Evolutionary Stochastic Policy Distillation
arxiv(2020)
摘要
Solving the Goal-Conditioned Reward Sparse (GCRS) task is a challenging reinforcement learning problem due to the sparsity of reward signals. In this work, we propose a new formulation of GCRS tasks from the perspective of the drifted random walk on the state space, and design a novel method called Evolutionary Stochastic Policy Distillation (ESPD) to solve them based on the insight of reducing the First Hitting Time of the stochastic process. As a self-imitate approach, ESPD enables a target policy to learn from a series of its stochastic variants through the technique of policy distillation (PD). The learning mechanism of ESPD can be considered as an Evolution Strategy (ES) that applies perturbations upon policy directly on the action space, with a SELECT function to check the superiority of stochastic variants and then use PD to update the policy. The experiments based on the MuJoCo robotics control suite show the high learning efficiency of the proposed method.
更多查看译文
关键词
distillation,policy
AI 理解论文
溯源树
样例
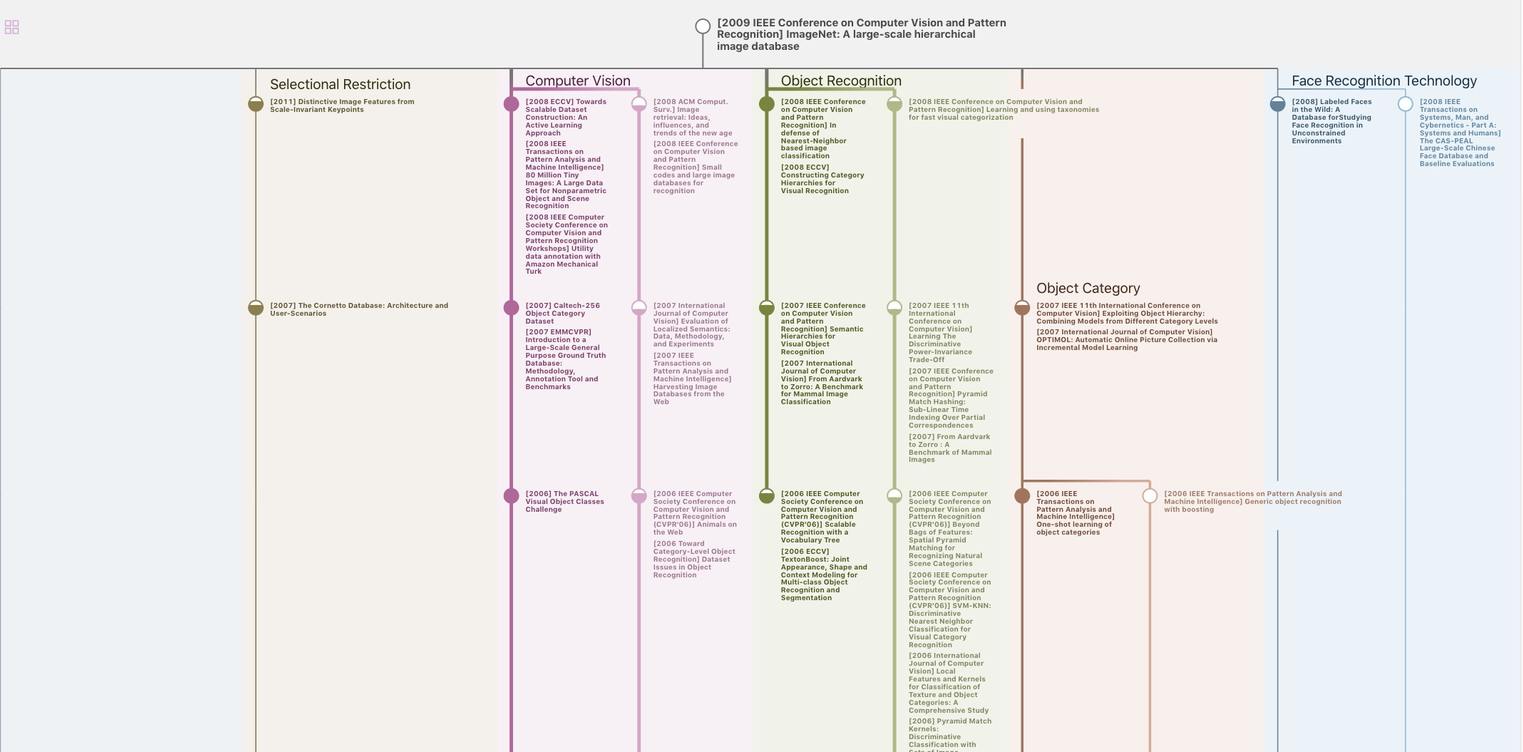
生成溯源树,研究论文发展脉络
Chat Paper
正在生成论文摘要