Impact of Gaussian uncertainty assumptions on probabilistic optimization in particle therapy.
PHYSICS IN MEDICINE AND BIOLOGY(2020)
摘要
Range and setup uncertainties in charged particle therapy may induce a discrepancy between the planned and the delivered dose. Countermeasures based on probabilistic (stochastic) optimization usually assume a Gaussian probability density to model the underlying range and setup error. While this standard assumption is generally taken for granted, this study explicitly investigates the dosimetric consequences if the actual range and setup errors obey a different probability density function (PDF) over the course of treatment to the one used during the probabilistic treatment plan optimization. Discrete random sampling was performed for conventionally and probabilistically optimized proton and carbon ion treatment plans utilizing various PDFs that modeled the setup and range error. This method allowed us to assess the treatment plan robustness against different PDFs of conventional and probabilistic plans, which both explicitly assume Gaussian uncertainties. The induced uncertainty in dose was quantified by estimating the expectation value and standard deviation of the RBE-weighted dose for each PDF on the basis of 2500-5000 random dose samples. Probabilistic dose metrics and standard deviation volume histograms were computed to quantify treatment plan robustness of both optimization approaches. It was shown that the classical planning target volume margin extension concept did not compensate the influence of range and setup errors and consequently resulted in a non-negligible average standard deviation in dose of 7.3% throughout the clinical target volume (CTV). In contrast, probabilistic optimization on normally distributed errors yielded treatment plans that not only entailed a lower standard deviation against normally distributed errors accounted for during optimization, but also lower standard deviations for other symmetric PDFs. It was shown that the impact of an incorrect probability distribution assumption is of lower importance after probabilistic optimization as the average uncertainty in the CTV drops to 3.9%. Probabilistic optimization is an effective tool to create robust particle treatment plans. Normally distributed range and setup error assumptions for probabilistic optimization are a reasonable first approximation and yield treatment plans that are also robust against other PDFs.
更多查看译文
关键词
intensity-modulated proton therapy,range uncertainties,probability distributions,robust optimization,uncertainty quantification,probabilistic optimization
AI 理解论文
溯源树
样例
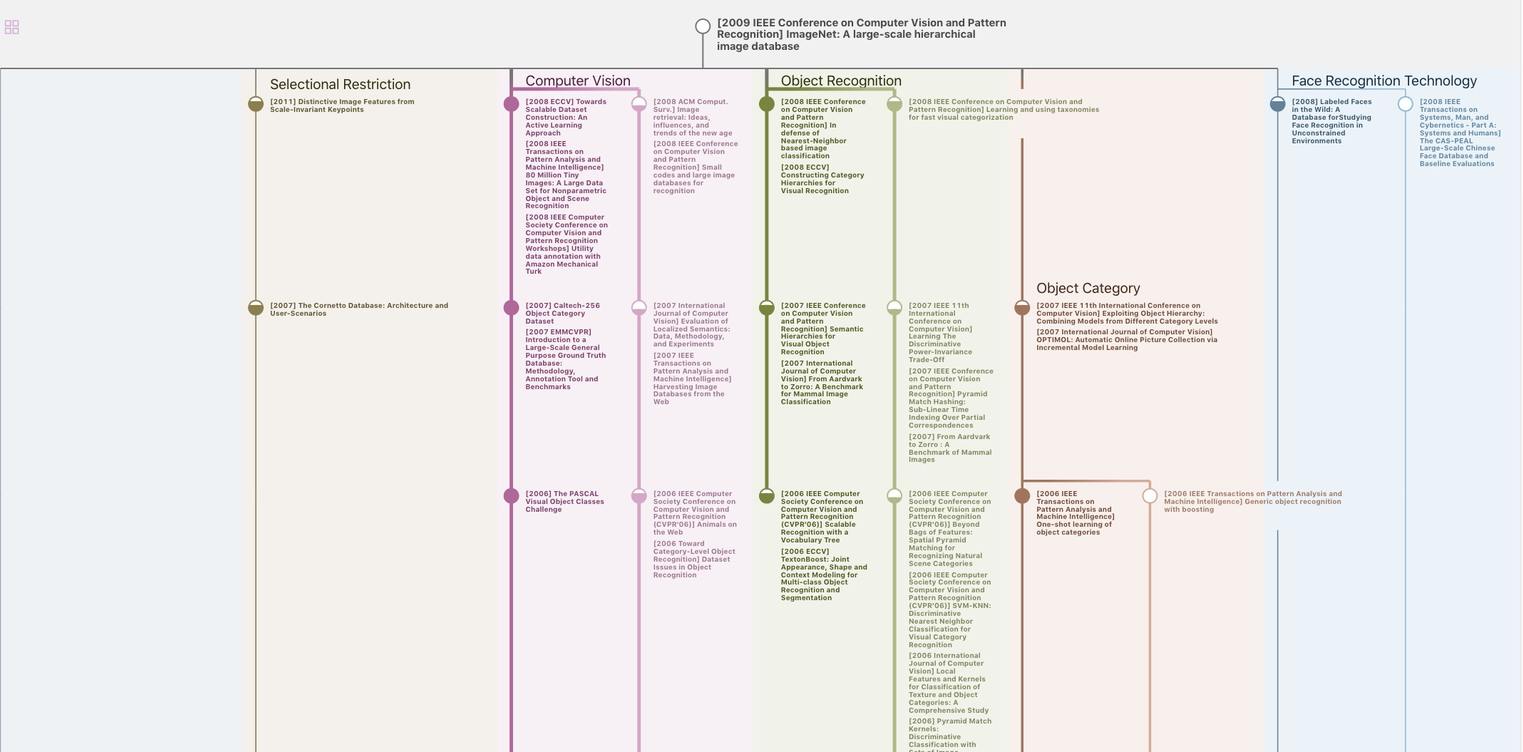
生成溯源树,研究论文发展脉络
Chat Paper
正在生成论文摘要