Joint Inference of States, Robot Knowledge, and Human (False-)Beliefs
ICRA(2020)
摘要
Aiming to understand how human (false-)belief--a core socio-cognitive ability--would affect human interactions with robots, this paper proposes to adopt a graphical model to unify the representation of object states, robot knowledge, and human (false-)beliefs. Specifically, a parse graph (pg) is learned from a single-view spatiotemporal parsing by aggregating various object states along the time; such a learned representation is accumulated as the robot's knowledge. An inference algorithm is derived to fuse individual pg from all robots across multi-views into a joint pg, which affords more effective reasoning and inference capability to overcome the errors originated from a single view. In the experiments, through the joint inference over pg-s, the system correctly recognizes human (false-)belief in various settings and achieves better cross-view accuracy on a challenging small object tracking dataset.
更多查看译文
关键词
robot knowledge,human interactions,graphical model,object states,parse graph,single-view spatiotemporal parsing,learned representation,inference algorithm,joint pg,effective reasoning,inference capability,states joint inference,human beliefs,socio-cognitive ability,false-beliefs,individual parse graph,small object tracking dataset
AI 理解论文
溯源树
样例
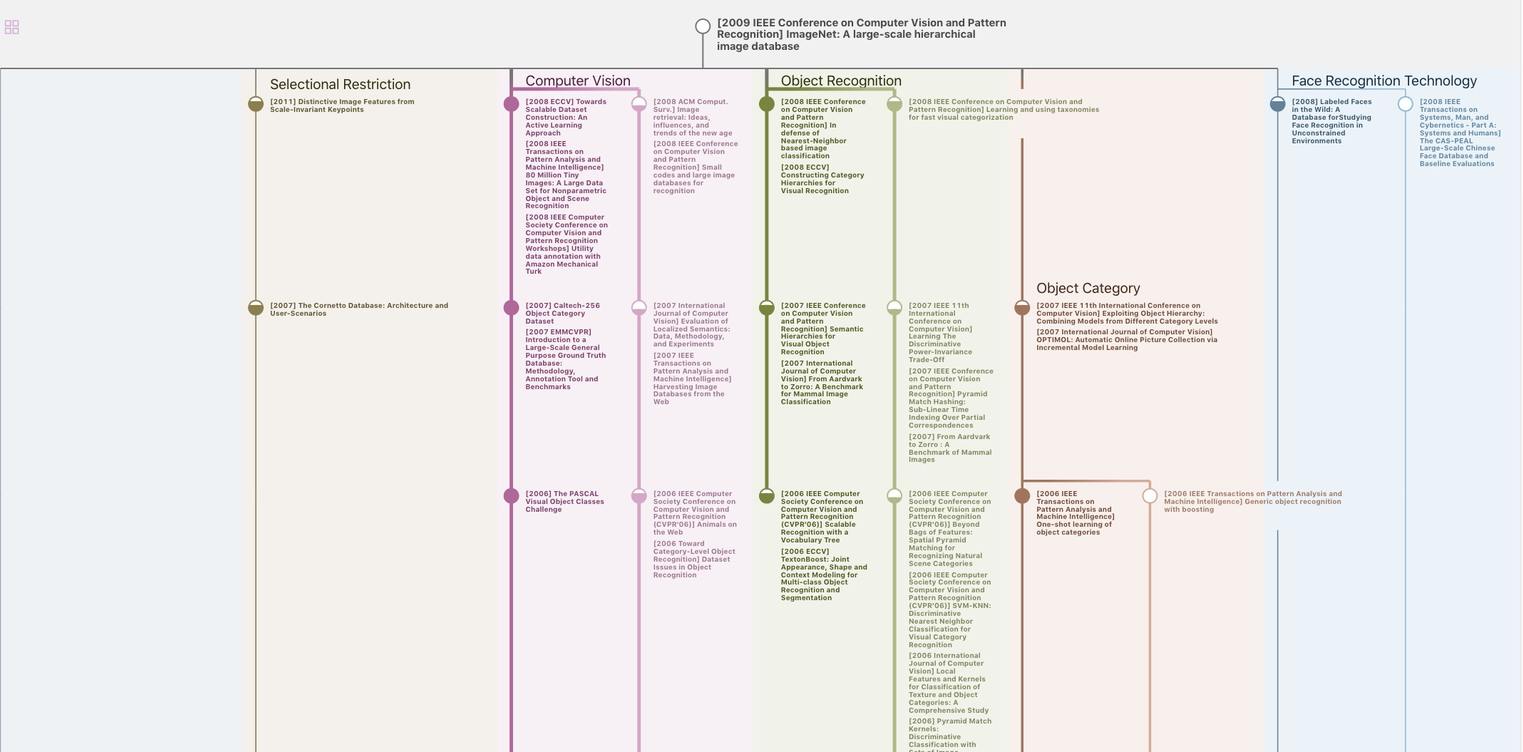
生成溯源树,研究论文发展脉络
Chat Paper
正在生成论文摘要