Convex Nonparanormal Regression
IEEE SIGNAL PROCESSING LETTERS(2021)
摘要
Quantifying uncertainty in predictions or, more generally, estimating the posterior conditional distribution, is a core challenge in machine learning and statistics. We introduce Convex Nonparanormal Regression (CNR), a conditional nonparanormal approach for coping with this task. CNR involves a convex optimization of a posterior defined via a rich dictionary of pre-defined non linear transformations on Gaussians. It can fit an arbitrary conditional distribution, including multimodal and non-symmetric posteriors. For the special but powerful case of a piecewise linear dictionary, we provide a closed form of the posterior mean which can be used for point-wise predictions. Finally, we demonstrate the advantages of CNR over classical competitors using synthetic and real world data.
更多查看译文
关键词
Dictionaries, Optimization, Maximum likelihood estimation, Convex functions, Training, Linear regression, Gaussian distribution, Linear regression, nonparanormal distribution, convex optimization
AI 理解论文
溯源树
样例
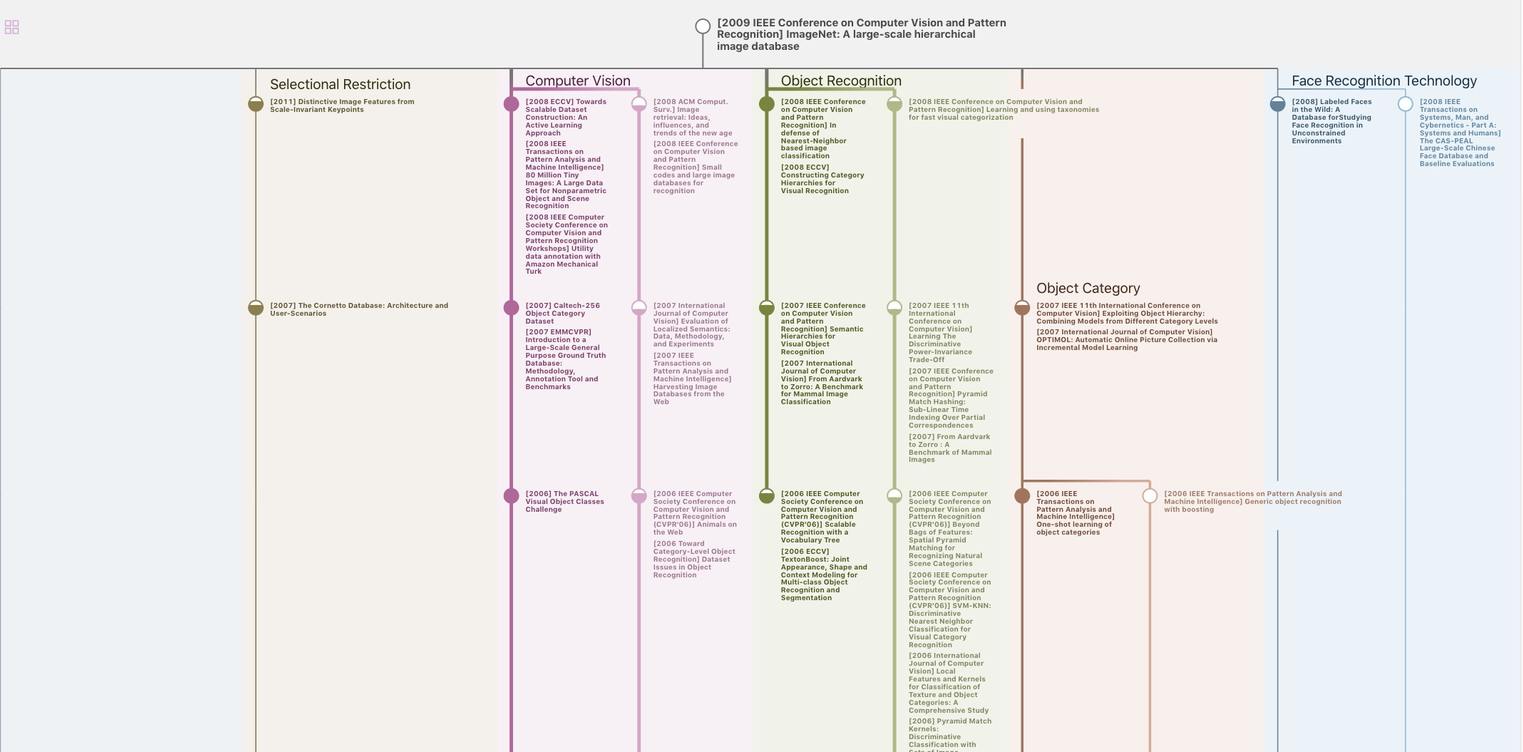
生成溯源树,研究论文发展脉络
Chat Paper
正在生成论文摘要