Reducing Traffic Congestion by LSTM-LOF Framework
2019 IEEE/ACS 16th International Conference on Computer Systems and Applications (AICCSA)(2019)
摘要
Traffic congestion is a phenomenon with which most drivers are familiar. It negatively affects drivers, city's residents and also the economic efficiency. Road pavement condition is one of the main causes of traffic congestion. Warning drivers of road pavement conditions is an effective and highly recommended solution to prevent a traffic jam before it actually occurs. Through this paper, we present a new strategy to reduce traffic congestion. We propose an unsupervised anomaly detection framework that predicts road obstacles. We suggest a well-motivated combination between the Long Short Term Memory (LSTM) and Local outlier value factor (LOF) techniques for predicting obstacles in time series. This combination can potentially offer very promising results in terms of prediction accuracy. We illustrate significant performance gains achieved by our technique with respect to the conventional methods.
更多查看译文
关键词
Anomaly detection,LSTM,LOF,Time series
AI 理解论文
溯源树
样例
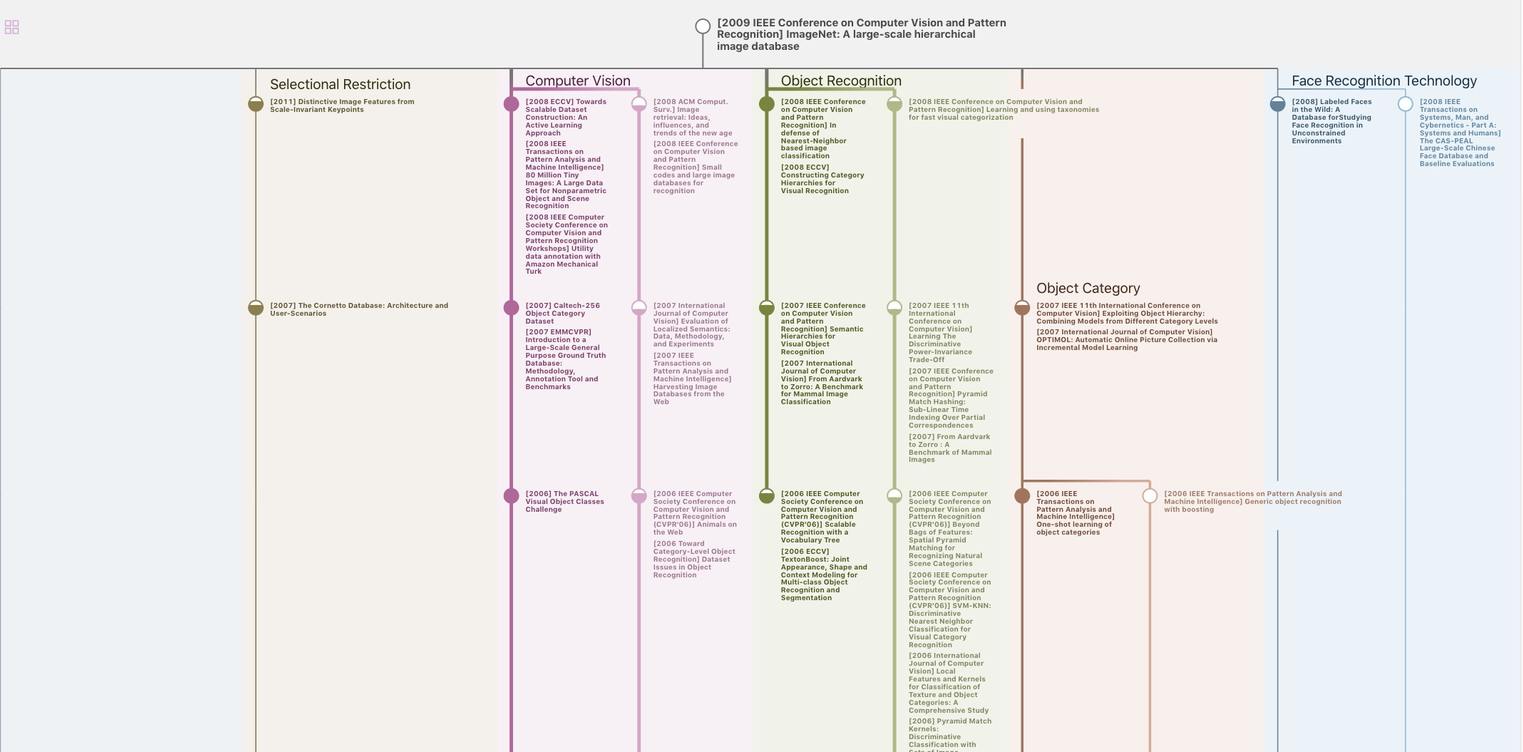
生成溯源树,研究论文发展脉络
Chat Paper
正在生成论文摘要