Assessing the Bilingual Knowledge Learned by Neural Machine Translation Models
arxiv(2020)
摘要
Machine translation (MT) systems translate text between different languages by automatically learning in-depth knowledge of bilingual lexicons, grammar and semantics from the training examples. Although neural machine translation (NMT) has led the field of MT, we have a poor understanding on how and why it works. In this paper, we bridge the gap by assessing the bilingual knowledge learned by NMT models with phrase table -- an interpretable table of bilingual lexicons. We extract the phrase table from the training examples that an NMT model correctly predicts. Extensive experiments on widely-used datasets show that the phrase table is reasonable and consistent against language pairs and random seeds. Equipped with the interpretable phrase table, we find that NMT models learn patterns from simple to complex and distill essential bilingual knowledge from the training examples. We also revisit some advances that potentially affect the learning of bilingual knowledge (e.g., back-translation), and report some interesting findings. We believe this work opens a new angle to interpret NMT with statistic models, and provides empirical supports for recent advances in improving NMT models.
更多查看译文
关键词
bilingual knowledge,neural machine translation models
AI 理解论文
溯源树
样例
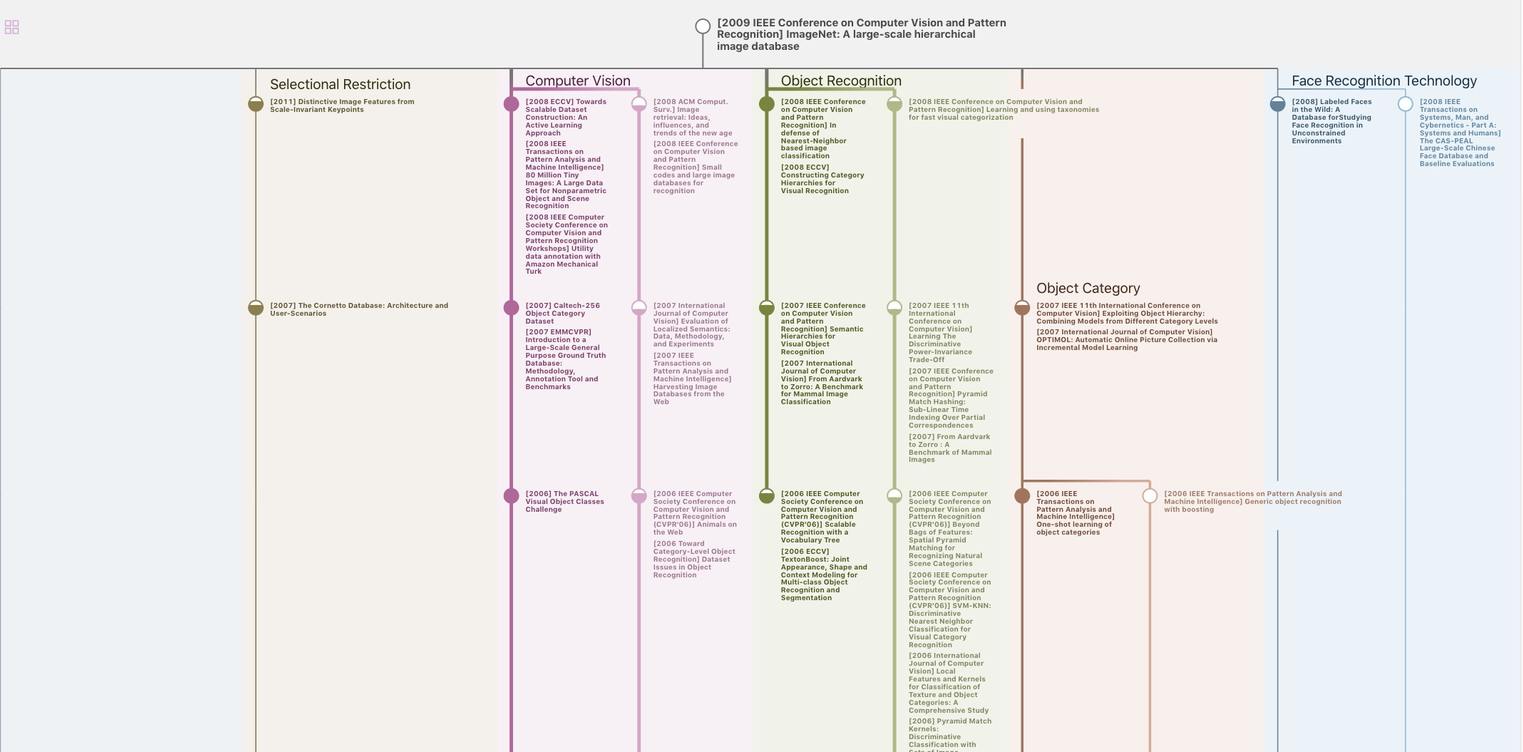
生成溯源树,研究论文发展脉络
Chat Paper
正在生成论文摘要