Retail Demand Forecasting: a Comparison Between Deep Neural Network and Gradient Boosting Method for Univariate Time Series
2019 IEEE 5TH INTERNATIONAL CONFERENCE FOR CONVERGENCE IN TECHNOLOGY (I2CT)(2019)
摘要
The traditional retailer had to deal with stocking of inventory and merchandising based on raw estimates of his daily or weekly product sales to make available the right product at the right time for his customers. The modern retailer has to deal with same store operations such as inventory planning and merchandising but with several forecasting techniques at hand such as ARIMA, Holt-Winters, Exponential Smoothing, Neural Networks and Gradient Boosting Methods, he is efficiently and more accurately able to predict store-product sales to replenish inventory with minimum capital requirement. This paper aims at implementation and comparison of two popular and intriguing machine learning techniques, Deep Neural Network (DNN) and Gradient Boosting Method (GBM) for univariate time series sales data at store-day level of a German retail giant. Several studies have leveraged DNN and GBM techniques for forecasting but on a multivariate time series data, that is predicting with the help of causal factors (variables). The paper discusses the feasibility and application of both these techniques on a univariate time series because not every retailer may have access to adequate supply chain metrics to create a multivariate model. The data used for analysis has been picked up from Kaggle. Performance measures used are mean absolute error (MAE) and root mean square error (RMSE). The DNN and GBM models have been developed in python using Keras and Ensemble packages.
更多查看译文
关键词
deep neural networks,gradient boosting,retail demand forecast,univariate time series,keras
AI 理解论文
溯源树
样例
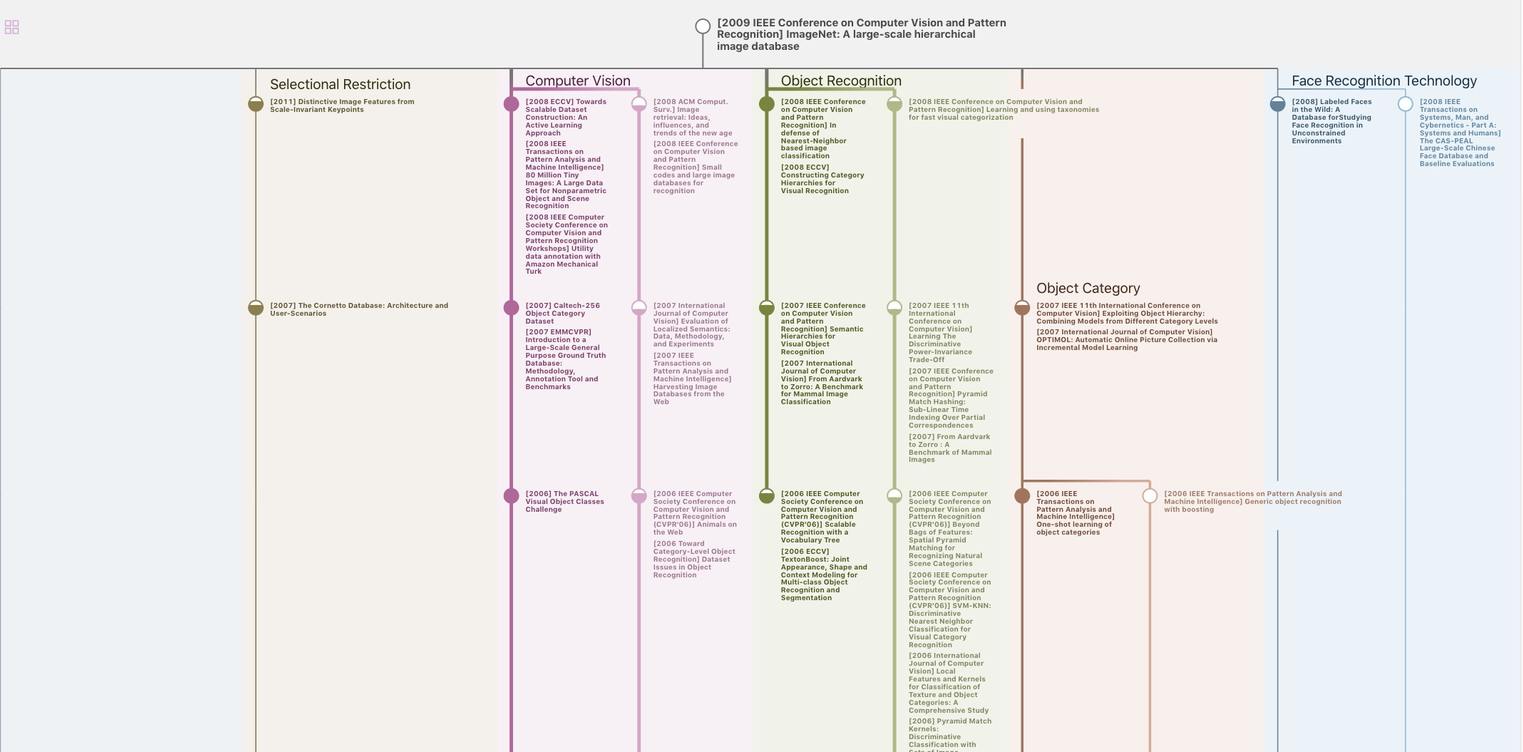
生成溯源树,研究论文发展脉络
Chat Paper
正在生成论文摘要