Bearing Fault Diagnosis Using Grad-CAM and Acoustic Emission Signals
APPLIED SCIENCES-BASEL(2020)
摘要
Bearing failure generates impulses when the rolling elements pass the cracked surface of the bearing. Over the past decade, acoustic emission (AE) techniques have been used to detect bearing failures operated in low-rotating speeds. However, since the high sampling rates of the AE signals make it difficult to design and extract discriminative fault features, deep neural network-based approaches have been proposed in several recent studies. This paper proposes a convolutional neural network (CNN)-based bearing fault diagnosis technique. In this work, the normalized bearing characteristic component (NBCC) is used as the input of CNN, which is an effective form of representing bearing failure symptoms. In addition, importance-weight is extracted using gradient-weighted class activation mapping (Grad-CAM) for visual explanation of CNN. In the experiment result, the proposed approach achieves high classification accuracy with reasonable visualization, which shows that CNN successfully learned the components of bearing characteristic frequency for each type of bearing failure.
更多查看译文
关键词
acoustic emission,bearing fault diagnosis,deep neural network,Grad-CAM
AI 理解论文
溯源树
样例
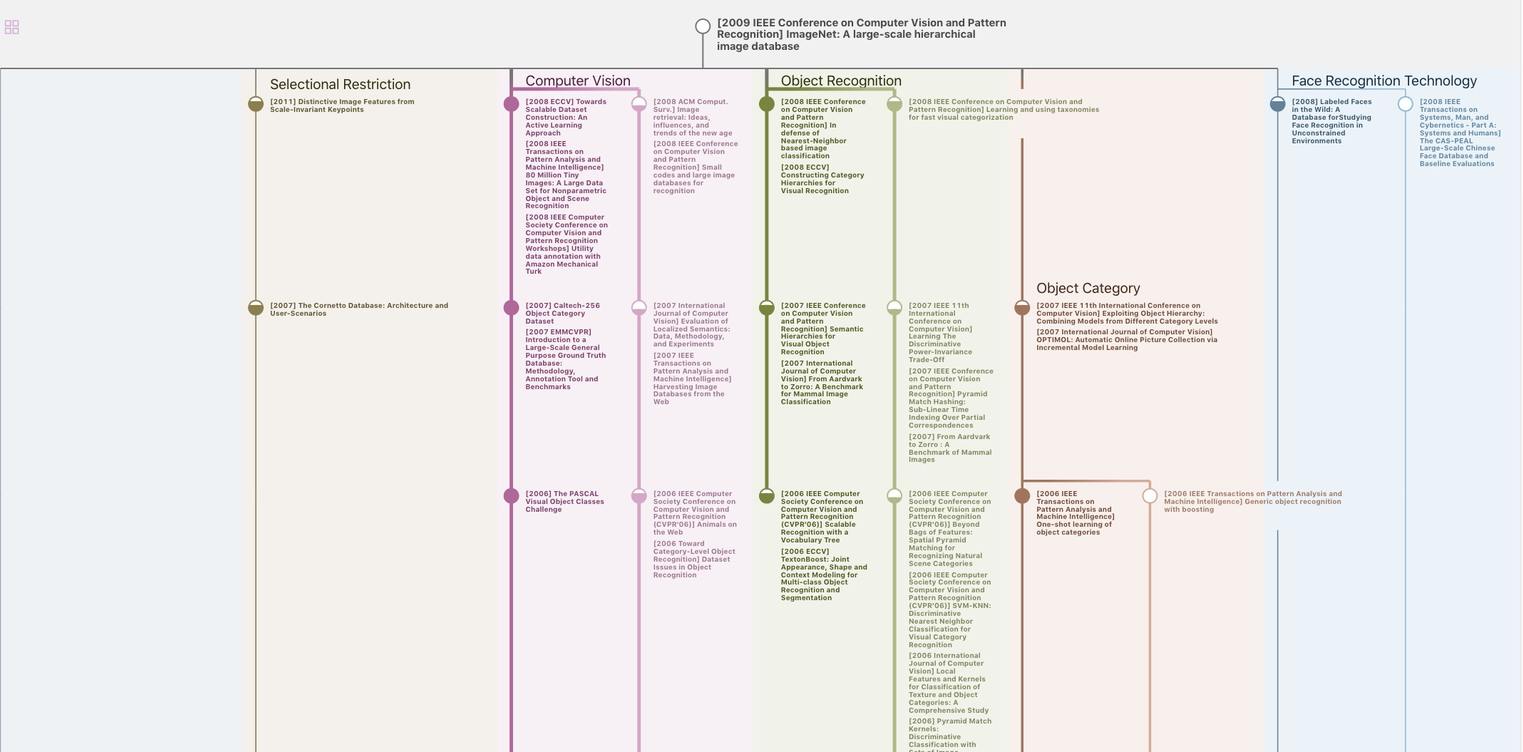
生成溯源树,研究论文发展脉络
Chat Paper
正在生成论文摘要