Synchronous n-Step Method for Independent Q-Learning in Multi-Agent Deep Reinforcement Learning.
SmartWorld/SCALCOM/UIC/ATC/CBDCom/IOP/SCI(2019)
摘要
Experience replay memory (ERM) is an effective tool for sampling decorrelated data to train the policy network and improving data utilization in off-policy deep reinforcement learning. However, ERM introduces fluctuations in the training processes of independent Q-learning (IQL) in multi-agent deep reinforcement learning (MA-DRL) because its stored experiences may become obsolete as the agents in IQL update their policies in parallel. Reducing the influence of obsolete experiences while extensively exploring the potential of data in training poses a huge challenge in IQL. Therefore, we propose the synchronous n-step method that totally eliminates obsolete experiences but suffers from the low data utilization problem. Then, we propose the ERM-helped synchronous n-step method that makes a balance between reducing the influence of obsolete experience and enhancing data utilization. We apply these methods to lenient deep reinforcement learning and propose the LSnDQN and LESnDQN algorithms, which we subsequently test in some extended variations of the coordinated multi-agent object transportation problem. Results show that LSnDQN has a great advantage on training with less iterations but no advantage on computation time over LDQN, while LESnDQN has a great advantage on computation time over both LDQN and LSnDQN.
更多查看译文
关键词
Multi-agent learning,Independent Q-learning,Synchronous method,Experience replay memory
AI 理解论文
溯源树
样例
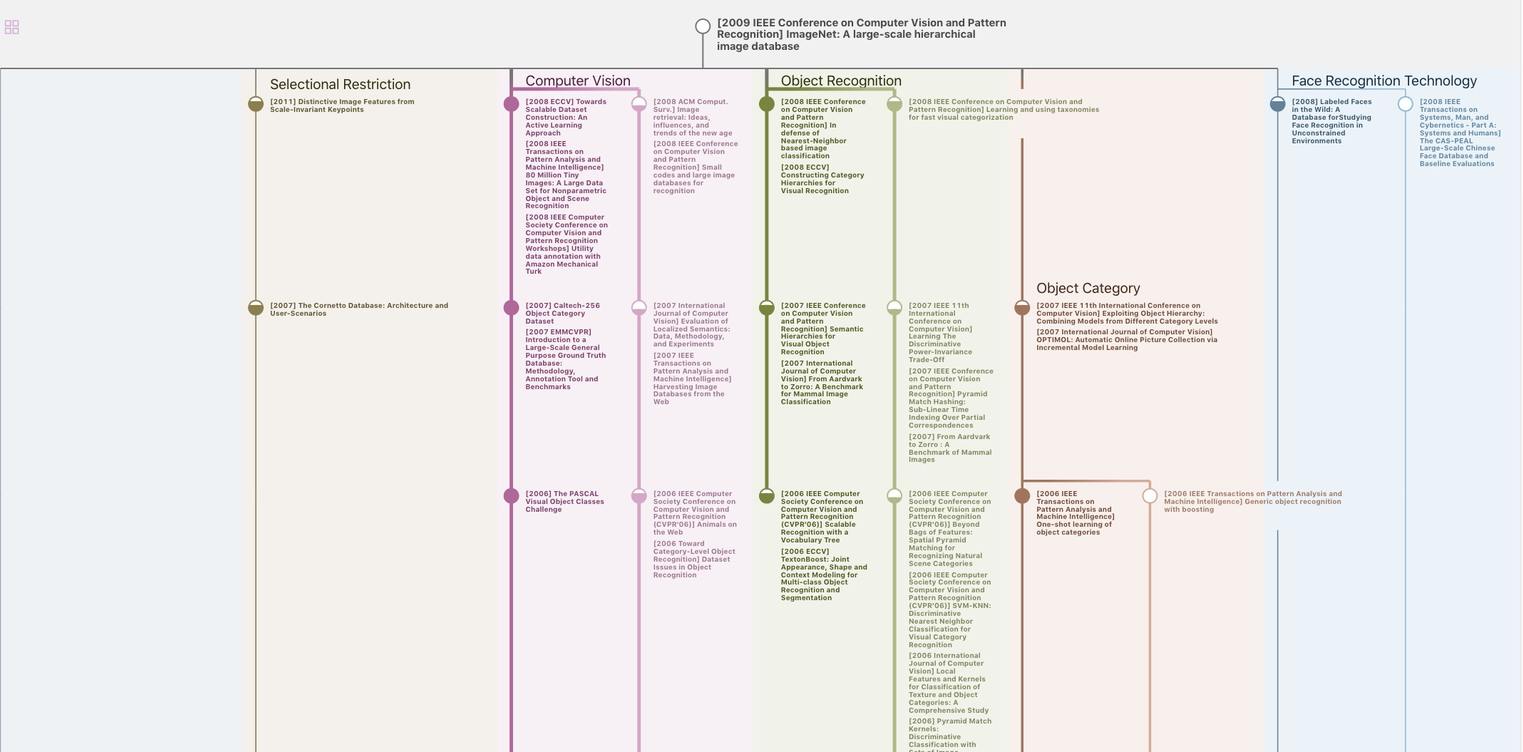
生成溯源树,研究论文发展脉络
Chat Paper
正在生成论文摘要