Towards Efficient and Accurate Spectroscopic Simulations in Extended Systems with Symmetry-Preserving Neural Network Models for Tensorial Properties
arxiv(2020)
摘要
Machine learning (ML) methods have revolutionized the high-dimensional representations for scalar molecular properties such as potential energy. However, there have been scarce ML models targeting tensorial properties, which suffer from intrinsic covariant transformation with regard to rotation. Here, we generalize an atomistic neural network (NN) model to learn both tensorial response and transition properties, in which atomic position vectors are multiplied with scalar NN outputs or their derivatives to preserve the rotationally covariant symmetry. This strategy keeps these geometric descriptors symmetry invariant so that the resulting tensorial NN models are as efficient as their scalar counterparts. We validate the performance and universality of this approach by learning response properties of water oligomers and liquid water. More impressively, by learning transition dipole moments of a model structural unit of proteins, the ab initio based ultraviolet spectra of real proteins are faithfully reproduced with ~1000000 times speedup. This concept can be readily adapted to any atomistic NN framework to predict tensorial properties, promising efficient and accurate spectroscopic simulations for biomolecules and materials.
更多查看译文
AI 理解论文
溯源树
样例
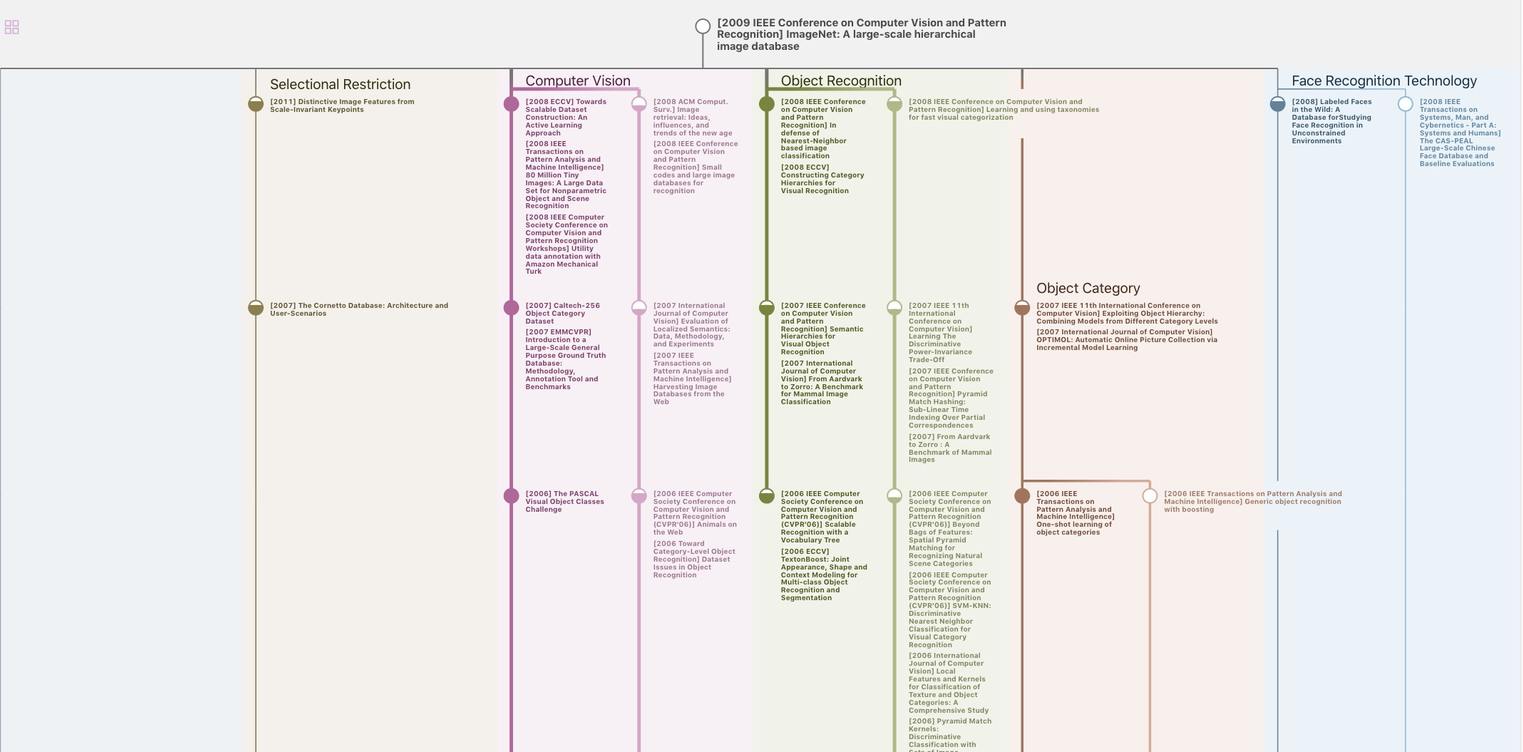
生成溯源树,研究论文发展脉络
Chat Paper
正在生成论文摘要