GIS-based spatial modeling of COVID-19 incidence rate in the continental United States.
Science of The Total Environment(2020)
摘要
During the first 90 days of the COVID-19 outbreak in the United States, over 675,000 confirmed cases of the disease have been reported, posing unprecedented socioeconomic burden to the country. Due to inadequate research on geographic modeling of COVID-19, we investigated county-level variations of disease incidence across the continental United States. We compiled a geodatabase of 35 environmental, socioeconomic, topographic, and demographic variables that could explain the spatial variability of disease incidence. Further, we employed spatial lag and spatial error models to investigate spatial dependence and geographically weighted regression (GWR) and multiscale GWR (MGWR) models to locally examine spatial non-stationarity. The results suggested that even though incorporating spatial autocorrelation could significantly improve the performance of the global ordinary least square model, these models still represent a significantly poor performance compared to the local models. Moreover, MGWR could explain the highest variations (adj. R2: 68.1%) with the lowest AICc compared to the others. Mapping the effects of significant explanatory variables (i.e., income inequality, median household income, the proportion of black females, and the proportion of nurse practitioners) on spatial variability of COVID-19 incidence rates using MGWR could provide useful insights to policymakers for targeted interventions.
更多查看译文
关键词
COVID-19,GIS,Multiscale GWR,Spatial non-stationarity
AI 理解论文
溯源树
样例
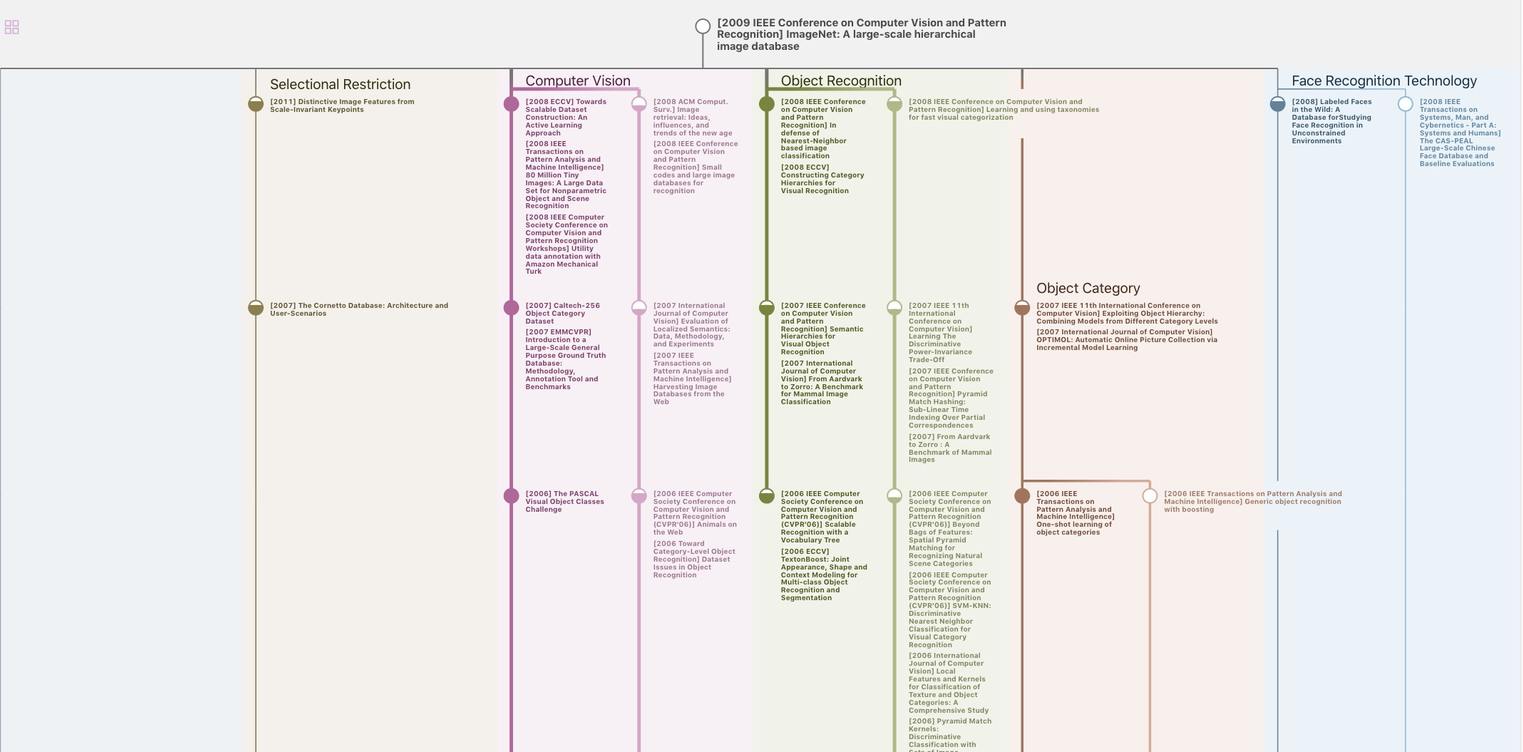
生成溯源树,研究论文发展脉络
Chat Paper
正在生成论文摘要