A Data and Compute Efficient Design for Limited-Resources Deep Learning
arxiv(2020)
摘要
Thanks to their improved data efficiency, equivariant neural networks have gained increased interest in the deep learning community. They have been successfully applied in the medical domain where symmetries in the data can be effectively exploited to build more accurate and robust models. To be able to reach a much larger body of patients, mobile, on-device implementations of deep learning solutions have been developed for medical applications. However, equivariant models are commonly implemented using large and computationally expensive architectures, not suitable to run on mobile devices. In this work, we design and test an equivariant version of MobileNetV2 and further optimize it with model quantization to enable more efficient inference. We achieve close-to state of the art performance on the Patch Camelyon (PCam) medical dataset while being more computationally efficient.
更多查看译文
关键词
deep learning,compute efficient design,limited-resources
AI 理解论文
溯源树
样例
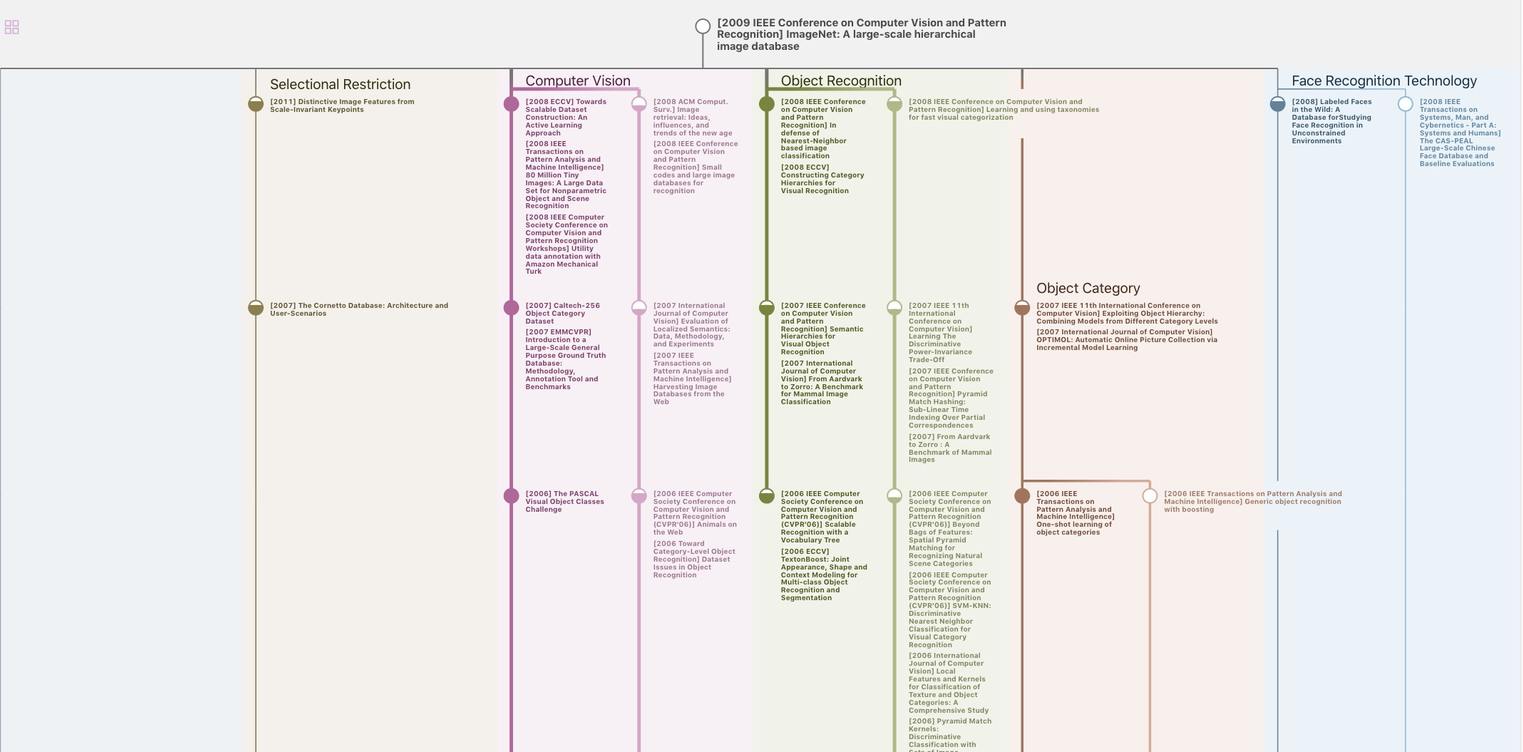
生成溯源树,研究论文发展脉络
Chat Paper
正在生成论文摘要