Sentence-SFV Model: Make Full Use of Word Embedding Actively
2019 International Conference on Intelligent Computing, Automation and Systems (ICICAS)(2019)
摘要
Word Embedding is a method that can learn and capture the grammatical and semantic features contained in the corpus through unsupervised training. Because of this superior characteristic, it is very common to use word vectors as inputs in the downstream tasks. However, little attention has been paid to the active mining of semantic information carried by word vectors. Therefore, we propose a Sentence based Scenario Feature Vector (Sentence-SFV) Model to represent the scenario related grammatical features of sentences quantitively in vector form. With the help of Sentence-SFV model, we extract scenario related features of different corpora and project them into a 3D feature space for visual analysis, by which means we demonstrate the interesting ability of our model like distinguishing grammatical features and explaining grammatical differences between different corpora. We also discuss on the methods to further improve our model, and verify the improvement effect by apply optimized SFV in CNN based sentiment classification experiments.
更多查看译文
关键词
Text classification, sentiment analysis, deep learning, attention mechanism, word embedding
AI 理解论文
溯源树
样例
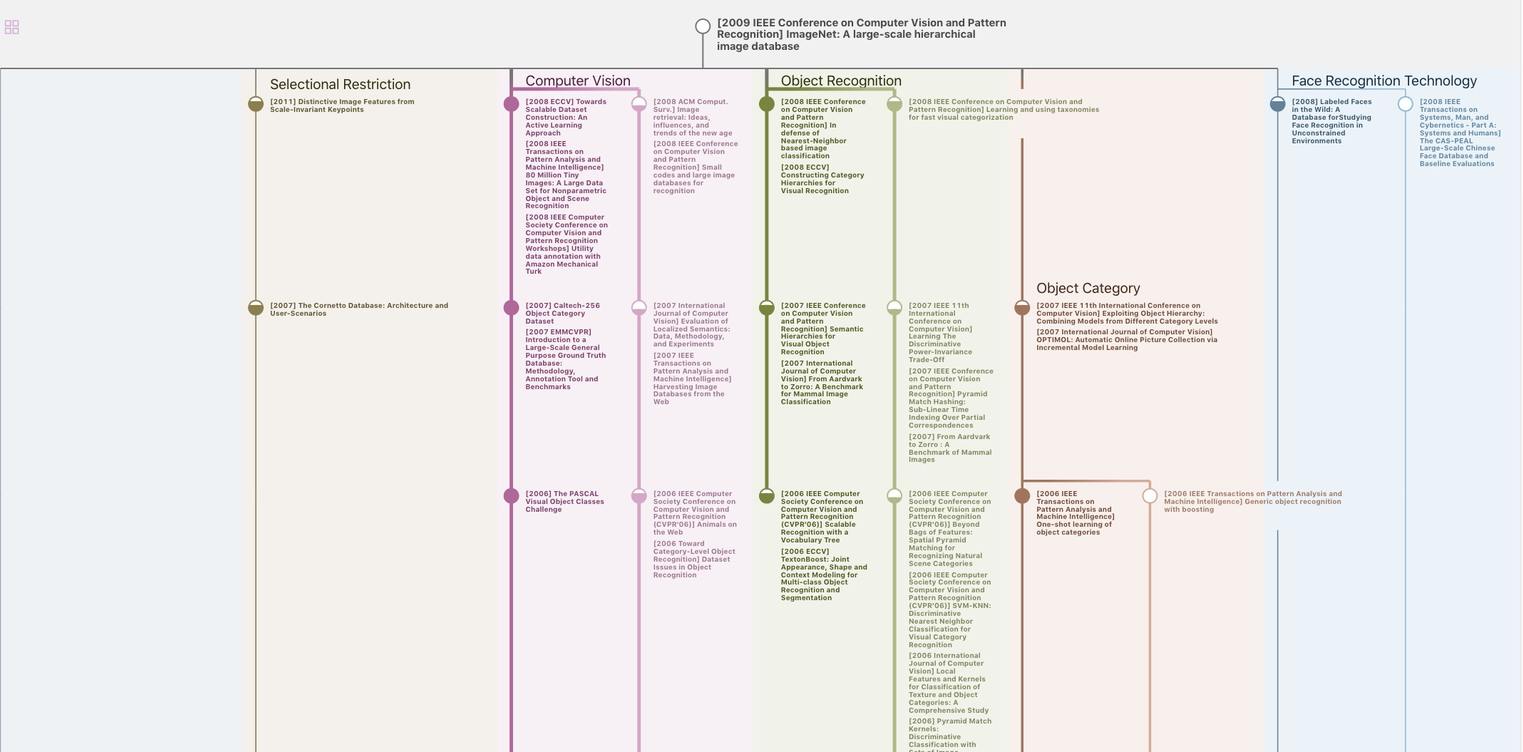
生成溯源树,研究论文发展脉络
Chat Paper
正在生成论文摘要