Global Optimization For Mixed Categorical-Continuous Variables Based On Gaussian Process Models With A Randomized Categorical Space Exploration Step
INFOR(2020)
摘要
Real industrial studies often give rise to complex optimization problems involving mixed variables and time consuming simulators. To deal with these difficulties we propose the use of a Gaussian process regression surrogate with a suitable kernal able to capture simultaneously the output correlations with respect to continuous and categorical/discrete inputs without relaxation of the categorical variables. The surrogate is integrated into the Efficient Global Optimization method based on the maximization of the Expected Improvement criterion. This maximization is a Mixed Integer Non-Linear problem which is solved by means of an adequate optimizer: the Mesh Adaptive Direct Search, integrated into the NOMAD library. We introduce a random exploration of the categorical space with a data-based probability distribution and we illustrate the full strategy accuracy on a toy problem. Finally we compare our approach with other optimizers on a benchmark of functions.
更多查看译文
关键词
Derivative free optimization, surrogate models, EGO, NOMAD, categorical variables
AI 理解论文
溯源树
样例
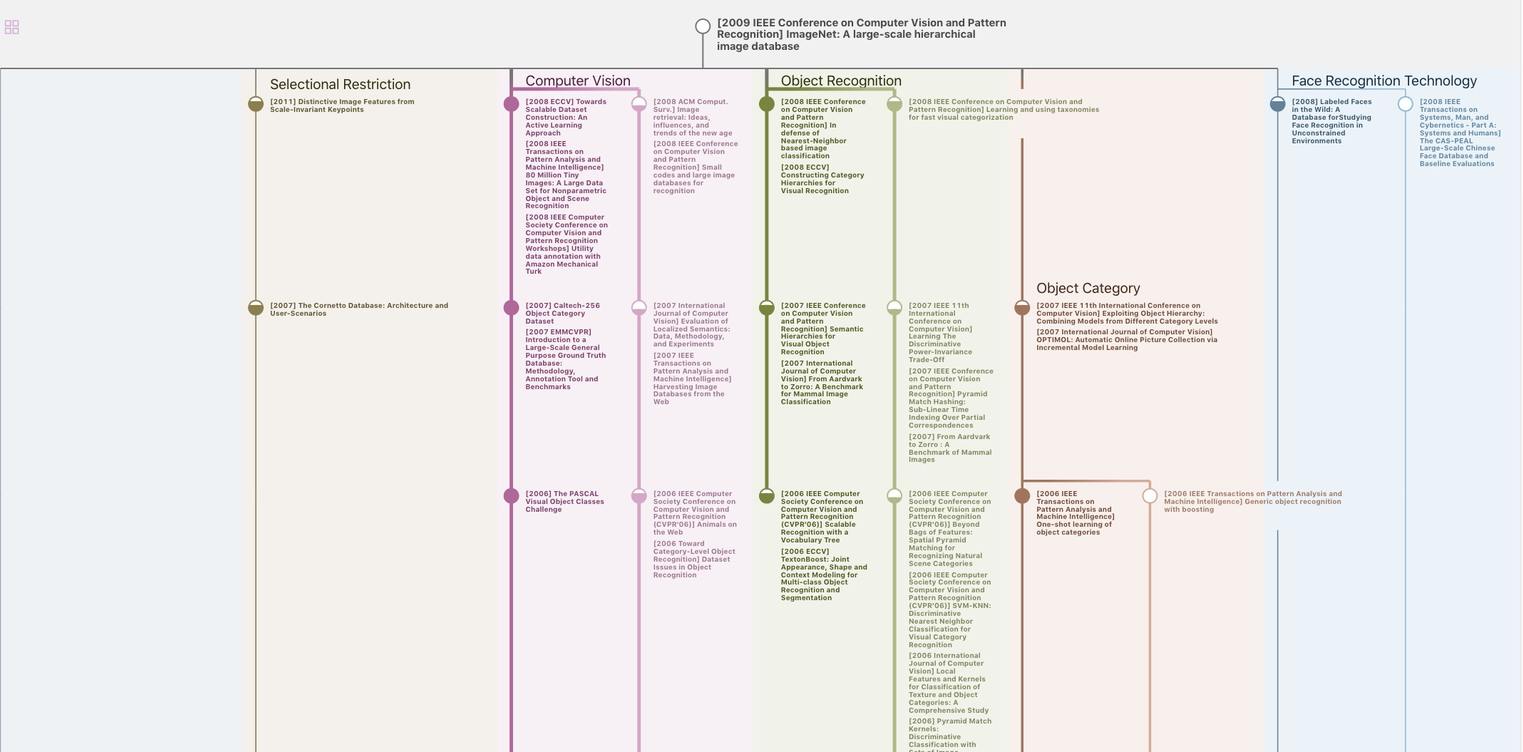
生成溯源树,研究论文发展脉络
Chat Paper
正在生成论文摘要