M-LVC: Multiple Frames Prediction for Learned Video Compression
2020 IEEE/CVF CONFERENCE ON COMPUTER VISION AND PATTERN RECOGNITION (CVPR)(2020)
摘要
We propose an end-to-end learned video compression scheme for low-latency scenarios. Previous methods are limited in using the previous one frame as reference. Our method introduces the usage of the previous multiple frames as references. In our scheme, the motion vector (MV) field is calculated between the current frame and the previous one. With multiple reference frames and associated multiple MV fields, our designed network can generate more accurate prediction of the current frame, yielding less residual. Multiple reference frames also help generate MV prediction, which reduces the coding cost of MV field. We use two deep auto-encoders to compress the residual and the MV, respectively. To compensate for the compression error of the auto-encoders, we further design a MV refinement network and a residual refinement network, taking use of the multiple reference frames as well. All the modules in our scheme are jointly optimized through a single rate-distortion loss function. We use a step-by-step training strategy to optimize the entire scheme. Experimental results show that the proposed method outperforms the existing learned video compression methods for low-latency mode. Our method also performs better than H.265 in both PSNR and MS-SSIM. Our code and models are publicly available.
更多查看译文
关键词
end-to-end learned video compression scheme,motion vector field,multiple reference frames,multiple MV fields,MV prediction,MV refinement network,M-LVC,multiple frame prediction,deep auto-encoders,residual refinement network,single rate-distortion loss function,step-by-step training strategy,PSNR,MS-SSIM
AI 理解论文
溯源树
样例
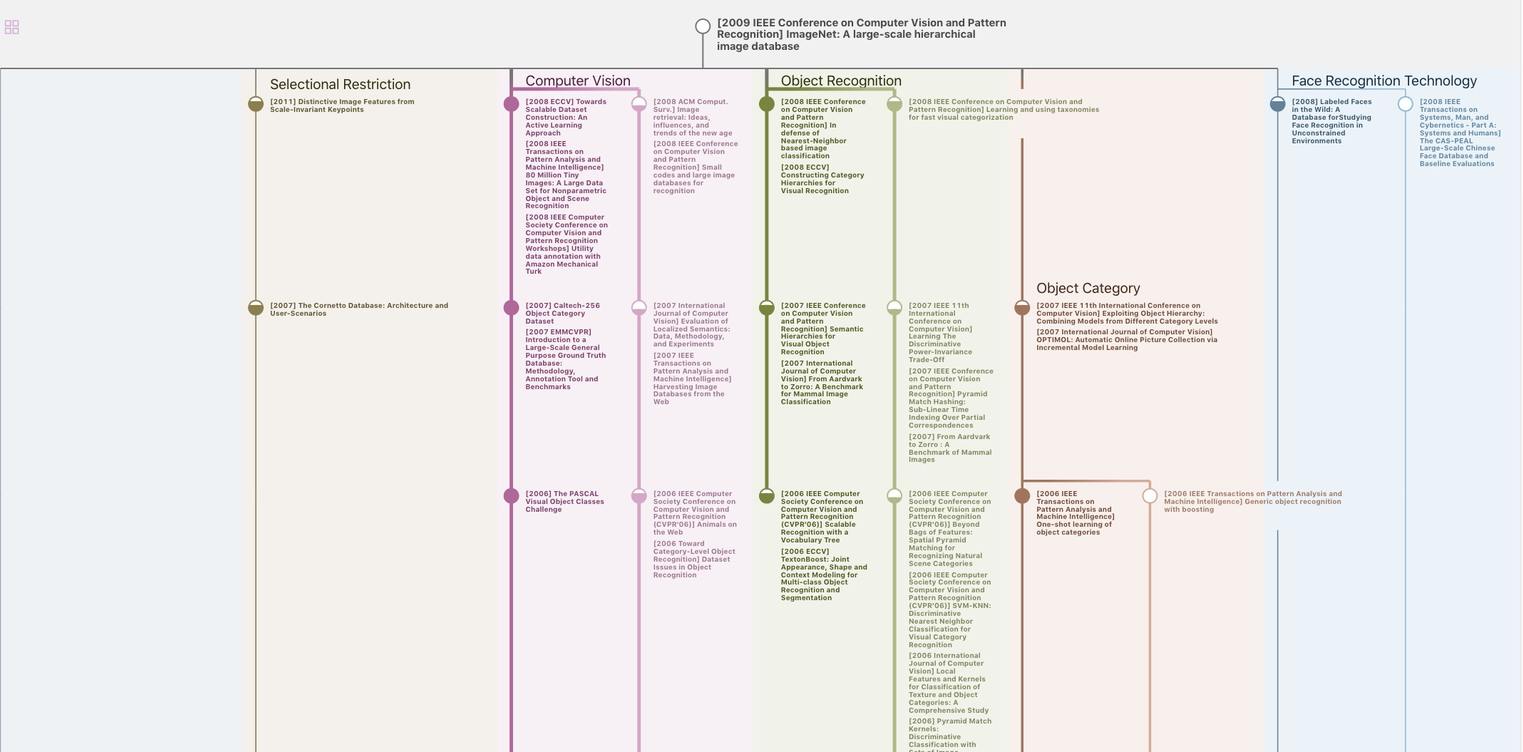
生成溯源树,研究论文发展脉络
Chat Paper
正在生成论文摘要