Adaptive collaborative graph for discriminant analysis of hyperspectral imagery
EUROPEAN JOURNAL OF REMOTE SENSING(2020)
摘要
Recently, sparse graph-based discriminant analysis (SGDA) and collaborative graph-based discriminant analysis (CGDA) have been developed for dimensionality reduction of hyperspectral imagery. In SGDA or CGDA, a graph is constructed by l(1)-norm minimization-based representation or l(2)-norm minimization-based representation, respectively, based on labeled samples. These two methods have shown success over traditional methods by reinforcing discriminative power, but are limited in within-class representation and distribution of representation coefficients. In order to preserve intrinsic geometrical structure of original data and improve the interpretability of the underlying graph, we propose an adaptive collaborative graph for discriminant analysis (ACGDA), which explicitly models the internal relationship among within-class pixels during graph construction. The proposed method couples distance-weighted Tikhonov regularization with l(2)-norm minimization-based representation, of which the coefficients are solutions to a closed-form expression. The graph adaptively adjusts the collaborative representation by using distance-weighted measurement, which produces stronger ability of discrimination. In addition, the graph weight matrix is designed in the form of a block-diagonal structure, reducing the computational cost and further improving discriminative power. The proposed approach is compared with several traditional and state-of-the-art methods on two benchmark datasets. Experimental results demonstrate that the proposed approach can yield superior classification performance.
更多查看译文
关键词
Hyperspectral classification,dimensionality reduction,collaborative graph,sparse representation
AI 理解论文
溯源树
样例
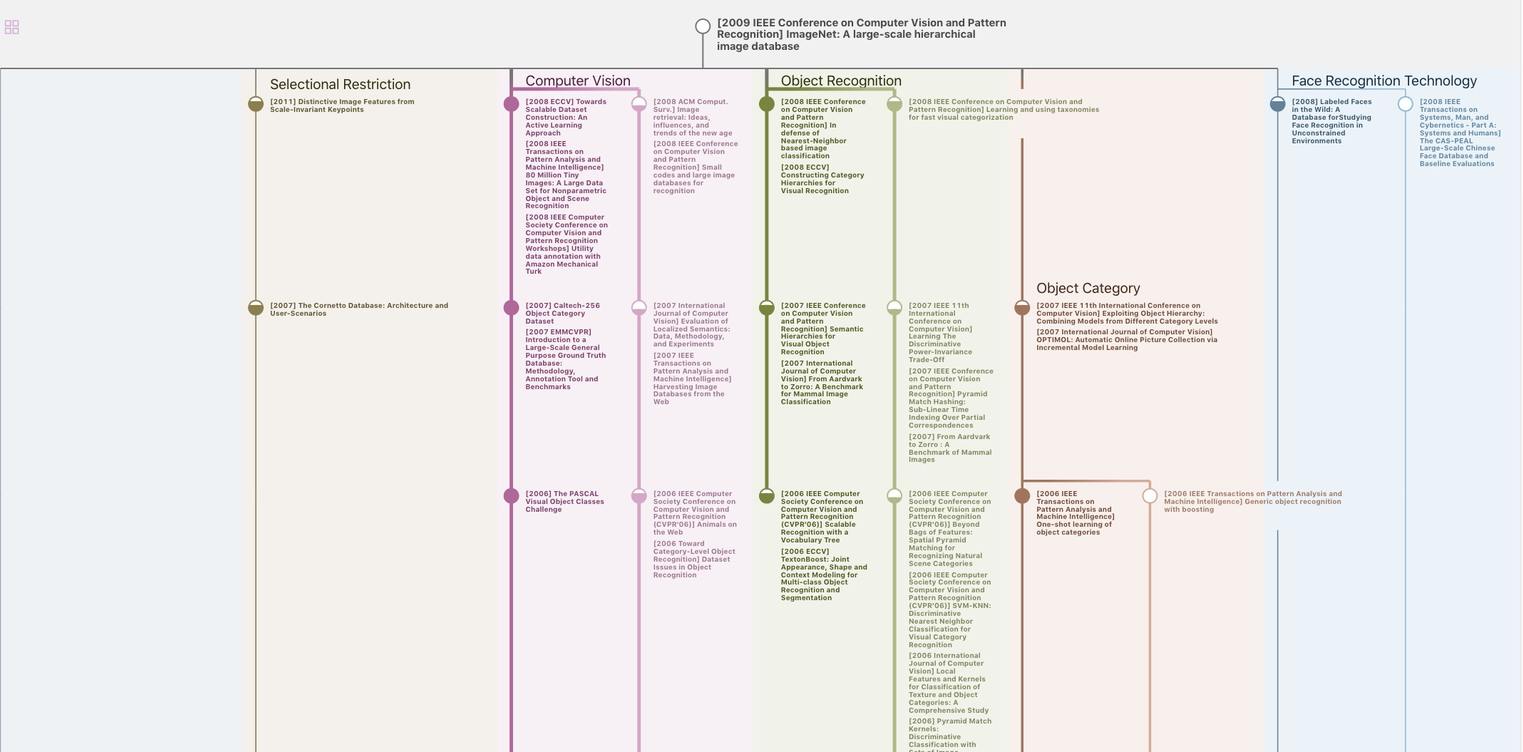
生成溯源树,研究论文发展脉络
Chat Paper
正在生成论文摘要