A mapping model of spectral tilt in normal-to-Lombard speech conversion for intelligibility enhancement
MULTIMEDIA TOOLS AND APPLICATIONS(2020)
摘要
Environmental noise degrades the speech intelligibility when listening to the phone. Although the phone has a clean signal source, it is still difficult for the listener to get information. Intelligibility enhancement (IENH) is a type of perceptual enhancement technique for clean speech rendered in noisy environments. This study focuses on IENH by normal-to-Lombard speech conversion, which is inspired by Lombard reflex. In this conversion process, the key point is to map the spectral tilt from the normal speech (normal style) to the Lombard speech (Lombard style). For mapping the spectral tilt, we propose a mapping model combining linear-prediction-based mapping networks and tilt modification. Compared with previous studies, we use deep neural networks (DNNs) instead of Gaussian-based models for higher dimensional mapping, and inventively add a tilt modification module to reduce the mapping errors of formant magnitudes further. In this paper, we use AVS-M codec and two datasets as the benchmark platform. The valuation shows that our method gets better results than reference methods in both objective and subjective experiments.
更多查看译文
关键词
Intelligibility Enhancement (IENH),Lombard reflex,Spectral tilt,Linear prediction,Deep Neural Networks (DNNs)
AI 理解论文
溯源树
样例
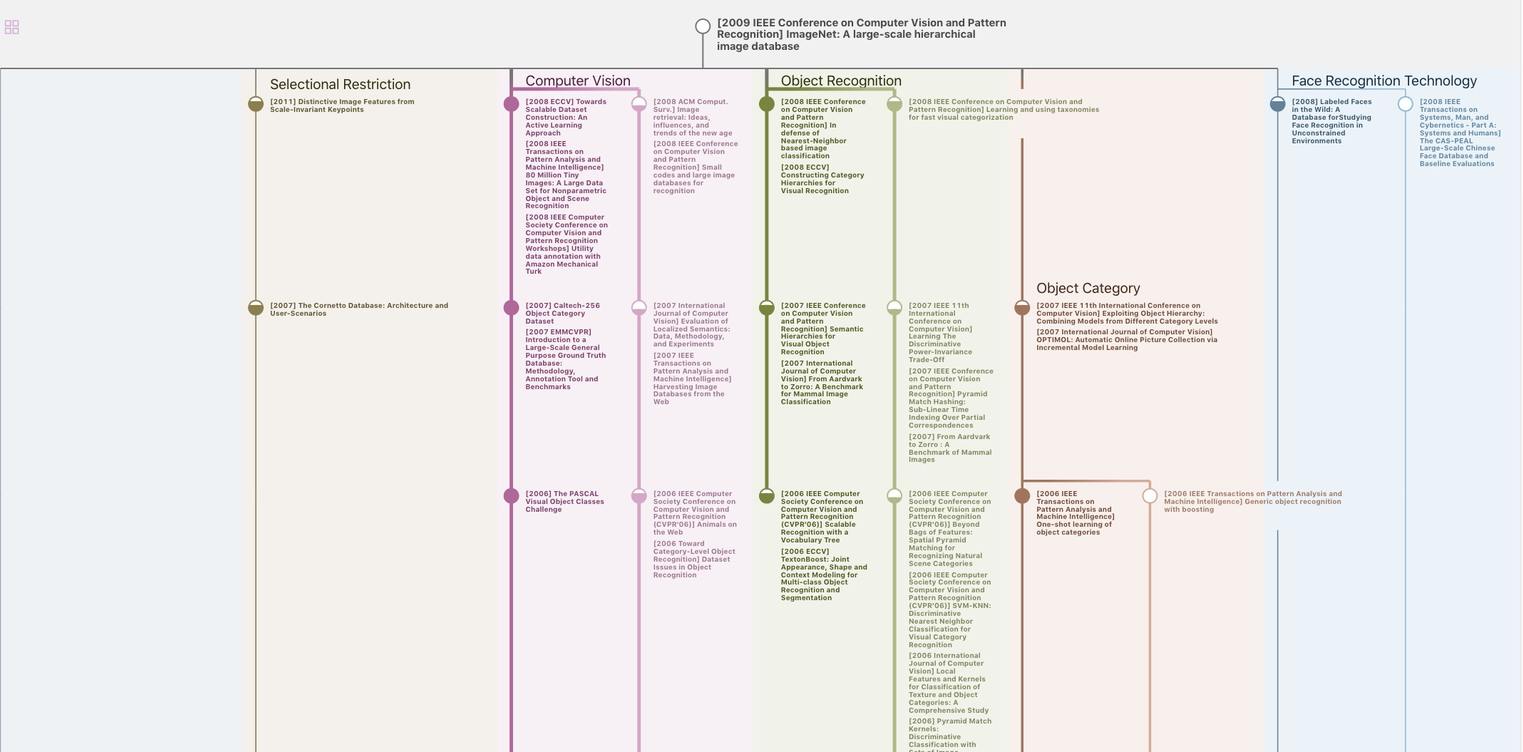
生成溯源树,研究论文发展脉络
Chat Paper
正在生成论文摘要