PalmBoard: Leveraging Implicit Touch Pressure in Statistical Decoding for Indirect Text Entry
CHI '20: CHI Conference on Human Factors in Computing Systems Honolulu HI USA April, 2020(2020)
摘要
We investigated how to incorporate implicit touch pressure, finger pressure applied to a touch surface during typing, to improve text entry performance via statistical decoding. We focused on one-handed touch-typing on indirect interface as an example scenario. We first collected typing data on a pressure-sensitive touchpad, and analyzed users' typing behavior such as touch point distribution, key-to-finger mappings, and pressure images. Our investigation revealed distinct pressure patterns for different keys. Based on the findings, we performed a series of simulations to iteratively optimize the statistical decoding algorithm. Our investigation led to a Markov-Bayesian decoder incorporating pressure image data into decoding. It improved the top-1 accuracy from 53% to 74% over a naive Bayesian decoder. We then implemented PalmBoard, a text entry method that implemented the Markov-Bayesian decoder and effectively supported one-handed touch-typing on indirect interfaces. A user study showed participants achieved an average speed of 32.8 WPM with 0.6% error rate. Expert typists could achieve 40.2 WPM with 30 minutes of practice. Overall, our investigation showed that incorporating implicit touch pressure is effective in improving text entry decoding.
更多查看译文
关键词
Touch-typing, text entry, input prediction, touch pressure
AI 理解论文
溯源树
样例
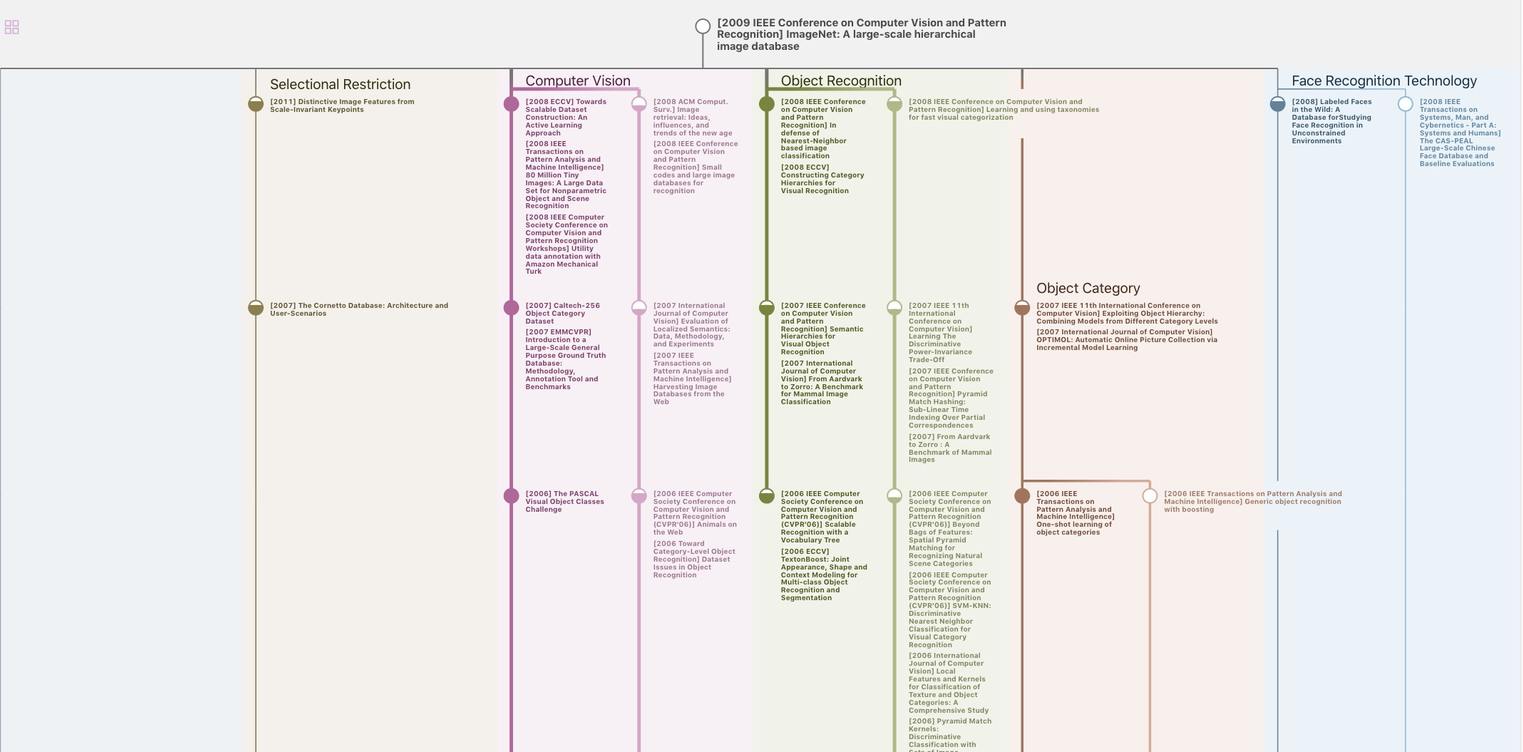
生成溯源树,研究论文发展脉络
Chat Paper
正在生成论文摘要