Modelling Performance During Repetitive Precision Tasks Using Wearable Sensors: a Data-Driven Approach.
Ergonomics(2020)
摘要
In modern manufacturing systems, especially assembly lines, human input is a critical resource to provide dexterity and flexibility. However, the repetitive precision tasks common in assembly lines can have adverse effects on workers and overall system performance. We present a data-driven approach to evaluating task performance using wearable sensor data (kinematics, electromyography and heart rate). Eighteen participants (gender-balanced) completed repeated cycles of maze tracking and assembly/disassembly. Various combinations of input data types and classification algorithms were used to model task performance. The use of the linear discriminant analysis (LDA) algorithm and kinematic data provided the most promising classification performance. The highest model accuracy was found using the LDA algorithm and all data types, with respective levels of 62.4, 88.6, 85.8 and 94.1% for predicting maze errors, maze speed, assembly/disassembly errors and assembly/disassembly speed. The presented approach provides the possibility for real-time, on-line and comprehensive monitoring of system performance in assembly-lines or similar industries. Practitioner summary: This paper proposed models the repetitive precision task performance using data collected from wearable sensors. The use of the LDA algorithm and kinematic data provided the most promising classification performance. The presented approach provides the possibility for real-time, on-line and comprehensive monitoring of system performance in assembly lines or similar industries. Abbreviations: AD: anterior deltoid; BB: biceps brachii; ECR: extensor carpi radialis; ECU: extensor carpi ulnaris; FCR: flexor carpi radialis; FCU: flexor carpi ulnaris; FN: false negatives; FP: false positives; HR: heart rate; HRR: heart rate reserve; IMUs: inertial measurement units; kNN: k-nearest neighbors; LDA: linear discriminant analysis; MD: medial deltoid; MF: median power frequency; MNF: mean power frequency; MVIC: maximum voluntary isometric contraction; nRMS: normalized root-mean-square amplitudes; PD: posterior deltoid; RandFor: random forests; RHR: resting heart rate; RMS: root-mean-square amplitudes; sEMG: surface electromyographic; SVM: support vector machines; TB: triceps brachii medial; TN: true negatives; TP: true positives; t-SNE: t-distributed Stochastic Neighbor Embedding; UT: upper trapezius.
更多查看译文
关键词
Performance modelling,wearable technologies,repetitive precision task,classification
AI 理解论文
溯源树
样例
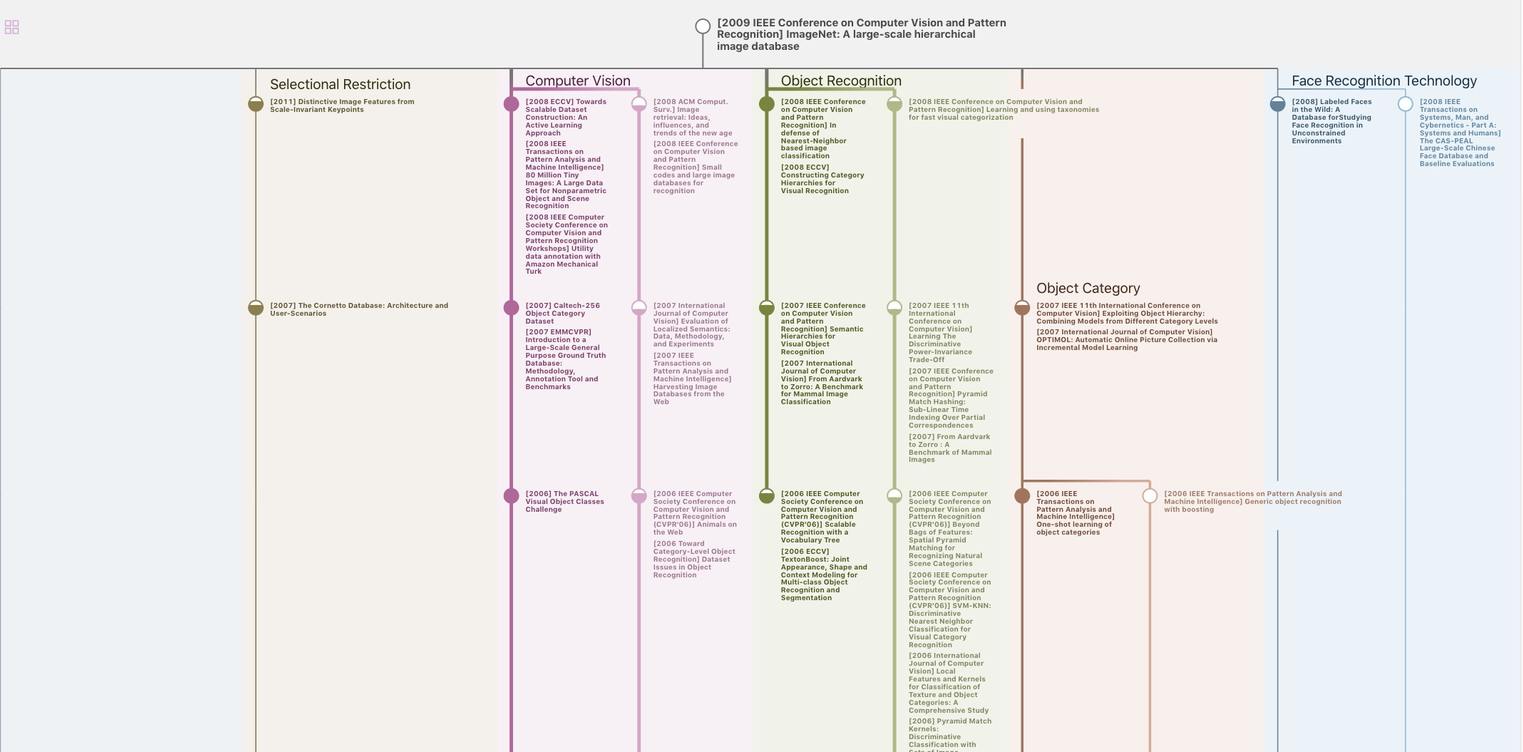
生成溯源树,研究论文发展脉络
Chat Paper
正在生成论文摘要