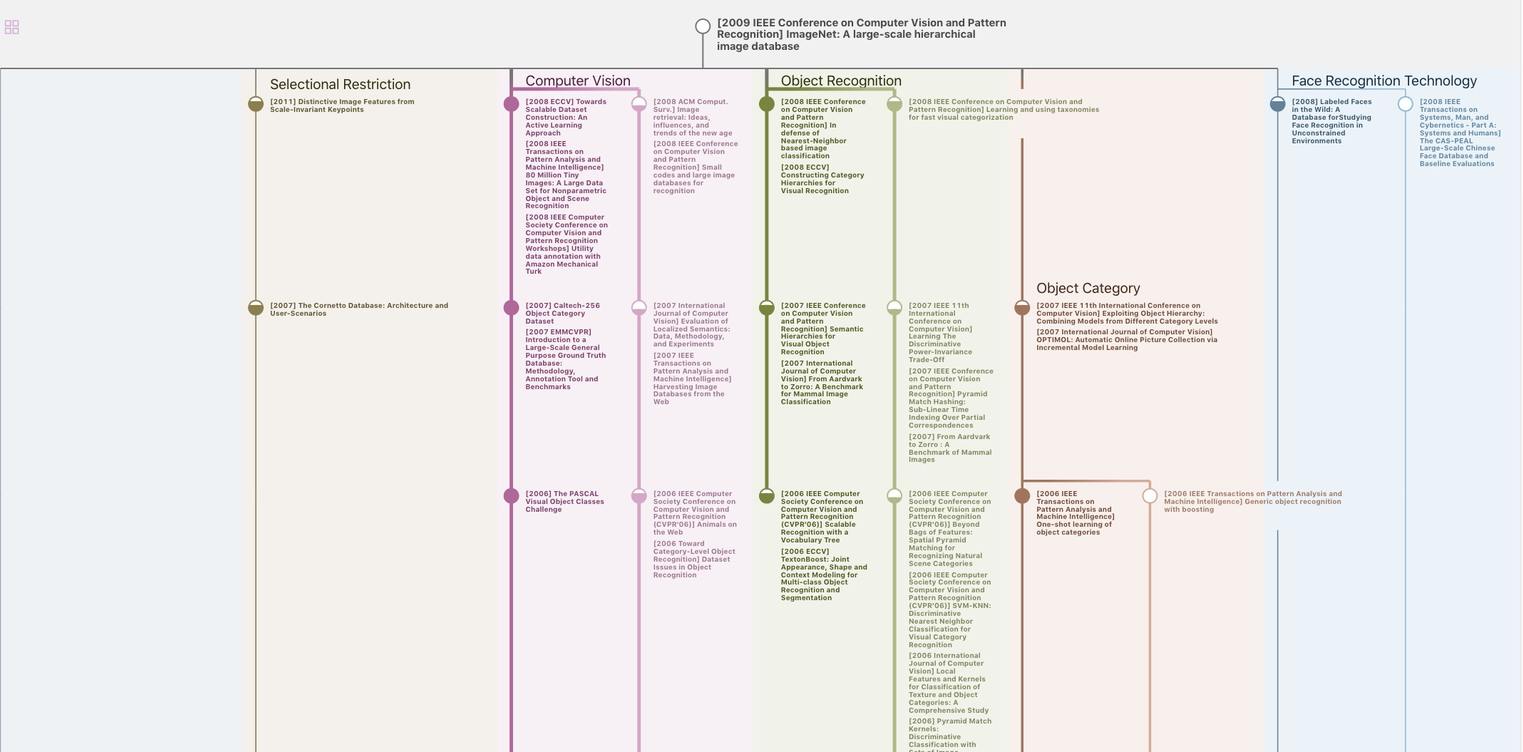
生成溯源树,研究论文发展脉络
Chat Paper
正在生成论文摘要