Regionally Optimized Background Earthquake Rates from ETAS (ROBERE) for Probabilistic Seismic Hazard Assessment
BULLETIN OF THE SEISMOLOGICAL SOCIETY OF AMERICA(2020)
摘要
We use an epidemic-type aftershock sequence (ETAS) based approach to develop a regionally optimized background earthquake rates from ETAS (ROBERE) method for probabilistic seismic hazard assessment. ROBERE fits parameters to the full seismicity catalog for a region with maximum-likelihood estimation, including uncertainty. It then averages the earthquake rates over a suite of catalogs from which foreshocks and aftershocks have been removed using stochastic declustering while maintaining the same Gaussian smoothing currently used for the U.S. Geological Survey National Seismic Hazard Model (NSHM). The NSHM currently determines these rates by smoothing a single catalog from which foreshocks and aftershocks have been removed using the method of Gardner and Knopoff (1974; hereafter, GK74). The parameters used in GK74 were determined from subjectively identified aftershock sequences, unlike ROBERE, in which both background rate and aftershock triggering parameters are objectively fitted. A major difference between the impacts of the two methods is GK74 significantly reduces the b-value, a critical value for seismic hazard analysis, whereas ROBERE maintains the original b-value from the full catalog. We apply these methods to the induced seismicity in Oklahoma and Kansas and tectonic activity in the San Francisco Bay Region. Using GK74 gives lower overall earthquake rates but estimates higher hazard due to the reduction in the b-value. ROBERE provides higher earthquake rates, at the magnitude of completeness, but lower hazard because it does not alter the b-value. We test two other declustering methods that produce results closer to ROBERE but do not use objectively fit parameters, include uncertainty, and may not work as well in other areas. We suggest adopting ROBERE for the NSHM so that our hazard estimates are based on an objective analysis, including uncertainty, and do not depend strongly on potentially biased b-values, which was never the goal of the existing methodology.
更多查看译文
AI 理解论文
溯源树
样例
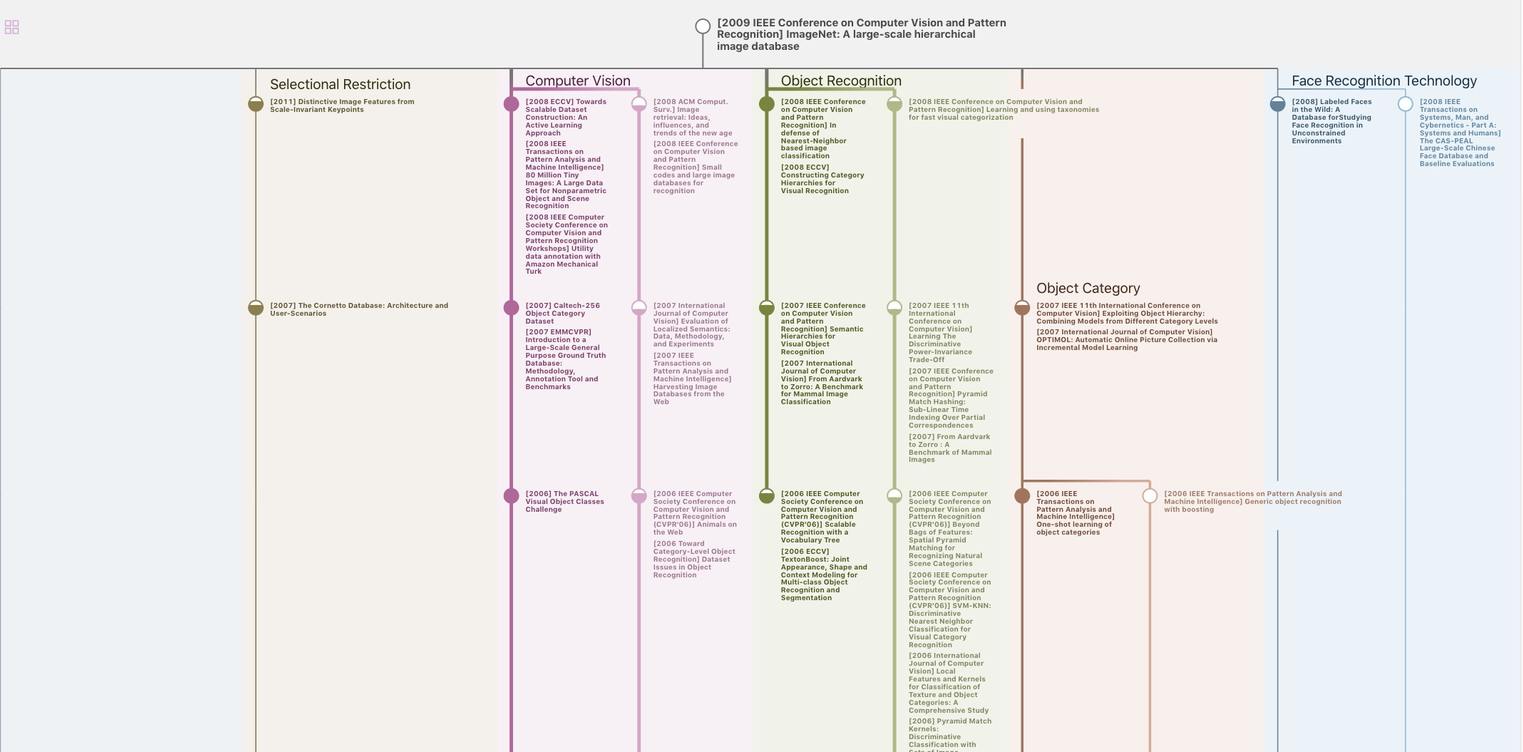
生成溯源树,研究论文发展脉络
Chat Paper
正在生成论文摘要