Disentangled And Controllable Face Image Generation Via 3d Imitative-Contrastive Learning
2020 IEEE/CVF CONFERENCE ON COMPUTER VISION AND PATTERN RECOGNITION (CVPR)(2020)
摘要
We propose an approach for face image generation of virtual people with disentangled, precisely-controllable latent representations for identity of non-existing people, expression, pose, and illumination. We embed 3D priors into adversarial learning and train the network to imitate the image formation of an analytic 3D face deformation and rendering process. To deal with the generation freedom induced by the domain gap between real and rendered faces, we further introduce contrastive learning to promote disentanglement by comparing pairs of generated images. Experiments show that through our imitative-contrastive learning, the factor variations are very well disentangled and the properties of a generated face can be precisely controlled. We also analyze the learned latent space and present several meaningful properties supporting factor disentanglement. Our method can also be used to embed real images into the disentangled latent space. We hope our method could provide new understandings of the relationship between physical properties and deep image synthesis.
更多查看译文
关键词
disentangled controllable face image generation,imitative-contrastive learning,virtual people,precisely-controllable latent representations,adversarial learning,image formation,rendering process,generation freedom,rendered faces,generated face,learned latent space,factor disentanglement,embed real images,disentangled latent space,deep image synthesis
AI 理解论文
溯源树
样例
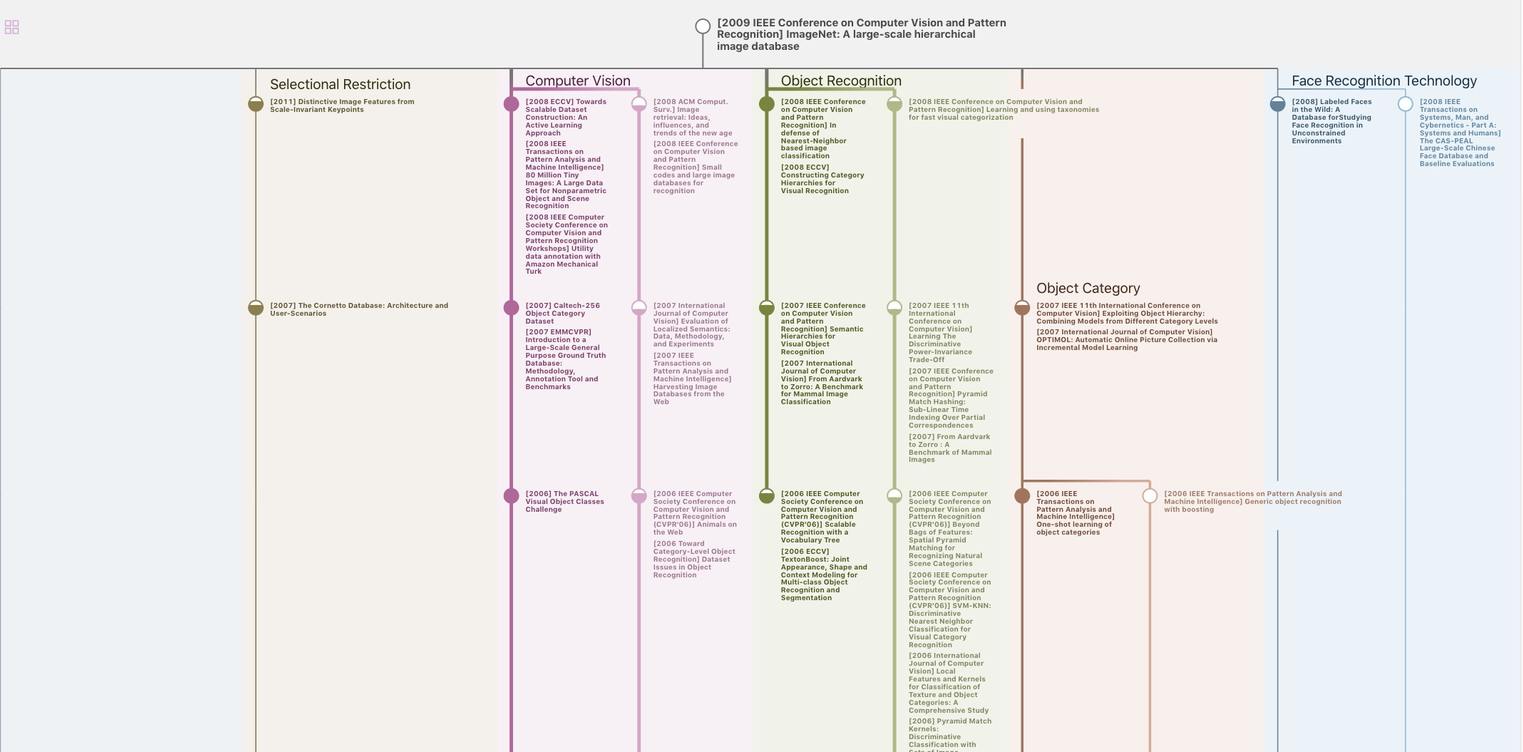
生成溯源树,研究论文发展脉络
Chat Paper
正在生成论文摘要