CO2 Separation from Flue Gas Mixture using [BMIM][BF4]/MOF composites: Linking High-throughput Computational Screening with Experiments
CHEMICAL ENGINEERING JOURNAL(2020)
摘要
In this study, we combined experiments with high-throughput molecular simulation methods to unlock CO2/N-2 separation performances of 1085 different types of ionic liquid (IL)/metal organic framework (MOF) composites. We first validated the accuracy of our proposed computational methodology by synthesizing and characterizing three different IL/MOF composites composed of [BMIM][BF4] (1-n-butyl-3-methylimidazolium tetrafluoroborate) and by comparing their experimental CO2, N-2 adsorption and separation performances with the simulation results. Motivated from the good agreement between experiments and simulations, we performed a high-throughput computational screening of 1085 different types of MOFs and their [BMIM][BF4]-incorporated counterparts to compute adsorption of CO2/N-2 mixture in each material. Adsorbent performance evaluation metrics of [BMIM][BF4]/MOF composites including selectivity, working capacity, adsorbent performance score, and regenerability were calculated and compared with those of pristine MOFs to assess adsorption-based CO2/N-2 separation performance limits of the composite materials. Our results revealed that [BMIM][BF4] incorporation remarkably increases CO2 selectivity, CO2 working capacity, and adsorption performance score of very large numbers of MOFs, resulting in excellent adsorbent candidates for separation of flue gas mixture. Analysis of the structure-performance relations showed that composites having narrow pore sizes, low porosities, and high IL loadings offer high CO2/N-2 selectivities. These results will be useful in guiding and accelerating the design and development of new IL/MOF composites having exceptional CO2 capture performances from flue gas mixtures.
更多查看译文
关键词
Molecular simulations,Ionic liquid (IL),Metal organic framework (MOF),Carbon dioxide (CO2),Gas adsorption
AI 理解论文
溯源树
样例
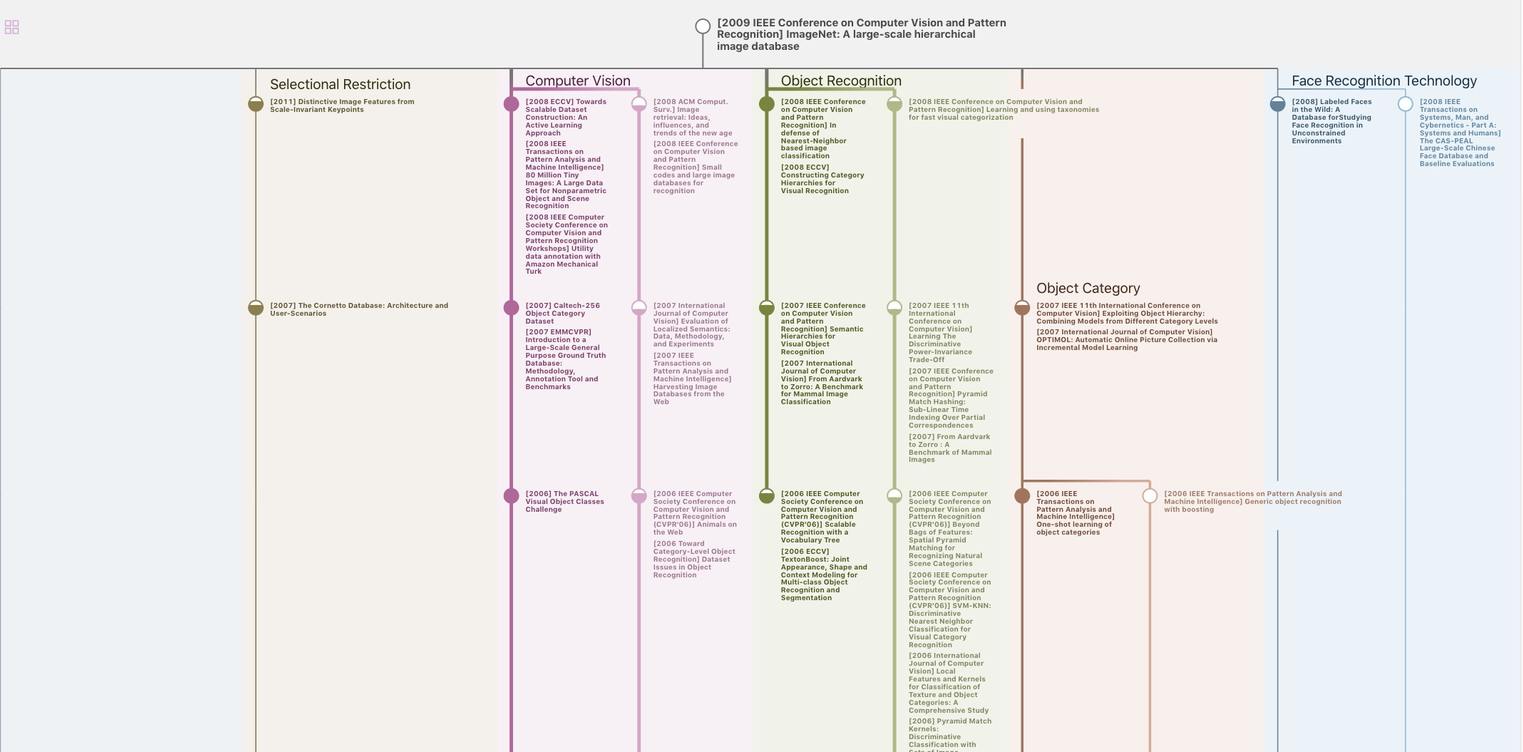
生成溯源树,研究论文发展脉络
Chat Paper
正在生成论文摘要