Language-agnostic Multilingual Modeling
2020 IEEE INTERNATIONAL CONFERENCE ON ACOUSTICS, SPEECH, AND SIGNAL PROCESSING(2020)
摘要
Multilingual Automated Speech Recognition (ASR) systems allow for the joint training of data-rich and data-scarce languages in a single model. This enables data and parameter sharing across languages, which is especially beneficial for the data-scarce languages. However, most state-of-the-art multilingual models require the encoding of language information and therefore are not as flexible or scalable when expanding to newer languages. Language-independent multilingual models help to address this issue, and are also better suited for multicultural societies where several languages are frequently used together (but often rendered with different writing systems). In this paper, we propose a new approach to building a language-agnostic multilingual ASR system which transforms all languages to one writing system through a many-to-one transliteration transducer. Thus, similar sounding acoustics are mapped to a single, canonical target sequence of graphemes, effectively separating the modeling and rendering problems. We show with four Indic languages, namely, Hindi, Bengali, Tamil and Kannada, that the language-agnostic multilingual model achieves up to 10% relative reduction in Word Error Rate (WER) over a language-dependent multilingual model.
更多查看译文
关键词
speech recognition, language-independent, multilingual, transliteration, RNN-T
AI 理解论文
溯源树
样例
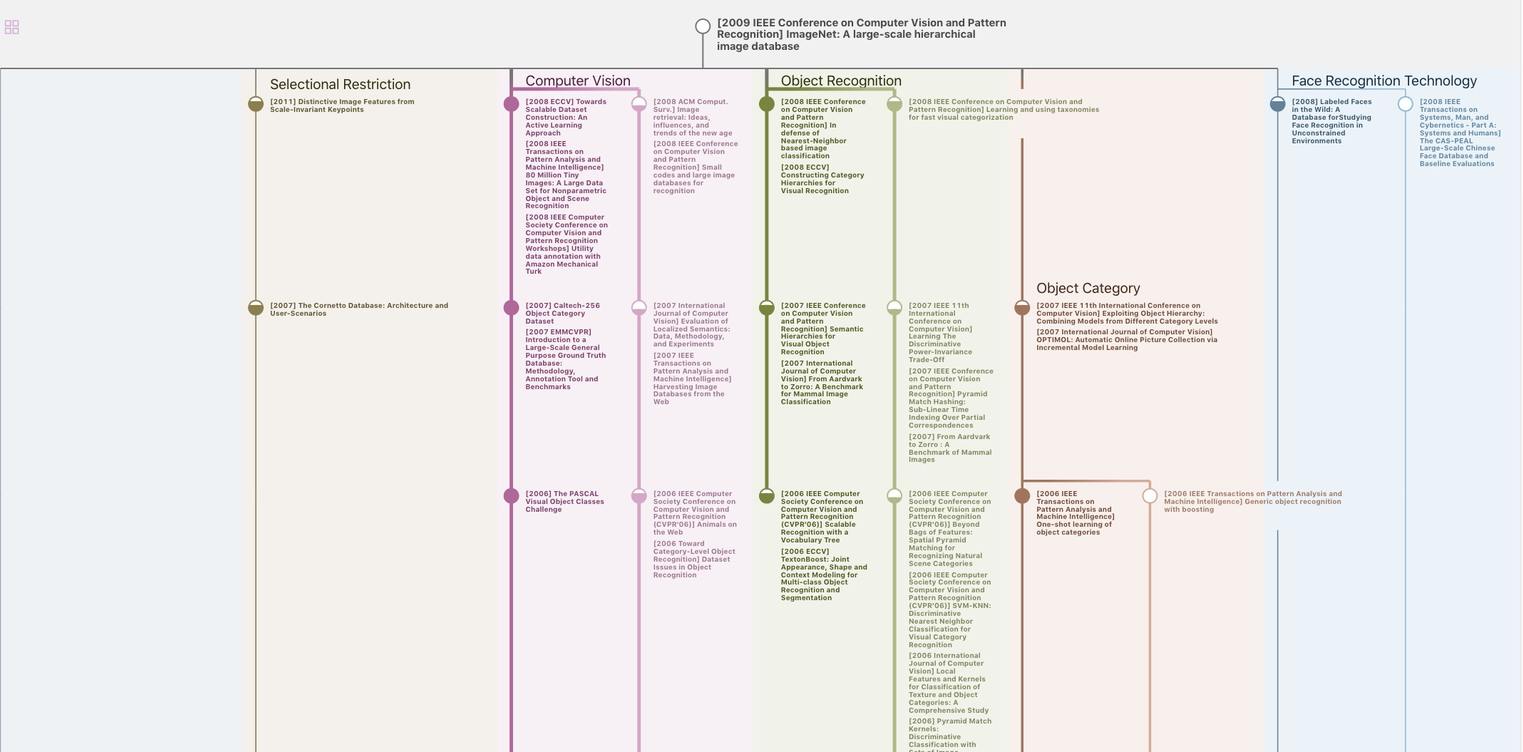
生成溯源树,研究论文发展脉络
Chat Paper
正在生成论文摘要