Data-driven Conflict Detection Enhancement in 3D Airspace with Machine Learning
2020 International Conference on Artificial Intelligence and Data Analytics for Air Transportation (AIDA-AT)(2020)
摘要
Trajectory prediction with Closest Point of Approach (CPA) concept is a fundamental element of aircraft Conflict Detection (CD) problem. Conventional motion-based CPA prediction model generally assumes that aircraft is flying in straight line with constant speed. But due to environment uncertainties and ground speed changes, this conventional method frequently lacks accuracy in the real world with a high rate of false alarms and missed detections. In this paper, we introduce a novel automated data-driven CD framework with Machine Learning (ML) for 3D CPA prediction in a lookahead time of less than 20 minutes. Firstly, a 3D CPA model with cylindrical norm is proposed as the baseline. Then, data preparation with Mode-S observation data in France is explained, including data collection and data processing, to convert raw Mode-S data to the close-to-reality dataset. Furthermore, feature engineering is applied to build up a feature set with 16 features. Finally, four prevailing ML models are used to predict the time, horizontal distance and vertical distance of CPA in 3D airspace. CD is conducted based on the predicted values. The prediction and CD results show that all proposed ML models outperform the baseline model. Especially, GBM and FFNNs could strongly enhance the performance of CD.
更多查看译文
关键词
Air Traffic Management,3D Conflict Detection,Closest Point of Approach,Machine Learning
AI 理解论文
溯源树
样例
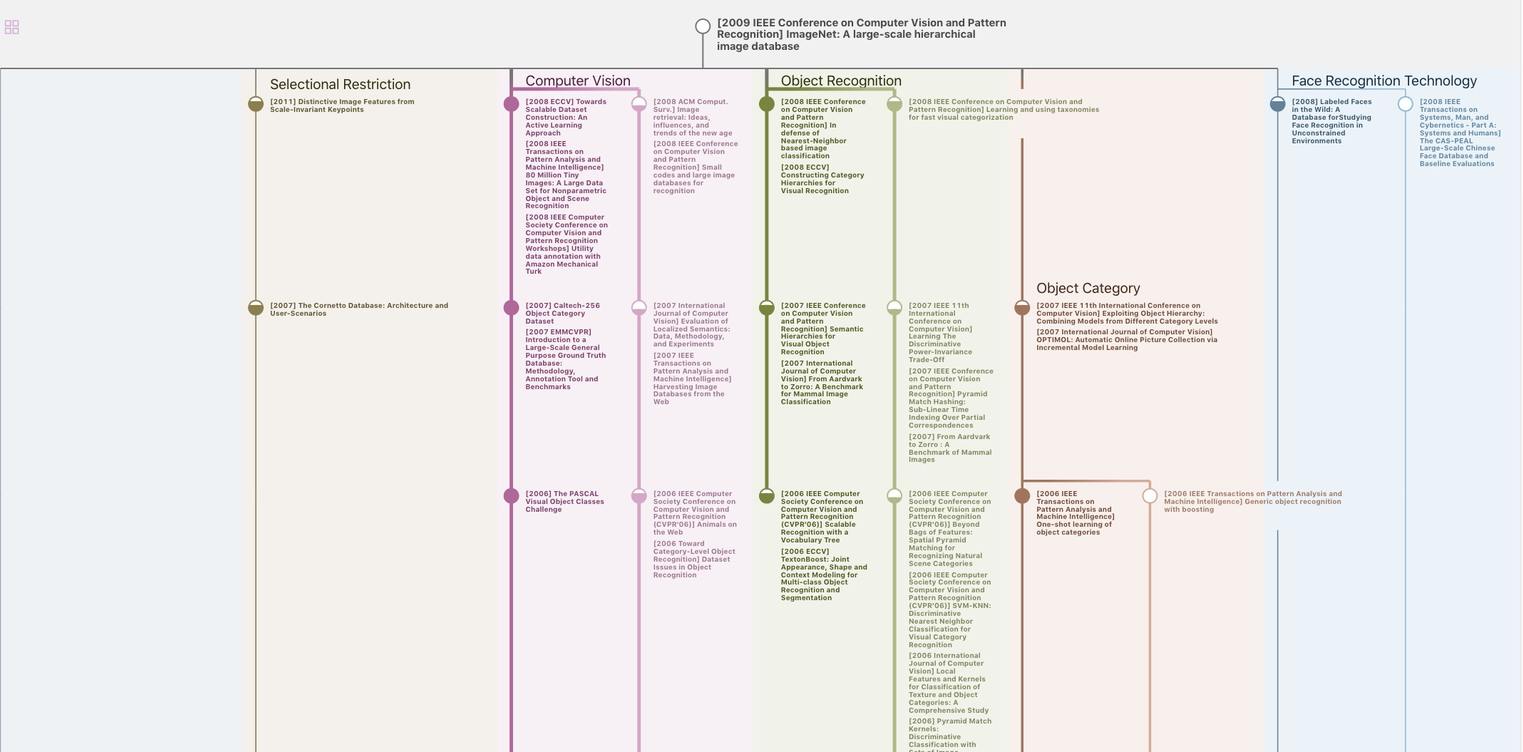
生成溯源树,研究论文发展脉络
Chat Paper
正在生成论文摘要