Deep Cnn For Extraction Of Sidelobes From Sar Imagery In Spectrally Restricted Environment
CONFERENCE RECORD OF THE 2019 FIFTY-THIRD ASILOMAR CONFERENCE ON SIGNALS, SYSTEMS & COMPUTERS(2019)
摘要
This paper explores the critical problem of spectral estimation and recovery via deep convolutional neural networks (CNNs). This problem often arises in ultra-wideband (UWB) synthetic aperture radar (SAR) applications where the wide available bandwidth dictates the final SAR image resolution and quality. Our simple robust solution here also complements well the recently developed stepped-frequency radars built by the U.S. Army Research Laboratory, allowing the system to bypass prohibited frequency bands specified by local frequency management agencies and congested frequency bands that are deemed to contain too much interference. Extensive experimental results confirm the validity as well as the promising prospect of the proposed approach - our CNN-based generative network can still robustly recover SAR images when up to 90% of the operating spectrum is missing.
更多查看译文
关键词
spectral estimation, spectral recovery, ultra-wideband, synthetic aperture radar, convolutional neural networks, deep learning, sparse modeling
AI 理解论文
溯源树
样例
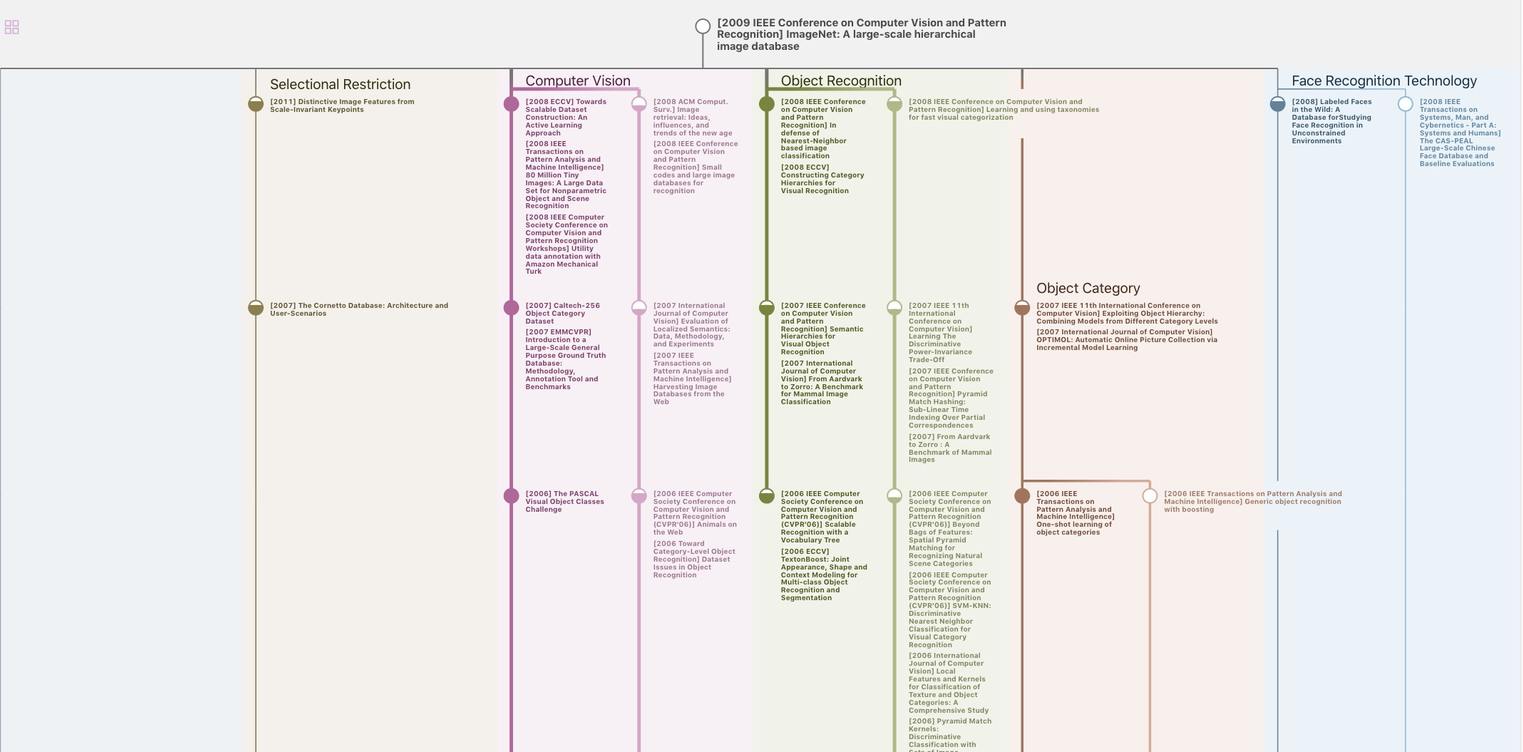
生成溯源树,研究论文发展脉络
Chat Paper
正在生成论文摘要