Textgail: Generative Adversarial Imitation Learning For Text Generation
THIRTY-FIFTH AAAI CONFERENCE ON ARTIFICIAL INTELLIGENCE, THIRTY-THIRD CONFERENCE ON INNOVATIVE APPLICATIONS OF ARTIFICIAL INTELLIGENCE AND THE ELEVENTH SYMPOSIUM ON EDUCATIONAL ADVANCES IN ARTIFICIAL INTELLIGENCE(2021)
摘要
Generative Adversarial Networks (GANs) for text generation have recently received many criticisms, as they perform worse than their MLE counterparts (Caccia et al. 2020; Tevet et al. 2019; Semeniuta, Severyn, and Gelly 2018). We suspect previous text GANs' inferior performance is due to the lack of a reliable guiding signal in their discriminators. To address this problem, we propose a generative adversarial imitation learning framework for text generation that uses large pre-trained language models to provide more reliable reward guidance. As previous text GANs suffer from high variance of gradients, we apply contrastive discriminator, and proximal policy optimization (PPO) to stabilize and improve text generation performance. For evaluation, we conduct experiments on a diverse set of unconditional and conditional text generation tasks. Experimental results show that TextGAIL achieves better performance in terms of both quality and diversity than the MLE baseline. We also validate our intuition that TextGAIL's discriminator demonstrates the capability of providing reasonable rewards with an additional task.
更多查看译文
关键词
generative adversarial imitation learning,generation,textgail
AI 理解论文
溯源树
样例
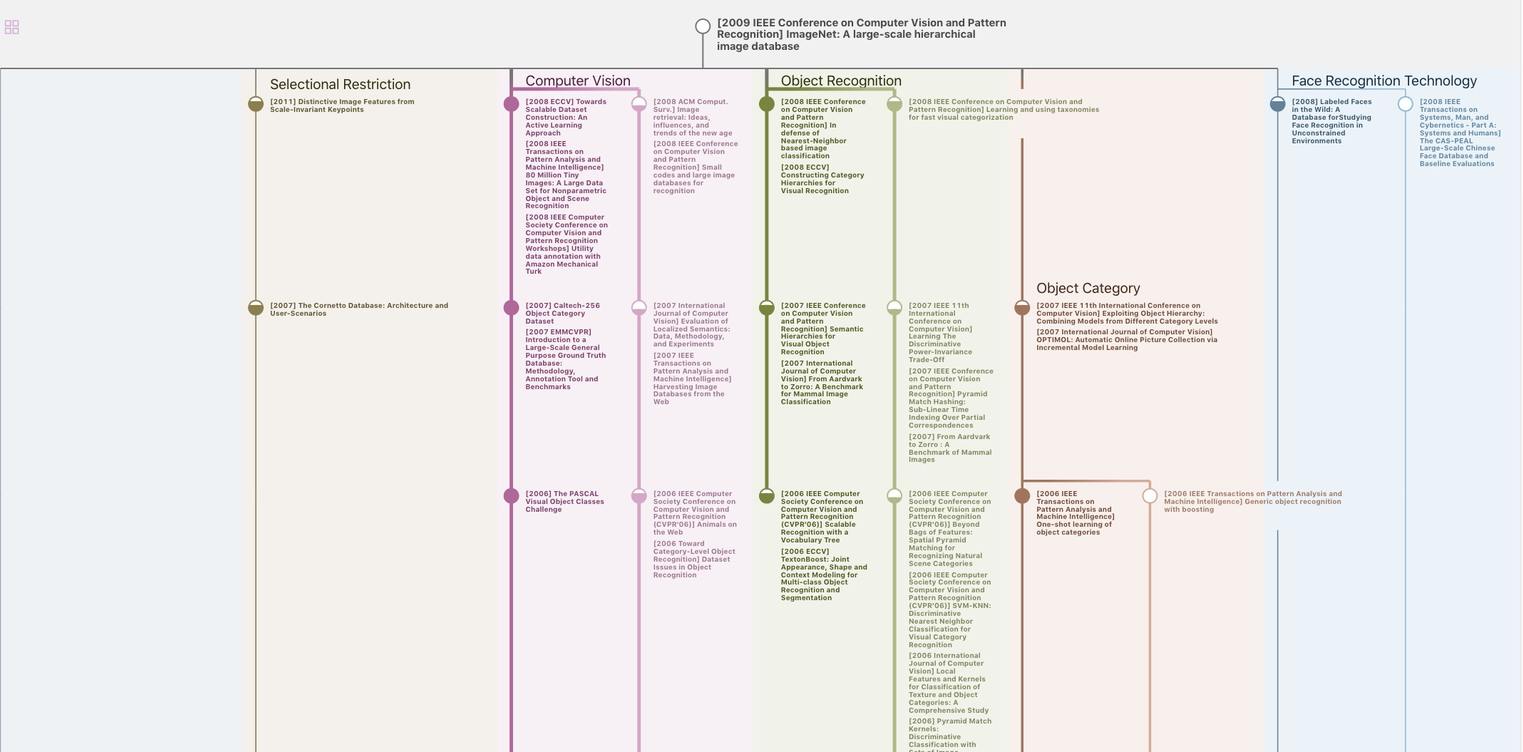
生成溯源树,研究论文发展脉络
Chat Paper
正在生成论文摘要