Multi-Weight Partial Domain Adaptation.
BMVC(2019)
摘要
Domain adaptation (DA) plays an important role in transfer learning. However, when target label space is a subset of source label space, standard DA cannot tackle this issue. Partial domain adaptation focuses on how to transfer knowledge from massive labelled dataset to unlabelled miniature one, which attracts extensive research interest. In this paper, we propose a Multi-Weight Partial Domain Adaptation (MWPDA) to solve the problem. We divide the source domain into two parts: shared classes and outlier classes. MWPDA aims to reduce negative transfer caused by outlier classes when transferring knowledge between domains. Based on OTSU-Algorithm, hard shared-class labels are obtained to decrease weights of outlier classes and increase ones of shared classes. A novel shared-sample classifier is trained for shared-sample weights to distinguish outlier samples. Shared-class weights and shared-sample weights are acted on source classifier and domain discriminator to jointly distinguish outlier classes and samples. This kind of multiweight mechanism can avoid misalignment to outlier classes and promote classification accuracy. Furthermore, our universal network framework is utilized for both partial domain adaptation and standard domain adaptation issues. Extensive experiments on three benchmark domain adaptation datasets illustrate our method achieves state-of-the-art results.
更多查看译文
关键词
adaptation,domain,multi-weight
AI 理解论文
溯源树
样例
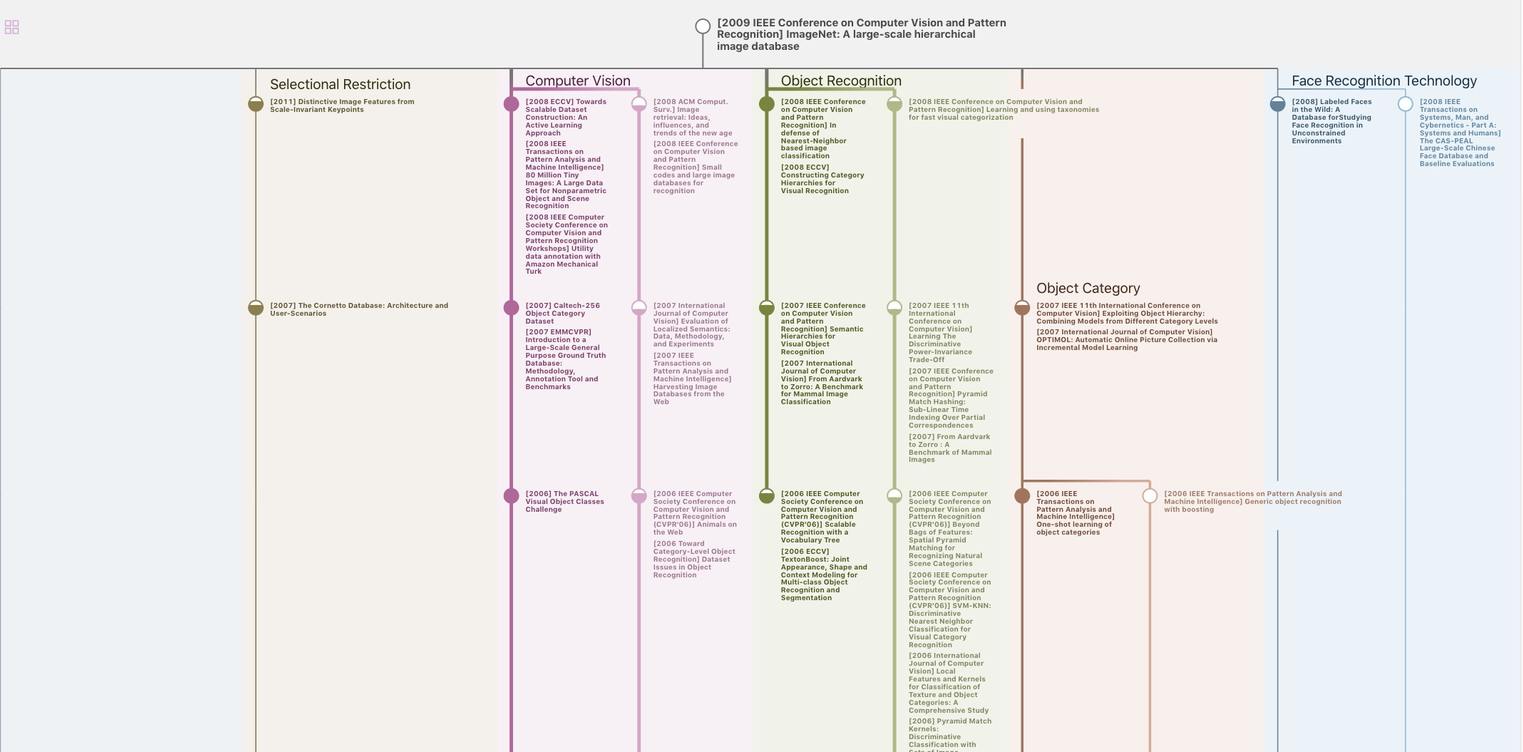
生成溯源树,研究论文发展脉络
Chat Paper
正在生成论文摘要