Numerical sensitive data recognition based on hybrid gene expression programming for active distribution networks
Applied Soft Computing(2020)
摘要
Complex and flexible access mode, and frequent data interaction bring about large security risks to data transmission for active distribution networks. How to ensure data security is critical to the safe and stable operation of active distribution networks. Traditional methods, like access control, data encryption, and text filtering based on intelligent algorithms, are difficult to ensure the security of dynamically increased and high-dimensional numerical data transmission in active distribution networks. In this paper, we first propose a rough feature selection algorithm based on the average importance measurement (RFS-AIM) to simplify the complexity of data recognition. Then, we propose a sensitive data recognition function mining algorithm based on RFS-AIM and improved gene expression programming (SDR-IGEP) where population update operation is constructed by chromosome similarity based on the Jaccard coefficient. The operation avoids local convergence of the gene express programming by increasing individual diversity in the new population. Finally, we present a new incremental mining algorithm for a sensitive data recognition function based on global function fitting (ISDR-GFF) by using a grain granulation model for incremental datasets. The experimental results on IEEE benchmark datasets and real datasets show that the algorithms proposed in this paper outperform the state-of-the-art algorithms in terms of the average running time, precision, recall, F1 index, accuracy, specificity and speedup on all experimental datasets.
更多查看译文
关键词
Function mining,Active distribution network,Sensitive data recognition,Gene expression programming,Rough set
AI 理解论文
溯源树
样例
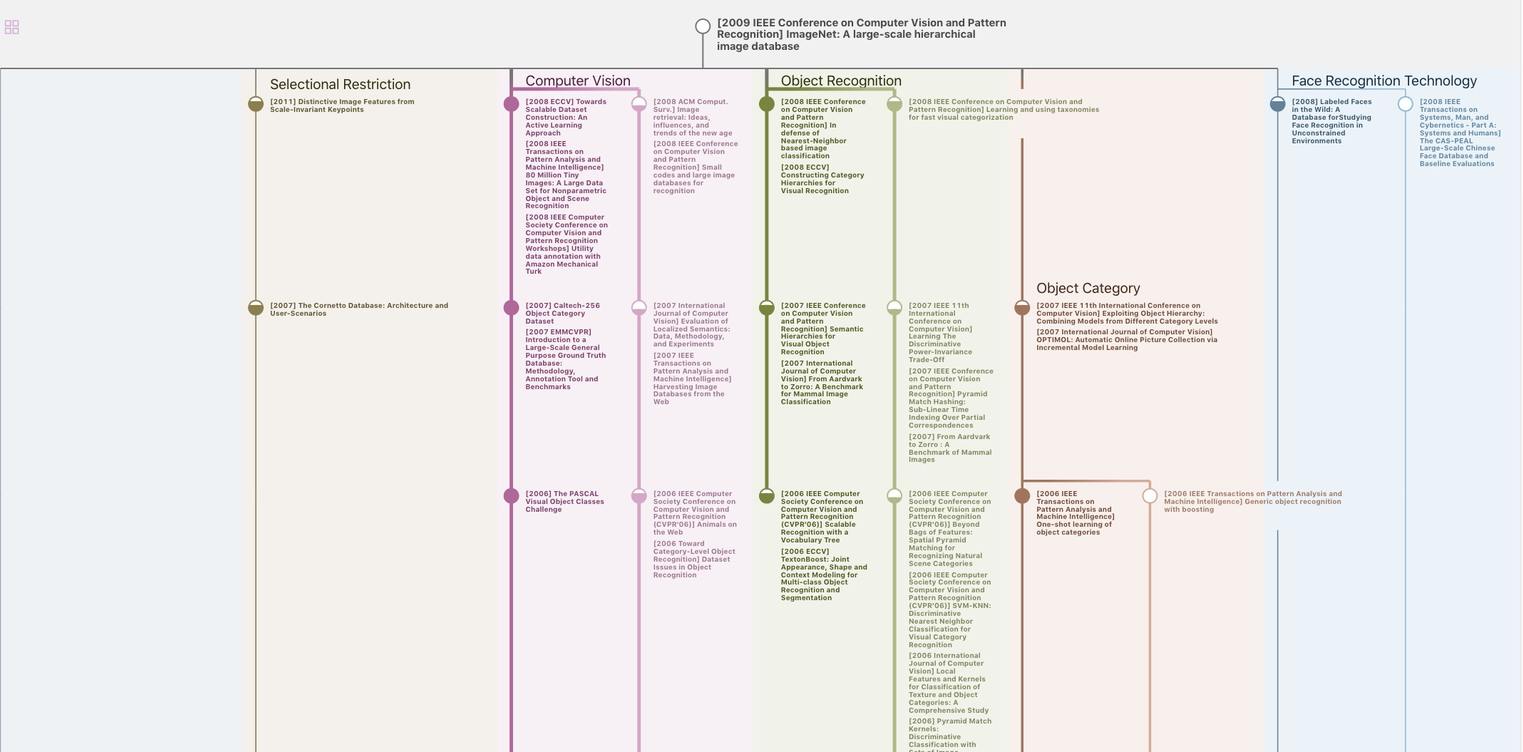
生成溯源树,研究论文发展脉络
Chat Paper
正在生成论文摘要