Correlational Neural Network Based Feature Adaptation in L2 Mispronunciation Detection
2019 International Conference on Asian Language Processing (IALP)(2019)
摘要
Due to the difficulties of collecting and annotating second language (L2) learner's speech corpus in Computer-Assisted Pronunciation Training (CAPT), traditional mispronunciation detection framework is similar to ASR, it uses speech corpus of native speaker to train neural networks and then the framework is used to evaluate non-native speaker's pronunciation. Therefore there is a mismatch between them in channels, reading style, and speakers. In order to reduce this influence, this paper proposes a feature adaptation method using Correlational Neural Network (CorrNet). Before training the acoustic model, we use a few unannotated non-native data to adapt the native acoustic feature. The mispronunciation detection accuracy of CorrNet based method has improved 3.19% over un-normalized Fbank feature and 1.74% over bottleneck feature in Japanese speaking Chinese corpus. The results show the effectiveness of the method.
更多查看译文
关键词
Computer-Assisted Pronunciation Training,Correlational Neural Network,Bottleneck feature
AI 理解论文
溯源树
样例
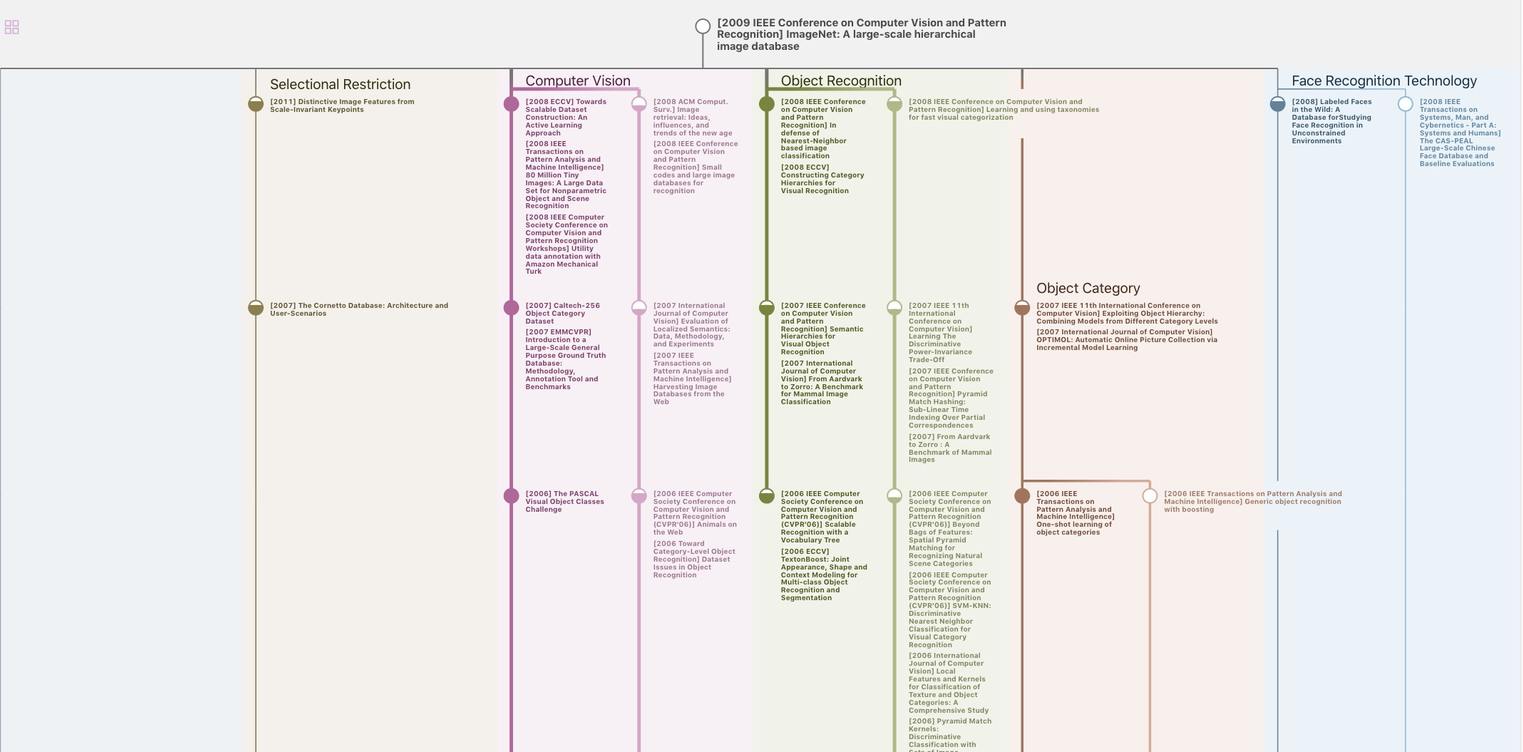
生成溯源树,研究论文发展脉络
Chat Paper
正在生成论文摘要