Gluecos : An Evaluation Benchmark For Code-Switched Nlp
58TH ANNUAL MEETING OF THE ASSOCIATION FOR COMPUTATIONAL LINGUISTICS (ACL 2020)(2020)
摘要
Code-switching is the use of more than one language in the same conversation or utterance. Recently, multilingual contextual embedding models, trained on multiple monolingual corpora, have shown promising results on cross-lingual and multilingual tasks. We present an evaluation benchmark, GLUECoS, for code-switched languages, that spans several NLP tasks in English-Hindi and English Spanish. Specifically, our evaluation benchmark includes Language Identification from text, POS tagging, Named Entity Recognition, Sentiment Analysis, Question Answering and a new task for code-switching, Natural Language Inference. We present results on all these tasks using cross-lingual word embedding models and multilingual models. In addition, we fine-tune multilingual models on artificially generated code-switched data. Although multilingual models perform significantly better than cross-lingual models, our results show that in most tasks, across both language pairs, multilingual models fine-tuned on code-switched data perform best, showing that multilingual models can be further optimized for code-switching tasks.
更多查看译文
关键词
evaluation benchmark,code-switched
AI 理解论文
溯源树
样例
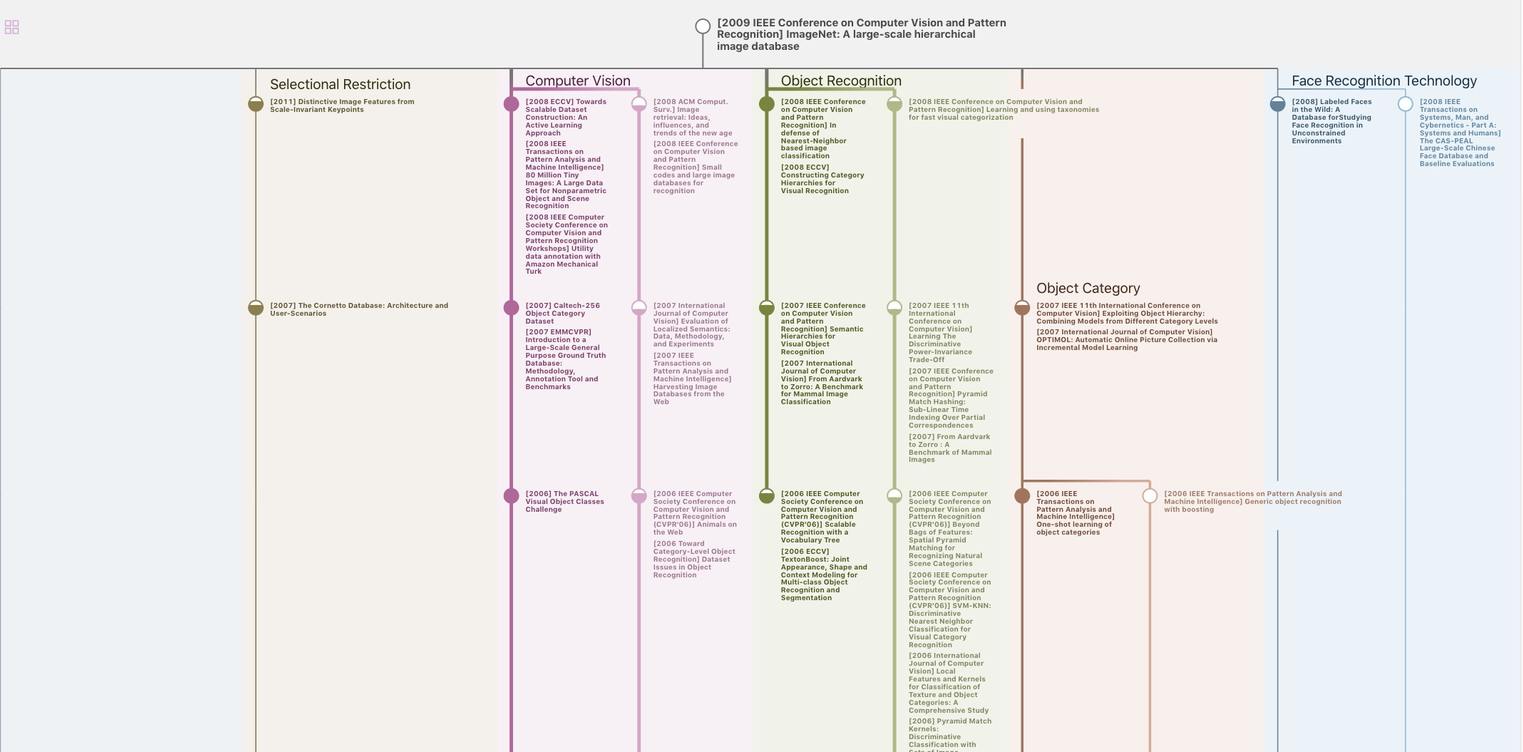
生成溯源树,研究论文发展脉络
Chat Paper
正在生成论文摘要