Recovering Individual-Level Spatial Inference From Aggregated Binary Data
SPATIAL STATISTICS(2021)
摘要
Binary regression models are commonly used in disciplines such as epidemiology and ecology to determine how spatial covariates influence individuals. In many studies, binary data are shared in a spatially aggregated form to protect privacy. For example, rather than reporting the location and result for each individual that was tested for a disease, researchers may report that a disease was detected or not detected within geopolitical units. Often, the spatial aggregation process obscures the values of response variables, spatial covariates, and locations of each individual, which makes recovering individual-level inference difficult. We show that applying a series of transformations, including a change of support, to a bivariate point process model allows researchers to recover individual-level inference for spatial covariates from spatially aggregated binary data. The series of transformations preserves the convenient interpretation of desirable binary regression models that are commonly applied to individual-level data. Using a simulation experiment, we compare the performance of our proposed method under varying types of spatial aggregation against the performance of standard approaches using the original individual-level data. We illustrate our method by modeling individual-level probability of infection using a data set that has been aggregated to protect an at-risk and endangered species of bats. Our simulation experiment and data illustration demonstrate the utility of the proposed method when access to original non-aggregated data is impractical or prohibited. Published by Elsevier B.V.
更多查看译文
关键词
Change of support, Data privacy, Ecological fallacy, Logistic regression, Poisson point process, Probit regression
AI 理解论文
溯源树
样例
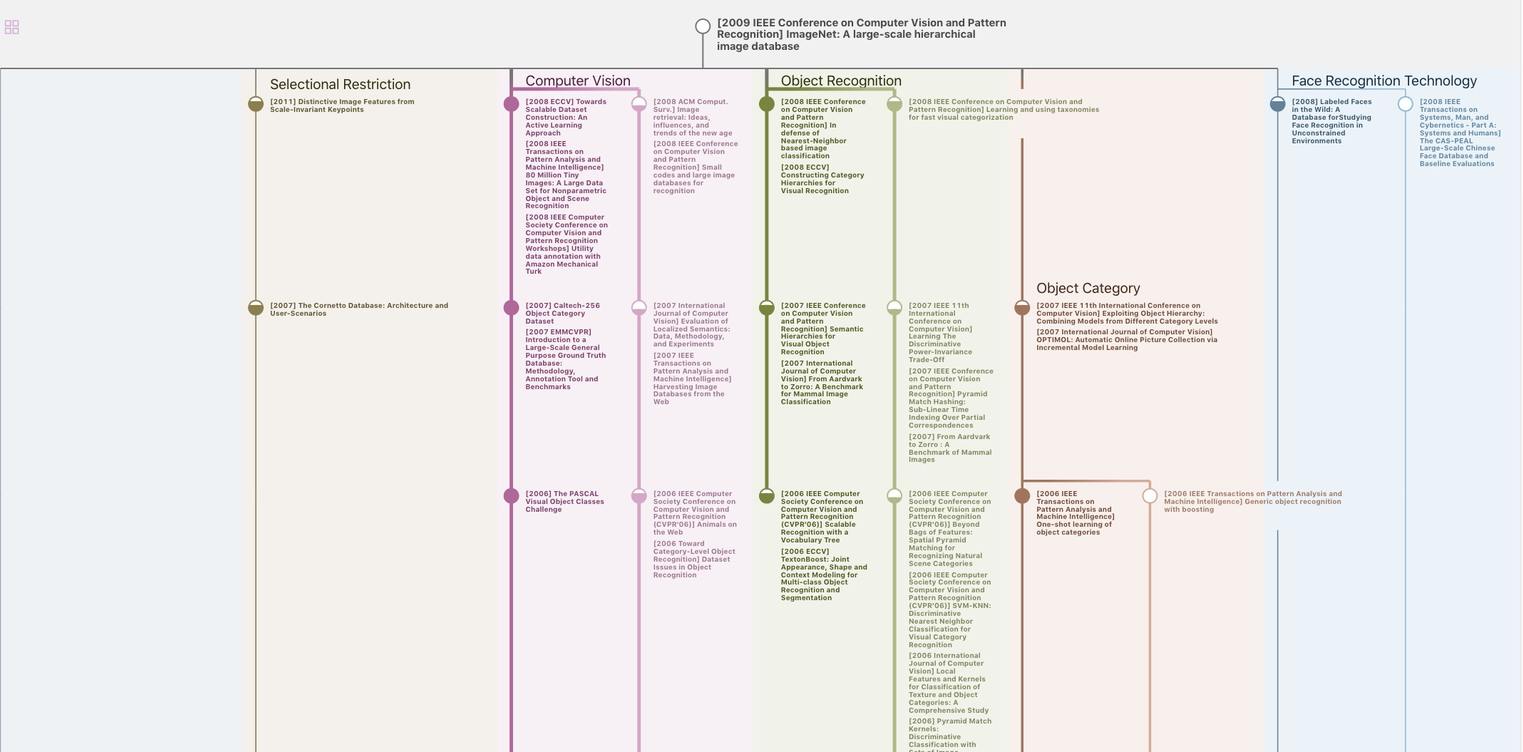
生成溯源树,研究论文发展脉络
Chat Paper
正在生成论文摘要