Survey Of Single Image Super-Resolution Reconstruction
IET IMAGE PROCESSING(2020)
摘要
Image super-resolution reconstruction refers to a technique of recovering a high-resolution (HR) image (or multiple images) from a low-resolution (LR) degraded image (or multiple images). Due to the breakthrough progress in deep learning in other computer vision tasks, people try to introduce deep neural network and solve the problem of image super-resolution reconstruction by constructing a deep-level network for end-to-end training. The currently used deep learning models can divide the SISR model into four types: interpolation-based preprocessing-based model, original image processing based model, hierarchical feature-based model, and high-frequency detail-based model, or shared the network model. The current challenges for super-resolution reconstruction are mainly reflected in the actual application process, such as encountering an unknown scaling factor, losing paired LR-HR images, and so on.
更多查看译文
关键词
feature extraction, learning (artificial intelligence), remote sensing, image resolution, computer vision, interpolation, neural nets, image processing, image reconstruction, generative adversarial network, super-resolution images, popular CNN-based deep learning method, GAN-based deep learning method, LR-HR images, multiscale super-resolution reconstruction, single image super-resolution reconstruction, or multiple images, LR images, hyperspectral imaging, medical imaging, deep neural network, deep-level network, image input information, original image processing-based model, hierarchical feature-based model, network model
AI 理解论文
溯源树
样例
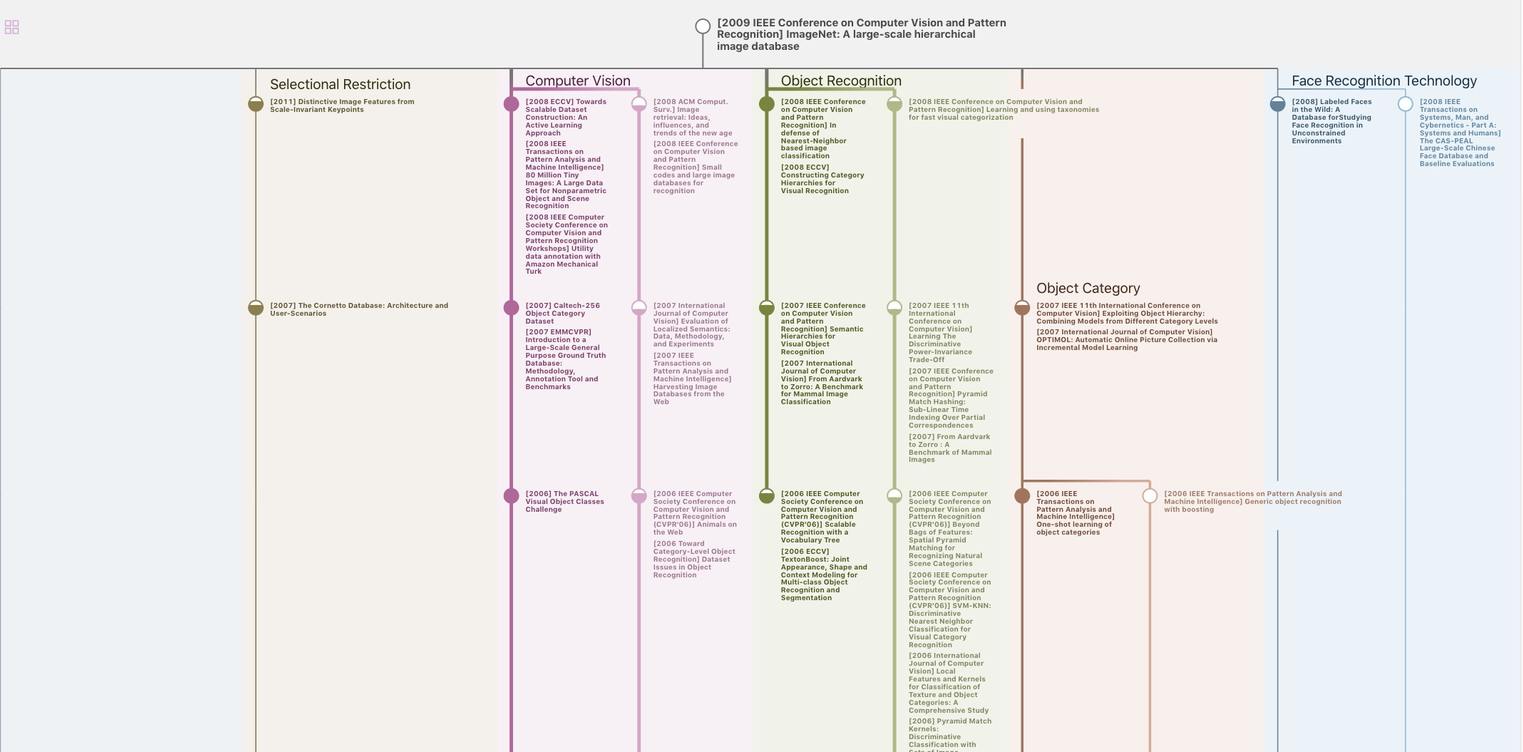
生成溯源树,研究论文发展脉络
Chat Paper
正在生成论文摘要