A Systematic Search Over Deep Convolutional Neural Network Architectures For Screening Chest Radiographs
42ND ANNUAL INTERNATIONAL CONFERENCES OF THE IEEE ENGINEERING IN MEDICINE AND BIOLOGY SOCIETY: ENABLING INNOVATIVE TECHNOLOGIES FOR GLOBAL HEALTHCARE EMBC'20(2020)
摘要
Chest radiographs are primarily employed for the screening of pulmonary and cardio-/thoracic conditions. Being undertaken at primary healthcare centers, they require the presence of an on-premise reporting Radiologist, which is a challenge in low and middle income countries. This has inspired the development of machine learning based automation of the screening process. While recent efforts demonstrate a performance benchmark using an ensemble of deep convolutional neural networks (CNN), our systematic search over multiple standard CNN architectures identified single candidate CNN models whose classification performances were found to be at par with ensembles. Over 63 experiments spanning 400 hours, executed on a 11:3 FP32 TensorTFLOPS compute system, we found the Xception and ResNet-18 architectures to be consistent performers in identifying co-existing disease conditions with an average AUC of 0.87 across nine pathologies. We conclude on the reliability of the models by assessing their saliency maps generated using the randomized input sampling for explanation (RISE) method and qualitatively validating them against manual annotations locally sourced from an experienced Radiologist. We also draw a critical note on the limitations of the publicly available CheXpert dataset primarily on account of disparity in class distribution in training vs. testing sets, and unavailability of sufficient samples for few classes, which hampers quantitative reporting due to sample insufficiency.
更多查看译文
关键词
Chest X-ray, CNN, transfer learning
AI 理解论文
溯源树
样例
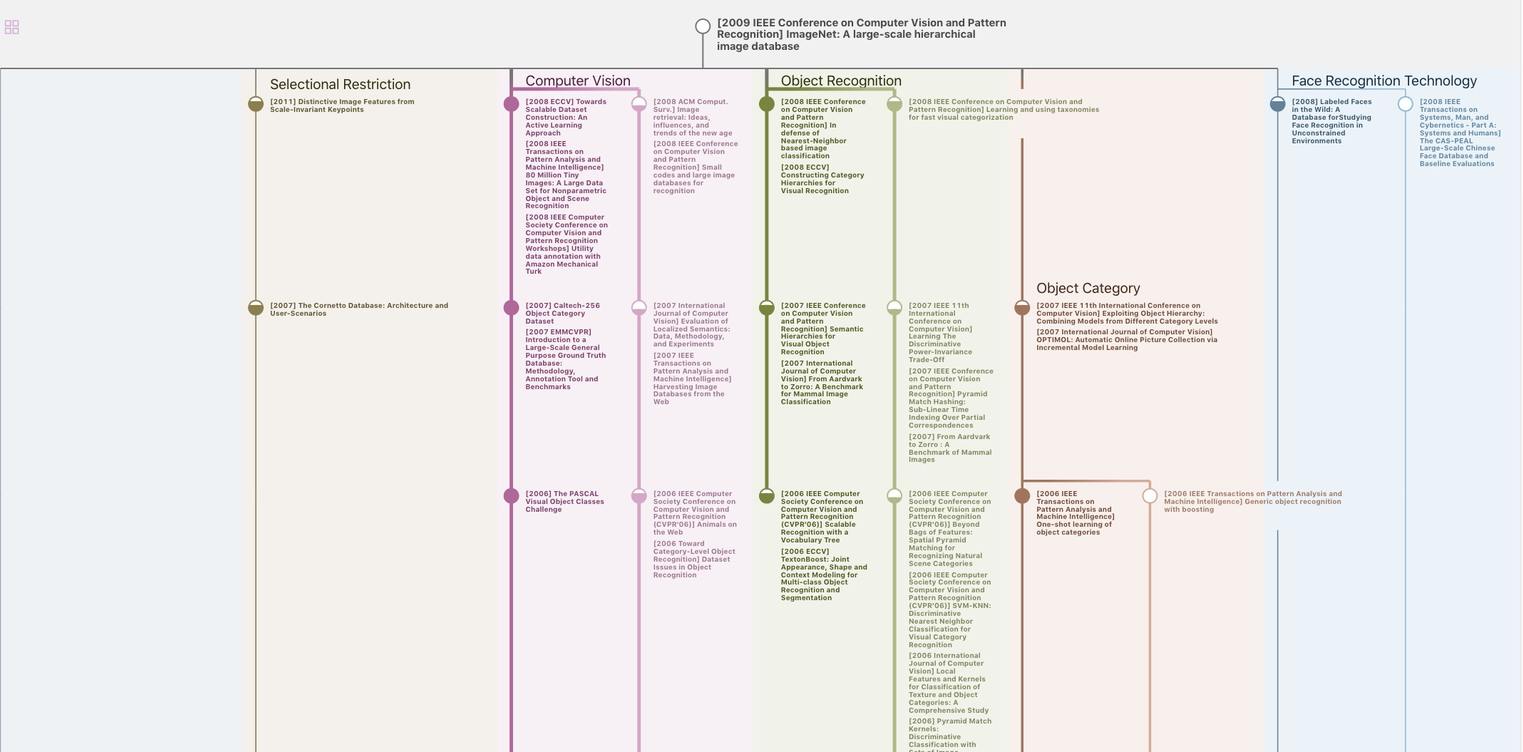
生成溯源树,研究论文发展脉络
Chat Paper
正在生成论文摘要