A Measure Of The Implicit Value Of Privacy Under Risk
JOURNAL OF CONSUMER MARKETING(2020)
摘要
PurposeMany online transactions and digital services depend on consumers' willingness to take privacy risks, such as when shopping online, joining social networks, using online banking or interacting with e-health platforms. Their decisions depend on not only how much they would suffer if their data were revealed but also how uncomfortable they feel about taking such a risk. Such an aversion to risk is a neglected factor when evaluating the value of privacy. The aim of this paper is to propose an empirical method to measure both privacy risk aversion and privacy worth and how those affect privacy decisions.Design/methodology/approachThe authors let individuals play privacy lotteries and derive a measure of the value of privacy under risk (VPR) and empirically test the validity of this measure in a laboratory experiment with 148 participants. Individuals were asked to make a series of incentivized decisions on whether to incur the risk of revealing private information to other participants.FindingsThe results confirm that the willingness to incur a privacy risk is driven by a complex array of factors, including risk aversion, self-reported value for private information and general attitudes to privacy (derived from surveys). The VPR does not depend on whether there is a preexisting threat to privacy. The authors find qualified support for the existence of an order effect, whereby presenting financial choices prior to privacy ones leads to less concern for privacy.Originality/valueThe authors present a novel method to measure the VPR that takes account of both the value of private information to consumers and their tolerance for privacy risks. The authors explain how this method can be used more generally to elicit attitudes to a wide range of privacy risks involving exposure of various types of private information.
更多查看译文
关键词
Privacy attitudes, Personal information, Disclosure, Risk, Control, Laboratory experiment, C91, D81, O30
AI 理解论文
溯源树
样例
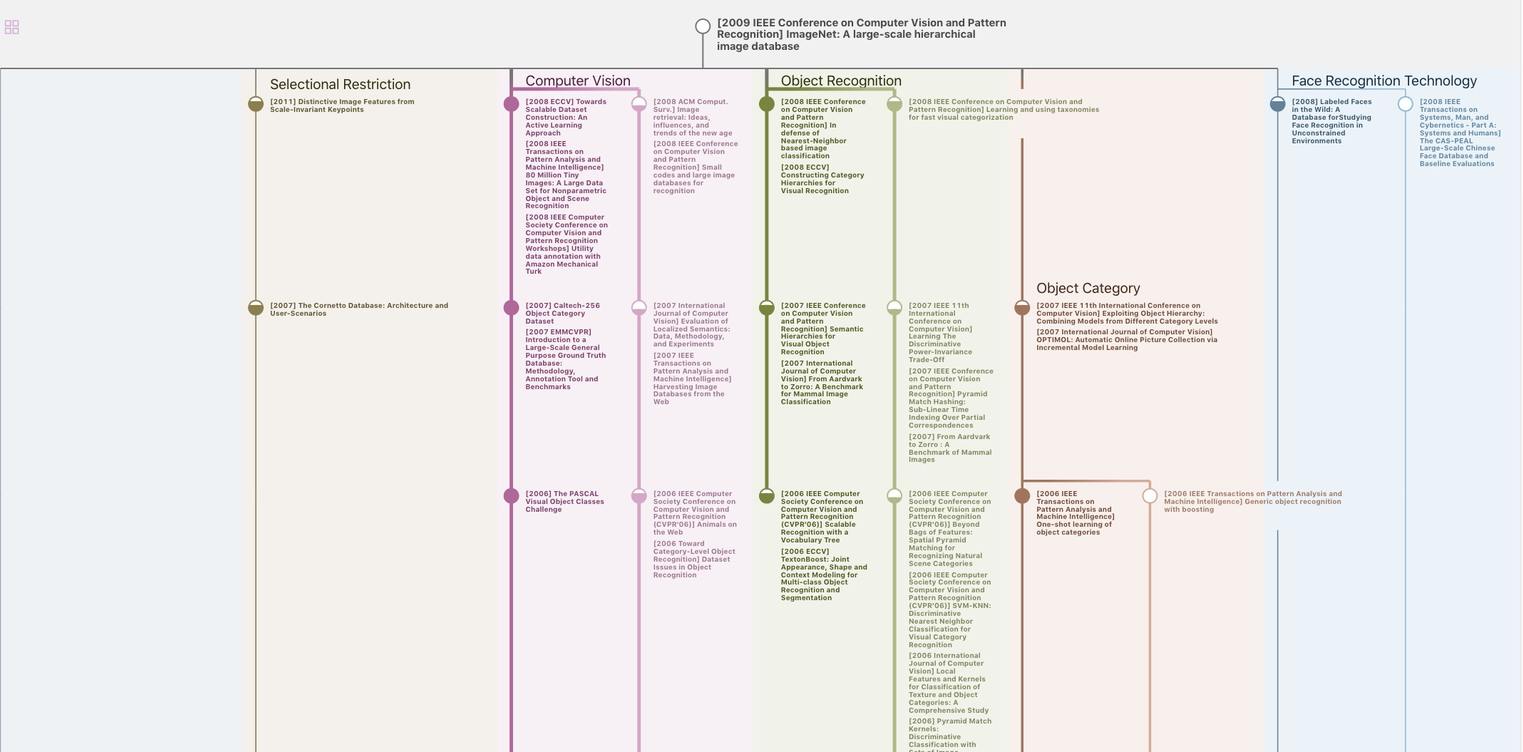
生成溯源树,研究论文发展脉络
Chat Paper
正在生成论文摘要