Hamiltonian Monte Carlo using an embedded Laplace approximation
arxiv(2020)
摘要
Latent Gaussian models are a popular class of hierarchical models with applications in many fields. Performing Bayesian inference on such models can be challenging. Markov chain Monte Carlo algorithms struggle with the geometry of the resulting posterior distribution and can be prohibitively slow. An alternative is to use an integrated nested Laplace approximation, whereby we marginalize out the latent Gaussian variables and estimate the hyperparameters with a deterministic scheme. This type of inference typically only works for low dimensional and unimodal hyperparameters. We bypass these limitations by coupling dynamic Hamiltonian Monte Carlo with an embedded Laplace approximation. Our implementation features a novel adjoint method to differentiate the marginal likelihood, which scales with high dimensional hyperparameters. We prototype the method in the probabilistic programming framework Stan and test the utility of the embedded Laplace approximation on several models: a classic Gaussian process, a general linear regression model with a sparsity inducing horseshoe prior, and a sparse kernel interaction model. The last two models are characterized by a high dimensional and a multimodal posterior distribution of the hyperparameters, and as such present novel applications of the embedded Laplace approximation. Depending on the cases, the benefits are either a dramatic speed-up, or an alleviation of the geometric pathologies that frustrate Hamiltonian Monte Carlo.
更多查看译文
AI 理解论文
溯源树
样例
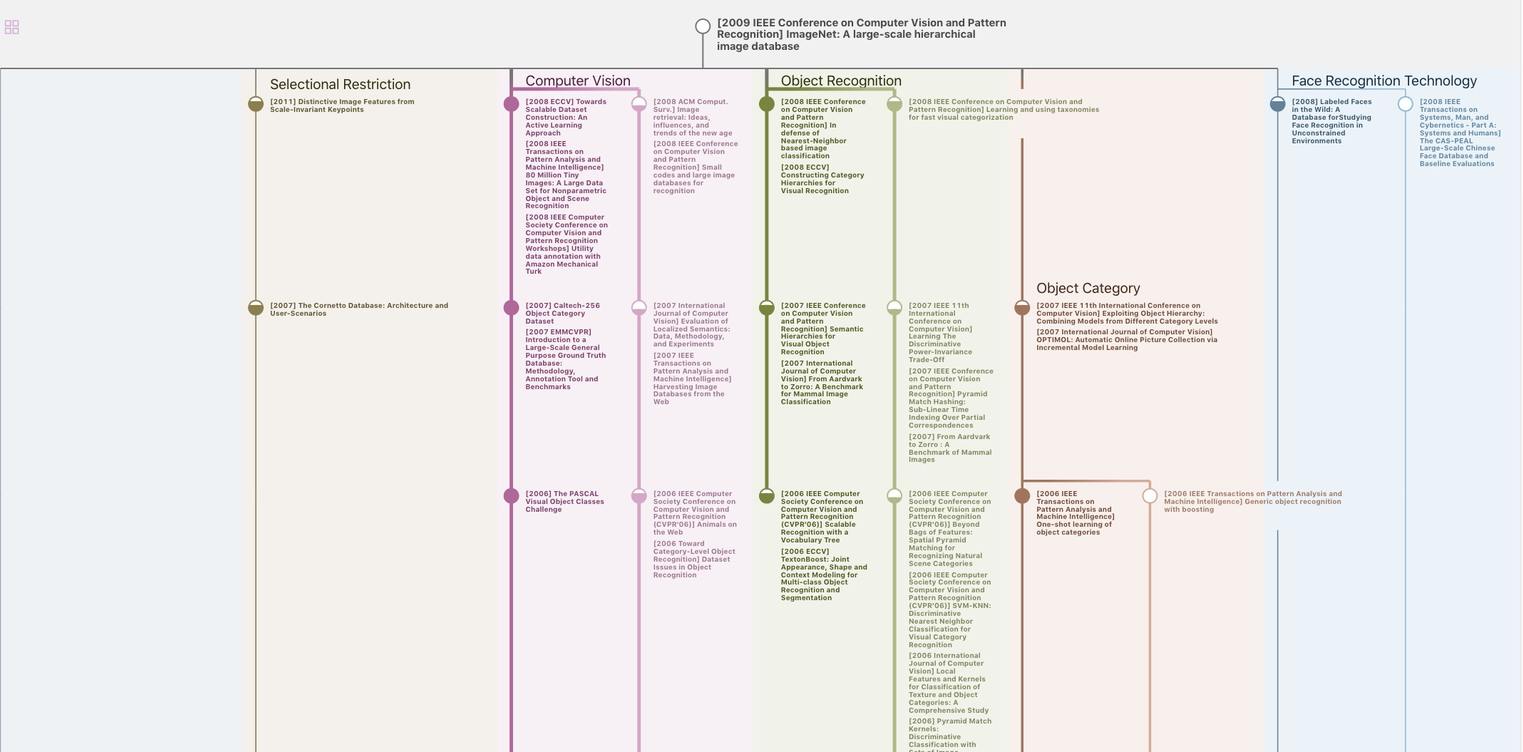
生成溯源树,研究论文发展脉络
Chat Paper
正在生成论文摘要