Modeling stress-strain curves with neural networks: a scalable alternative to the return mapping algorithm
Computational Materials Science(2020)
摘要
Modeling stress-strain curves in mechanics of material needs deriving suitable constitutive equations and solving those equations using some Euler-like scheme in th more general setting of a return mapping algorithm. However, such an approach which is based on establishing a framework that is thermodynamically consistent and physically motivated may require a substantial theoretical and experimental work. In order to bypass this traditional scheme, we consider an alternative data-driven approach motivated by tools developed in the larger scope of machine learning. In particular, we show how feedforward neural networks have the ability to accurately model stress-strain relationships for an isotropic and rate independent elastoplastic solid. Taking into account the experimental cost of producing many measurements and the computational cost associated with the training of neural networks, we elaborate a full end-to-end methodology to model stress-strain curves with neural networks in a low-data regime.
更多查看译文
关键词
Neural Networks,Return Mapping Algorithm,Constitutive Equations
AI 理解论文
溯源树
样例
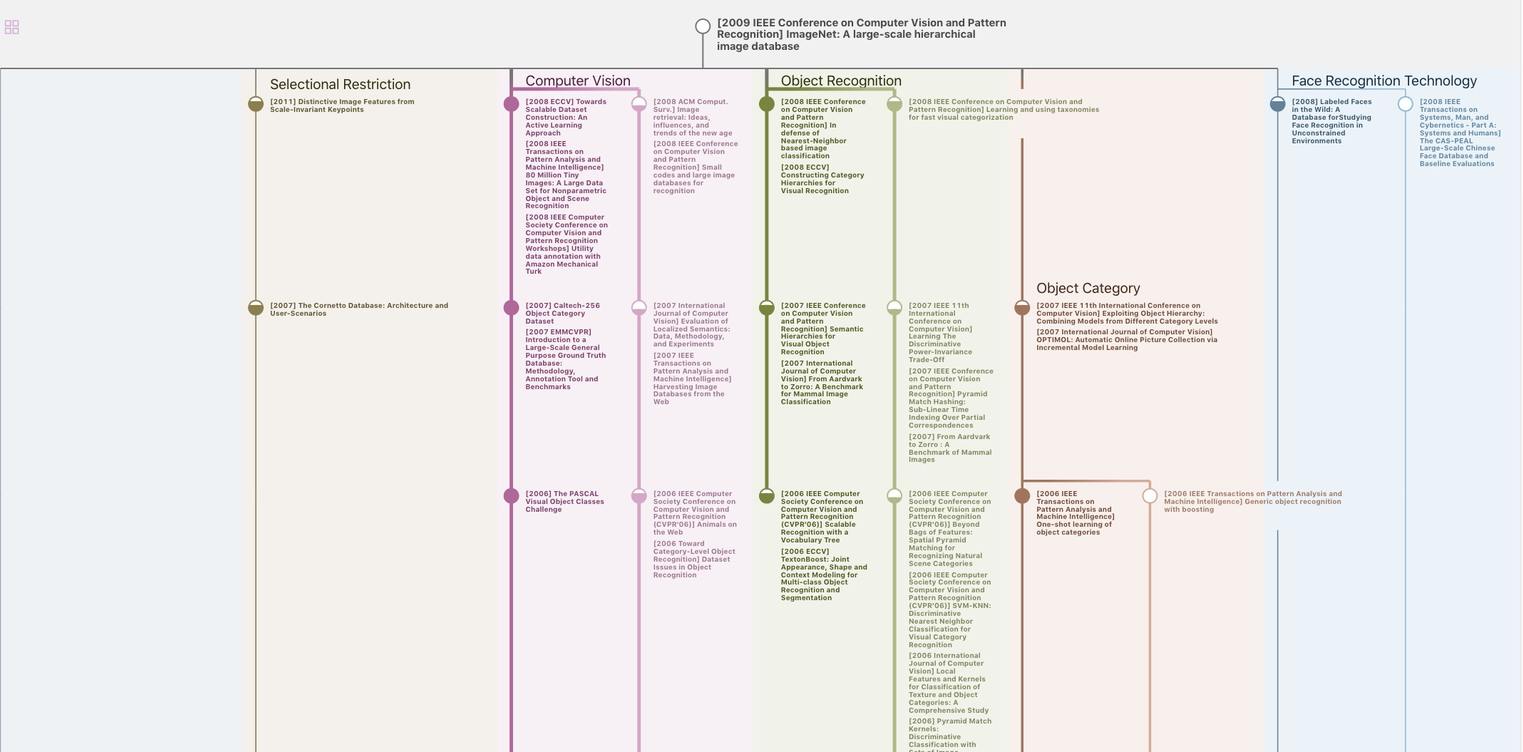
生成溯源树,研究论文发展脉络
Chat Paper
正在生成论文摘要