Physics-Based Approach for a Neural Networks Enabled Design of All-Dielectric Metasurfaces
arxiv(2020)
摘要
Machine learning methods have found novel application areas in various disciplines as they offer low computational cost solutions to complex problems. Recently, metasurface design has joined these applications, and neural networks enabled significant improvements within a short period of time. However, there are still outstanding challenges that need to be overcome. Here, we propose a data preprocessing approach based on the governing laws of the physical problem to eliminate dimensional mismatch between a high dimensional optical response and a low dimensional feature space of metasurfaces. We train forward and inverse models to predict optical responses of cylindrical meta-atoms and to retrieve their geometric parameters for a desired optical response, respectively. Our approach provides accurate prediction capability even outside the training spectral range. Finally, using our inverse model, we design and demonstrate a focusing metalens as a proof-of-concept application, thus, validating the capability of our proposed approach. We believe our method will pave the way toward practical learning-based models to solve more complicated photonic design problems.
更多查看译文
关键词
deep learning,neural networks,metasurfaces,inverse design,all-dielectric
AI 理解论文
溯源树
样例
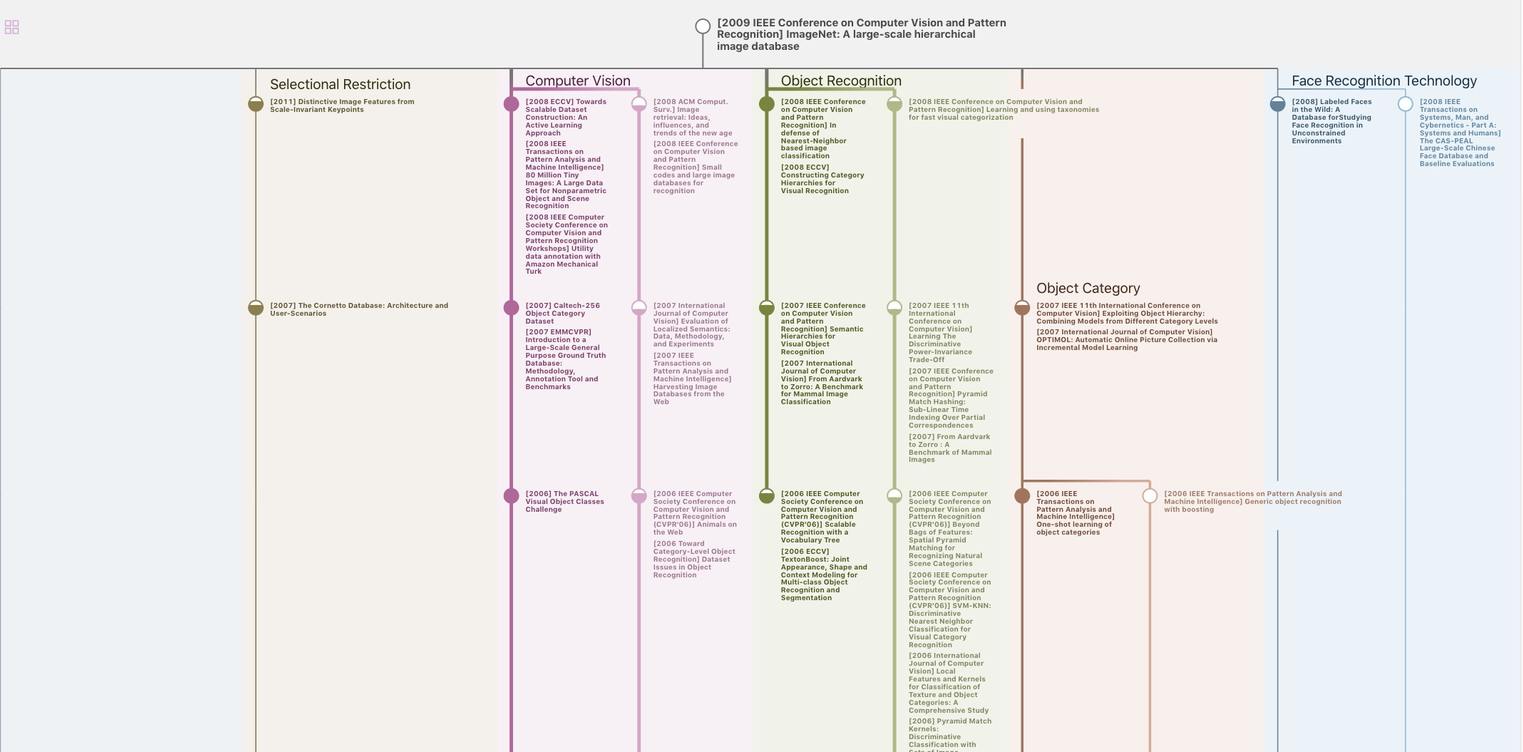
生成溯源树,研究论文发展脉络
Chat Paper
正在生成论文摘要