Microphone array speech enhancement using LSTM neural network
2019 17th International Conference on Emerging eLearning Technologies and Applications (ICETA)(2019)
摘要
The article encompasses microphone array speech processing using neural networks. Noisy microphone array, which consists of 12 elements, is simulated from clean and noise mono-channel speech recordings with the utilization of open custom-modified software framework MCRoomSim, which is executable in an integrated development environment called MATLAB. The modified framework applies beamforming methods, e.g. Frost algorithm in order to suppress noise signal, this is known as primary speech enhancement. Such beamformed signal is filtrated by the application of the Wiener filter, which is predicted from noisy speech spectrograms using a deep neural network model. This neural network predicted Wiener filter, originally calculated out of spectrograms, is subsequently multiplied with beamformed signal for the purpose of secondary speech enhancement. The latter way of speech enhancement is generally called beamforming with post-filtering. There are various parameters for objective evaluation of speech enhancement effectivity, whether concerning the very beamforming or application of neural network, i.e. STOI, PESQ, MOS-LQO, and even fwSNRseg. The beneficiality of beamforming is discussed in the last chapter of this paper.
更多查看译文
关键词
microphone array speech enhancement,LSTM neural network,noisy microphone array,clean noise mono-channel speech recordings,open custom-modified software framework MCRoomSim,integrated development environment,modified framework,noise signal,primary speech enhancement,beamformed signal,Wiener filter,noisy speech spectrograms,deep neural network model,secondary speech enhancement,speech enhancement effectivity
AI 理解论文
溯源树
样例
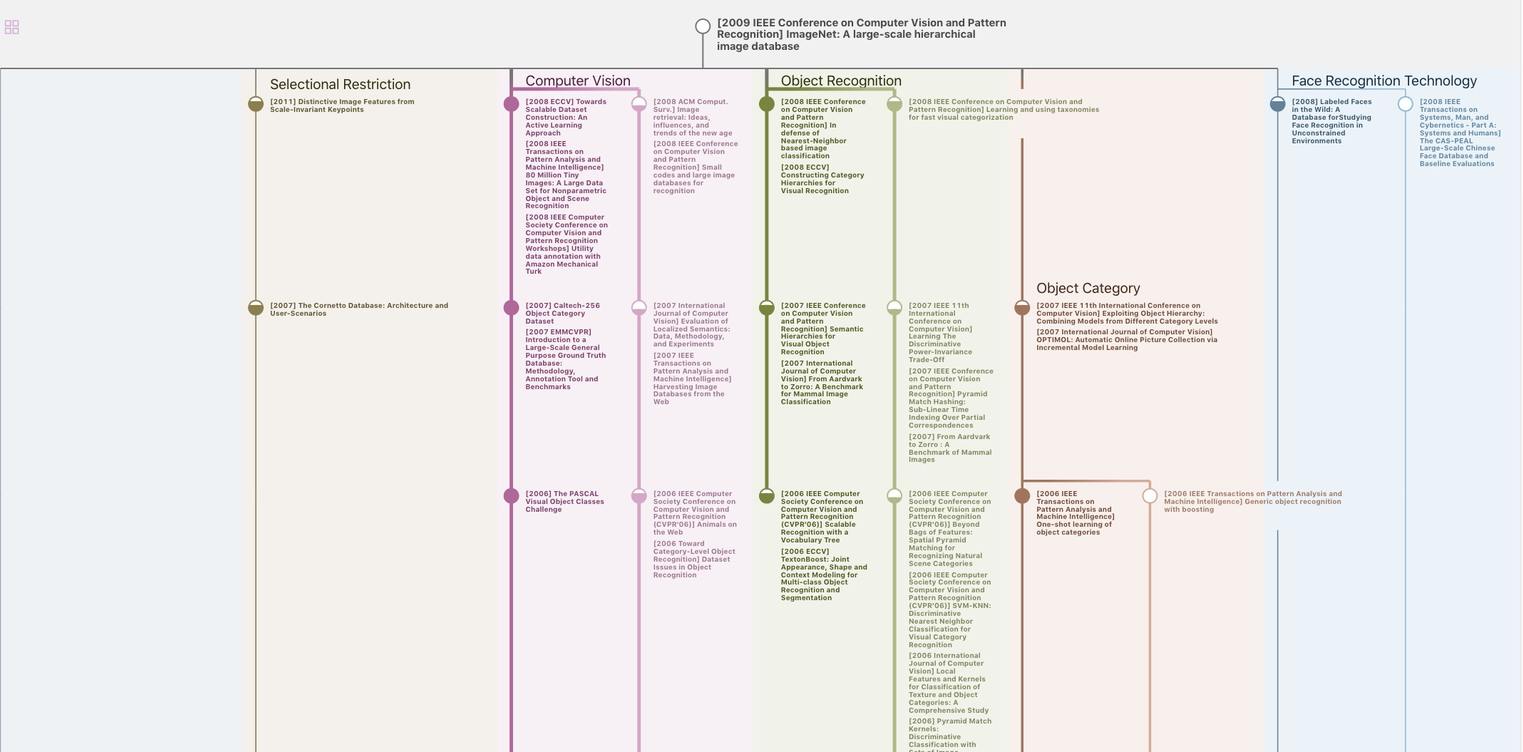
生成溯源树,研究论文发展脉络
Chat Paper
正在生成论文摘要